Learning fusion feature representation for garbage image classification model in human-robot interaction
Infrared Physics & Technology(2023)
摘要
Garbage image classification often suffers from three aspect challenges: complex image background, same-shape category, and low-quality image. The existing machine vision methods have excellent learning capabilities. However, they require powerful computational resources. In this work, an efficient garbage image classification network (GScbamKL-Net) is proposed in this work to address the problems mentioned. The proposed network is designed from the following three aspects. First, the new network unit with group convolution and channel shuffle is designed. This unit can significantly reduce the number of parameters of the model and achieve good performance. Second, the CBAM attention mechanism, which can extract key features by weighting the output features in space and channel, is added to the network unit. Furthermore, the LeakyReLu function is introduced as the activation function model. A label smoothing function is constructed as the loss function. It can mitigate the errors and effects of sample imbalance and obtain a good nonlinear transformation effect. The normal garbage images and garbage images using infrared imaging technology were tested respectively. Experimental results show that the proposed GScbam-Net has excellent classification performance while maintaining its lightweight.
更多查看译文
关键词
Infrared imaging,Image classification,Group convolution,Channel shuffle,CBAM,Label smoothing
AI 理解论文
溯源树
样例
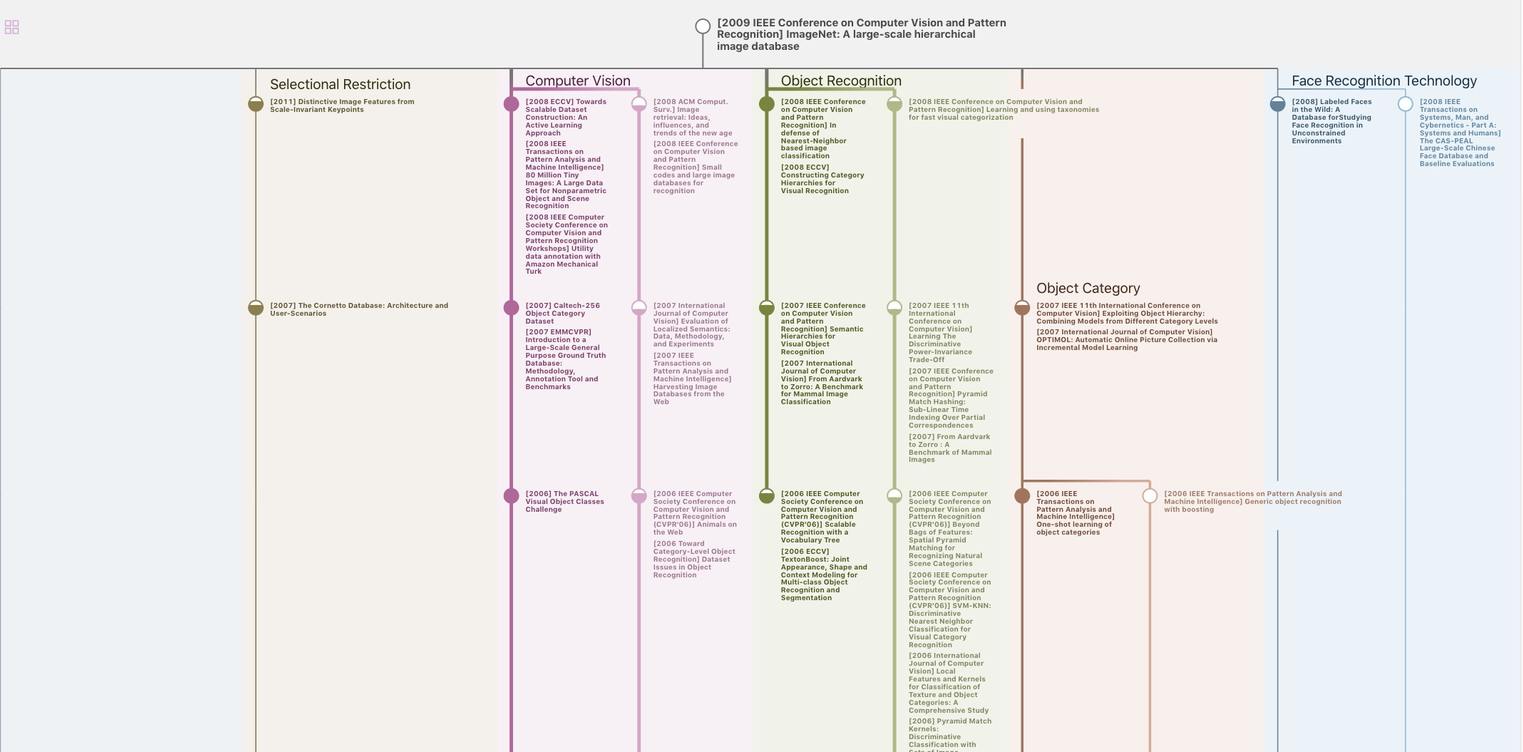
生成溯源树,研究论文发展脉络
Chat Paper
正在生成论文摘要