Machine-learning-based monitoring and optimization of processing parameters in 3D printing
INTERNATIONAL JOURNAL OF COMPUTER INTEGRATED MANUFACTURING(2022)
Abstract
Additive manufacturing (AM), commonly known as 3D printing, is a rapidly growing technology. Guaranteeing the quality and mechanical strength of printed parts is an active research area. Most of the existing methods adopt open-loop-like Machine Learning (ML) algorithms that can be used only for predicting properties of printed parts without any quality assuring mechanism. Some closed-loop approaches, on the other hand, consider a single adjustable processing parameter to monitor the properties of a printed part. This work proposes both open-loop and closed-loop ML models and integrates them to monitor the effects of processing parameters on the quality of printed parts. By using experimental 3D printing data, an open-loop classification model formulates the relationship between processing parameters and printed part properties. Then, a closed-loop control algorithm that combines open-loop ML models and a fuzzy inference system is constructed to generate optimized processing parameters for better printed part properties. The proposed system realizes the application of a closed-loop control system to AM.
MoreTranslated text
Key words
Additive manufacturing, closed-loop, 3D printing, digital manufacturing, machine learning, processing parameters
AI Read Science
Must-Reading Tree
Example
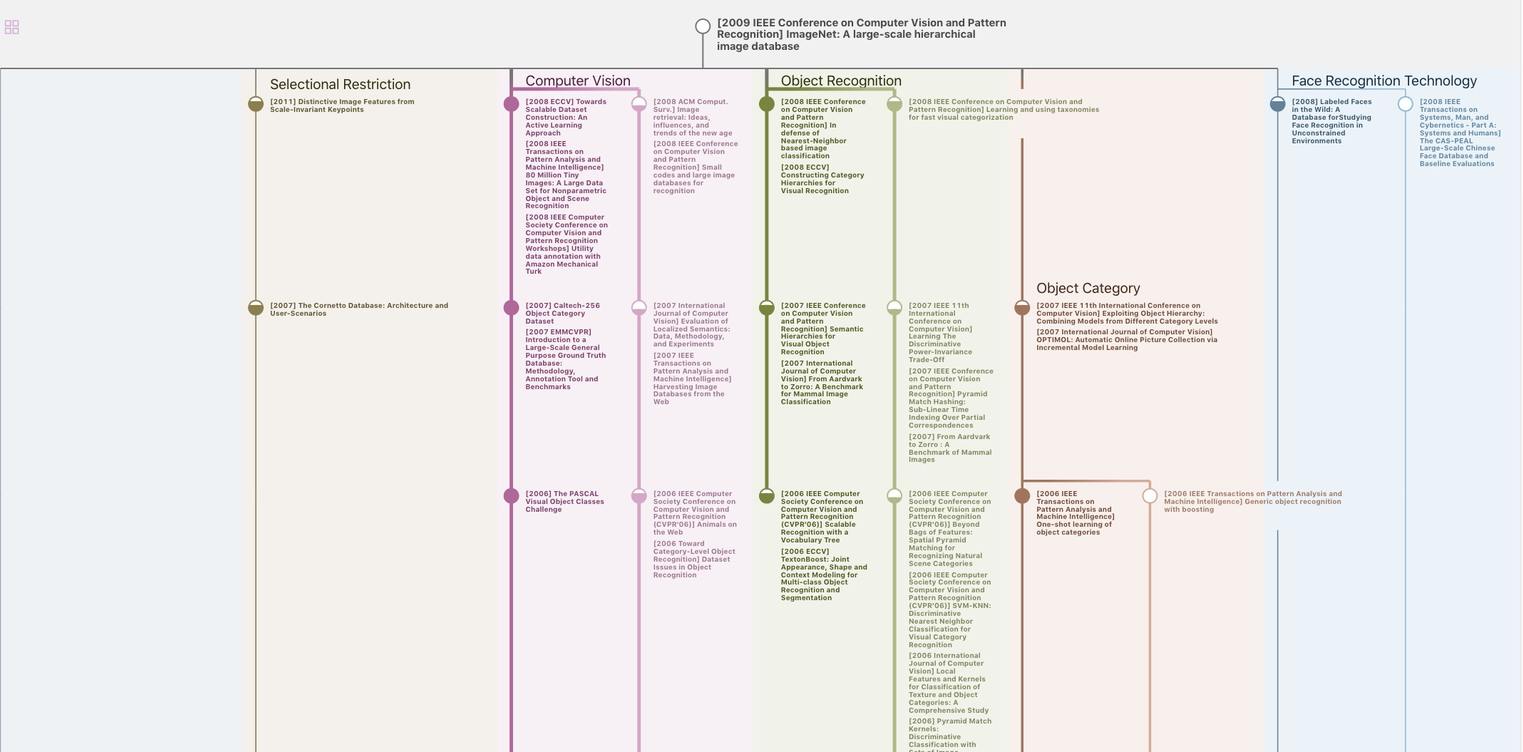
Generate MRT to find the research sequence of this paper
Chat Paper
Summary is being generated by the instructions you defined