Towards physician's experience: Development of machine learning model for the diagnosis of autism spectrum disorders based on complex T-spherical fuzzy-weighted zero-inconsistency method
COMPUTATIONAL INTELLIGENCE(2023)
摘要
Autism spectrum disorders (ASD) are a diverse group of conditions characterized by difficulty with social interaction and communication. ASD is expected to be a high-risk disease. Recent studies have focused on the diagnosis based on sociodemographic and family characteristics factors. The development of a diagnosis model, which is primarily based on machine learning methods, has been carried out to alleviate the detection of autism. However, they neglected the importance of ASD features in a training dataset, especially because some features have different levels of contributions to the processing data and possess more relevancies to the classification information than others. Such limitations use preprocessing techniques for the construction of the machine learning model, but the role of the physician's experience towards feature contributions remains limited. However, for certain autism datasets, the relevancies of sociodemographic and family characteristic feature concerning the given class labels should be considered. Accordingly, this study developed a new machine learning model for the diagnosis of ASD based on multi-criteria decision-making (MCDM). By using three methodology phases, the model combines two representative theories, namely, MCDM and machine learning. The identification phase for imbalance ASD dataset and application of pre-possessing stages by imputing missing values, feature selection of sociodemographic and family characteristics, and data imbalanced approach resulted in balanced ASD dataset, including 107,573 cases. The development phase for the new model was achieved by the proposed complex T-spherical fuzzy-weighted zero-inconsistency (CT-SFWZIC) method. CT-SFWZIC was developed based on a new fuzzy set (i.e., complex T-spherical fuzzy) for weighting affected features, and then applied for training and testing the machine learning model considering various complex T-spherical fuzzy membership functions (i.e., T = 1, 2, 3, 5, 7, and 10). The results obtained from a 10-fold cross-validation test for all T values by using nine machine learning classifiers were measured under seven evaluation metrics, namely AUC, accuracy, F1, precision, recall, training time (s), and test time (s). Performance evaluation results reveal that AdaBoost can be used to boost the ASD diagnosis as the best machine learning algorithm for all T values based on all metrics to improve the diagnosis based on physician's assessment. Under the most extreme evaluation metric, which is accuracy, the results of the AdaBoost classifiers for T = 1, 2, 3, 5, 7, 10 have obtained 0.99948, 0.99934, 0.99930, 0.99939, 0.99910, and 0.99930, respectively.
更多查看译文
关键词
autism spectrum disorders,detection and diagnosis,machine learning,MCDM,prediction
AI 理解论文
溯源树
样例
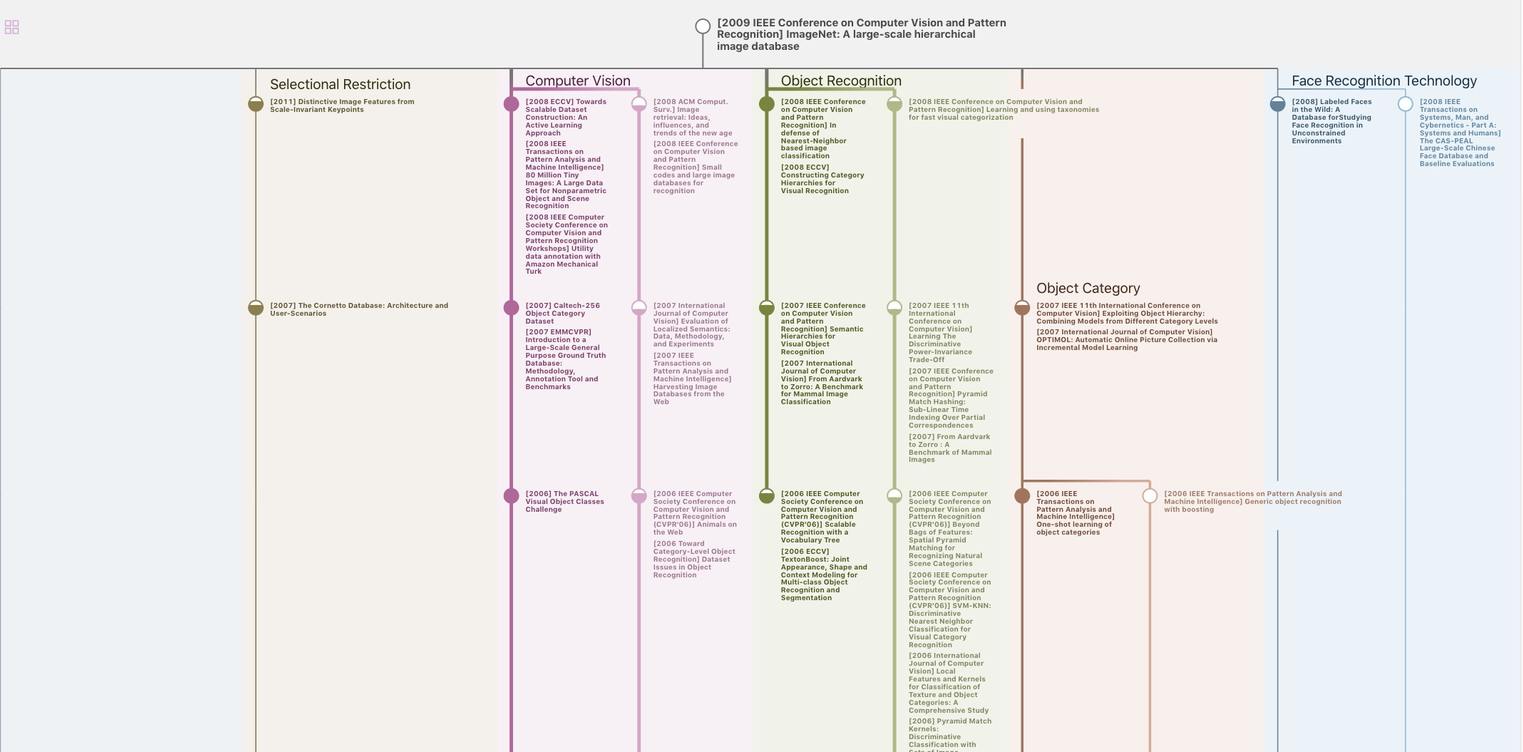
生成溯源树,研究论文发展脉络
Chat Paper
正在生成论文摘要