Analysis of the spike responses in the neuromorphic implementation of the two-compartmental model of hippocampal pyramidal neuron
Journal of Computational Science(2023)
摘要
Designing a low-power neuromorphic circuit for the main units of the nervous system, neurons, plays a crucial role in neural engineering and neuroprosthesis. Due to the CA1 pyramidal neurons’ specific characteristics, these neurons have been a focus of research for several years. The Pinsky-Rinzel (P-R) model is a nonlinear two-compartmental model of the Hippocampal neuron that reveals various modes of firing patterns. In this paper, a low-power neuromorphic circuit for the Hippocampal P-R model is designed at 180 nm TSMC technology. Then, we analyze the recorded spiking responses from the neuroscience point of view. We also quantitatively compare the P-R model simulated in MATLAB with the proposed circuit in the Cadence simulator to show that the obtained responses are in good agreement. For the proposed circuit, the maximum energy consumption per spike is 97pJ/spike in the worst case. The effect of the device mismatch is also studied by performing a 200-point Monte Carlo simulation for different values of input currents. It is demonstrated that the proposed circuit is robust under process variation. Next, considering temporal coding, the ISI (inter-spike interval) and VP-d (Victor-Purpura distance) are extracted from the spike responses collected from the designed circuit. Finally, utilizing machine-learning algorithms, the applied input current to the circuit was accurately identified from the recorded spike trains. In this way, the spike patterns produced by the designed P-R circuit are diverse and consistent to be able to decode the input stimulus using typical machine learning classifiers. This supports the application of the proposed circuit in the hybrid neurocomputing systems and thus it can be integrated easily into large neuromorphic arrays in future works.
更多查看译文
关键词
Spike analysis,Compartmental model,Neuromorphic circuit,Temporal coding
AI 理解论文
溯源树
样例
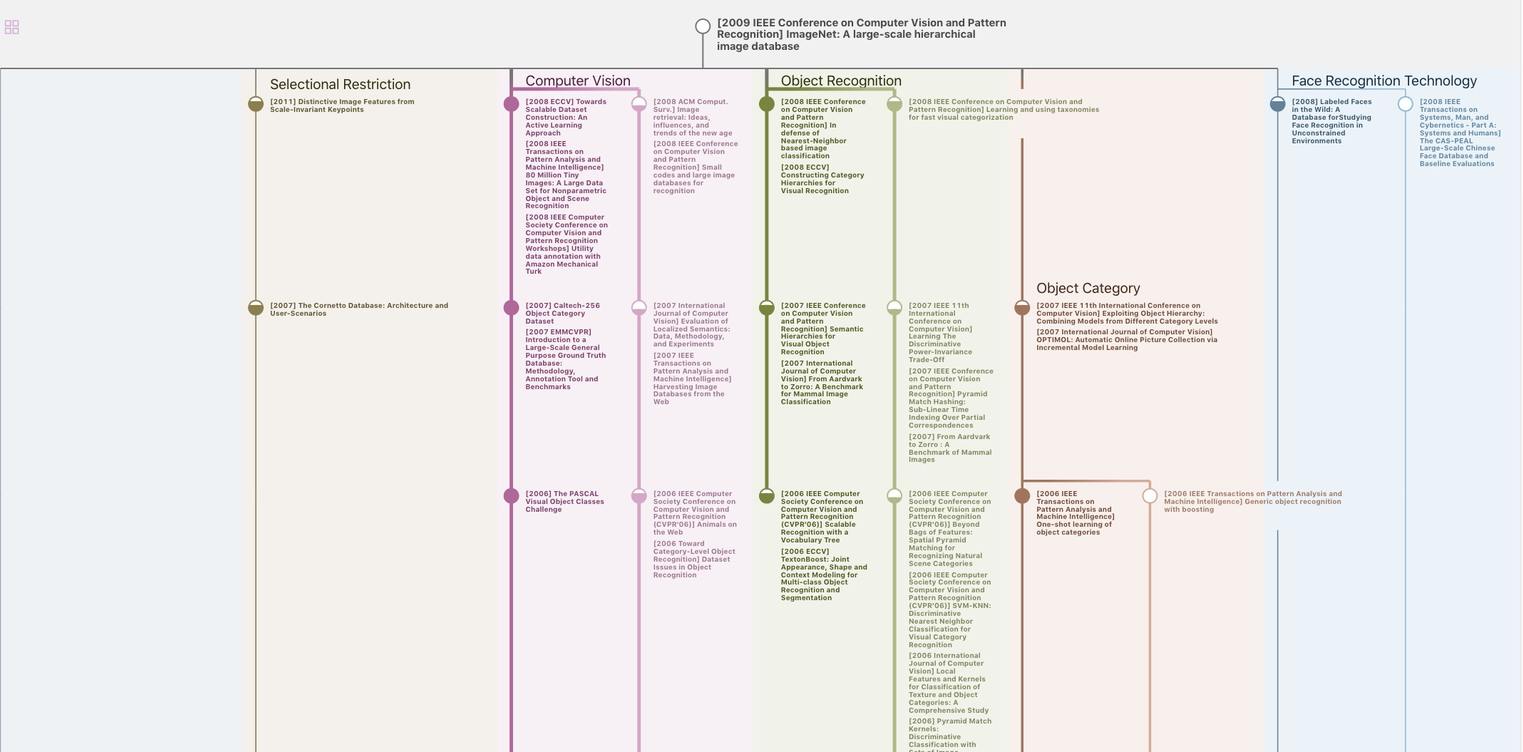
生成溯源树,研究论文发展脉络
Chat Paper
正在生成论文摘要