DRJOA: intelligent resource management optimization through deep reinforcement learning approach in edge computing
CLUSTER COMPUTING-THE JOURNAL OF NETWORKS SOFTWARE TOOLS AND APPLICATIONS(2022)
摘要
Mobile edge computing (MEC) can enhance the computation capabilities of smart mobile devices for computation-intensive mobile applications via supporting computation offloading efficiently. However, the limitation of wireless resources and computational resources of edge servers often becomes the bottlenecks to realizing the developments of MEC. In order to address the computation offloading problem in the time-varying wireless networks, the offloading decisions and the allocation of radio and computation resources need to be jointly managed. Traditional optimization methods are challenging to deal with the combinatorial optimization problem in complex real-time dynamic network environments. Therefore, we propose a deep reinforcement learning (DRL)-based optimization approach, named DRJOA, which jointly optimizes offloading decisions, computation, and wireless resources allocation. The optimization algorithm based on DRL has the advantages of fast solving speed and strong generalization ability, which makes it possible to solve combinatorial optimization problems online. Simulation results show that our proposed DRJOA in this study dramatically outperforms the benchmark methods for offloading decisions and system utility.
更多查看译文
关键词
Computation offloading,Deep reinforcement learning,Mobile edge computing,Resource allocation.
AI 理解论文
溯源树
样例
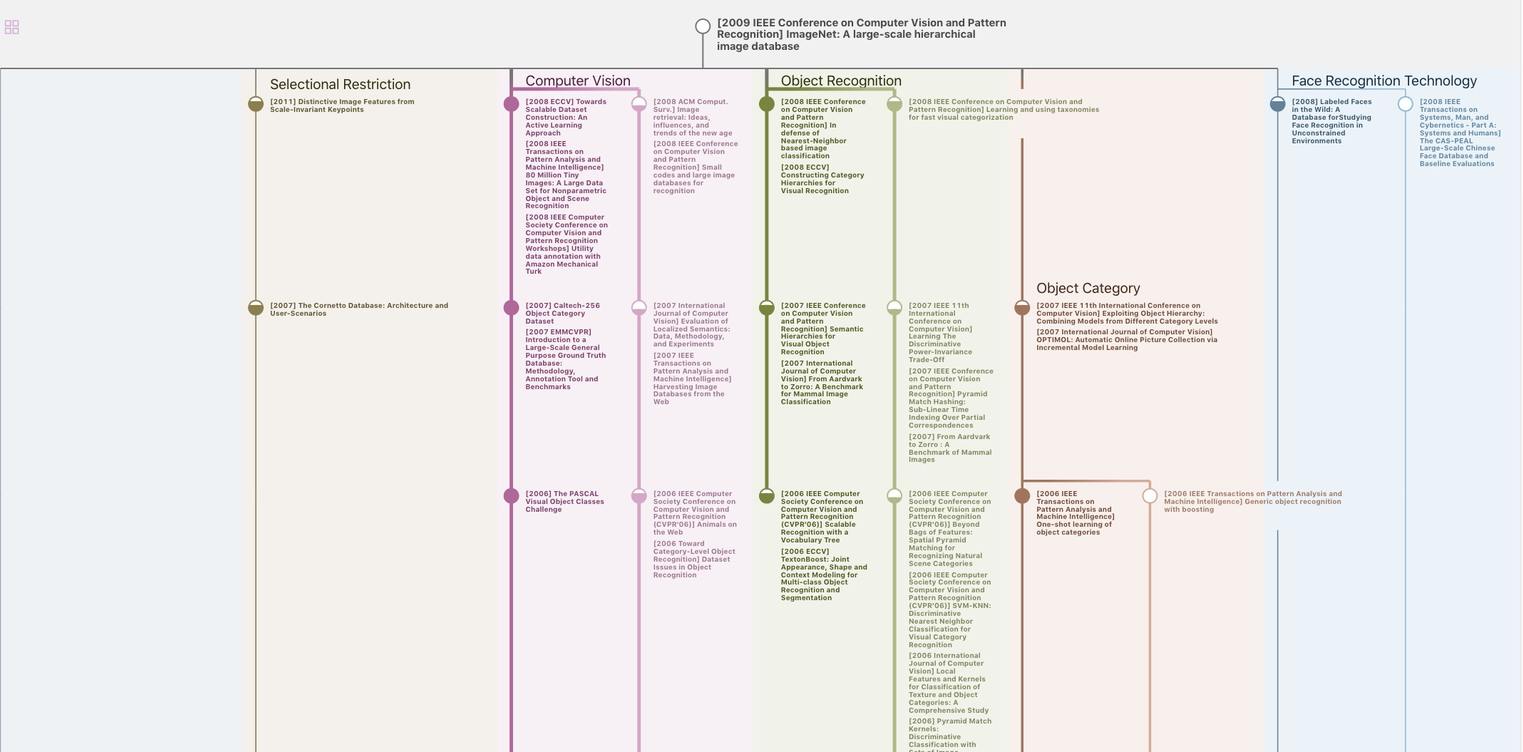
生成溯源树,研究论文发展脉络
Chat Paper
正在生成论文摘要