Fault Diagnosis of Complex Industrial Systems Based on Multi-Granularity Dictionary Learning and Its Application
IEEE TRANSACTIONS ON AUTOMATION SCIENCE AND ENGINEERING(2024)
摘要
Nowadays, the intelligent fault diagnosis problem of modern industrial systems has received increasing attention. However, with the increasing scale of industrial systems, the same category of faulty data often contains multiple working conditions, which leads to the multi-granularity of faulty data and the increasing complexity of data distribution. The possible existence of fine-granularity inter-class similarities in multi-granularity data can interfere with coarse-granularity recognition tasks, which brings challenges to data-driven fault diagnosis tasks. To solve the above issue, a fault diagnosis method based on multi-granularity dictionary learning is proposed in this paper. Specifically, the proposed method integrates prior knowledge into the dictionary learning framework by introducing the concept of "centroid atom ", which embeds the multi-granularity structure in the dictionary and improves the discrimination of dictionary atoms. In order to validate the proposed approach, experiments are conducted on a numerical simulation case and a zinc smelting roasting process. Compared with some state-of-the-art methods, the proposed approach performs better in the classification accuracy which shows that it can make full use of the knowledge provided by the multi-granularity structure while it is difficult to be modeled by the other methods. Therefore, it can be applied to fault diagnosis tasks more precisely and efficiently. Note to Practitioners-Motivated by the fact that industrial faults often occur in different modes, and different faults may occur in the same mode, the fault data often has multi-granularity characteristics, this paper proposes a multi-granularity dictionary learning method for complex industrial fault diagnosis. The proposed method can learn the multi-granularity structure in the data, and the obtained model contains both coarse-grained and fine-grained information, which effectively improves the fault diagnosis accuracy. Intensive experimental results show that the proposed method performs better than some state-of-the-art methods, it can make full use of the knowledge provided by the multi-granularity structure, while it is difficult to be modeled by others. Above all, it is verified as suitable for fault diagnosis of real industrial systems.
更多查看译文
关键词
Fault diagnosis,dictionary learning,multi-granularity structure
AI 理解论文
溯源树
样例
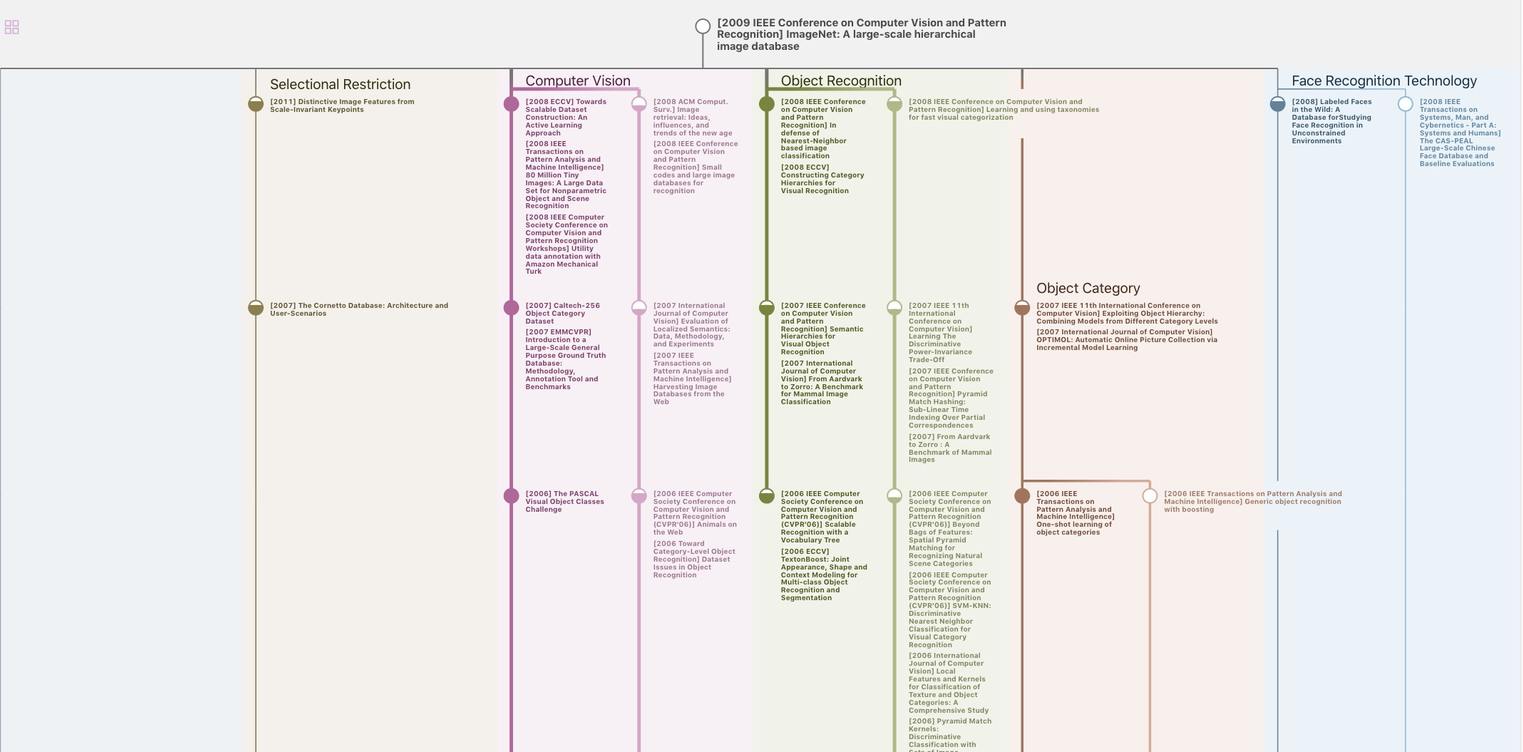
生成溯源树,研究论文发展脉络
Chat Paper
正在生成论文摘要