Mapping of snow water equivalent by a deep-learning model assimilating snow observations
Journal of Hydrology(2023)
Abstract
To estimate snowpack water storage in mountain basins, this study presents a framework that couples a deep -learning long short-term memory (LSTM) model and a zonal bias-correction approach for assimilating ground snow observations. We explored the framework's ability for snow water equivalent (SWE) mapping and fore-casting in a domain, including the Feather, Yuba-Bear, and American River basins in California's Sierra Nevada. Through a series of different modeling experiments, performances of the LSTM model and bias-correction approach were independently investigated. The LSTM model trained with meteorological forcing (precipita-tion and temperature), landscape attributes, and antecedent lagged snow conditions, showed good agreement with SWE reanalysis data (Nash-Sutcliffe efficiency, NSE = 0.99). However, for continuous SWE projection without using SWE reanalysis as the antecedent snow condition, the LSTM model underestimated basin-scale and site-scale SWE (NSE = 0.19), reflecting large discrepancies since model bias accumulates over time. Thus, a zonal inverse-distance-weighting (IDW) bias-correction approach was proposed to assimilate ground observations and correct model trajectory. With the bias correction, SWE estimates were significantly improved (NSE = 0.79). The zonal IDW approach accounts for snow differences across different areas by zonal distance, based on historical snow patterns, showing better predictions than the original IDW, which showed larger biases in complex terrain with a rain shadow. We observed that SWE estimates from the framework were not sensitive to the difference in precipitation and temperature forcings between observation-based data and one-day-ahead weather forecasts, suggesting that the framework can use weather-forecast forcing for snowpack forecasting. Comparison between results from monthly and daily bias corrections showed that increasing bias-correction frequency and integrating more snow observations can further improve SWE estimates. Using the continuous SWE products, analyses of 170 precipitation events showed that snowmelt often occurred when daily temperature was above 3.7 degrees C, aug-menting runoff. The proposed framework leverages ground observations and a deep-learning model to provide daily gridded snowpack estimates, which are important for runoff forecasting and water supply.
MoreTranslated text
Key words
Snowpack,Snowmelt,Bias correction,Inverse-distance weight,LSTM model
AI Read Science
Must-Reading Tree
Example
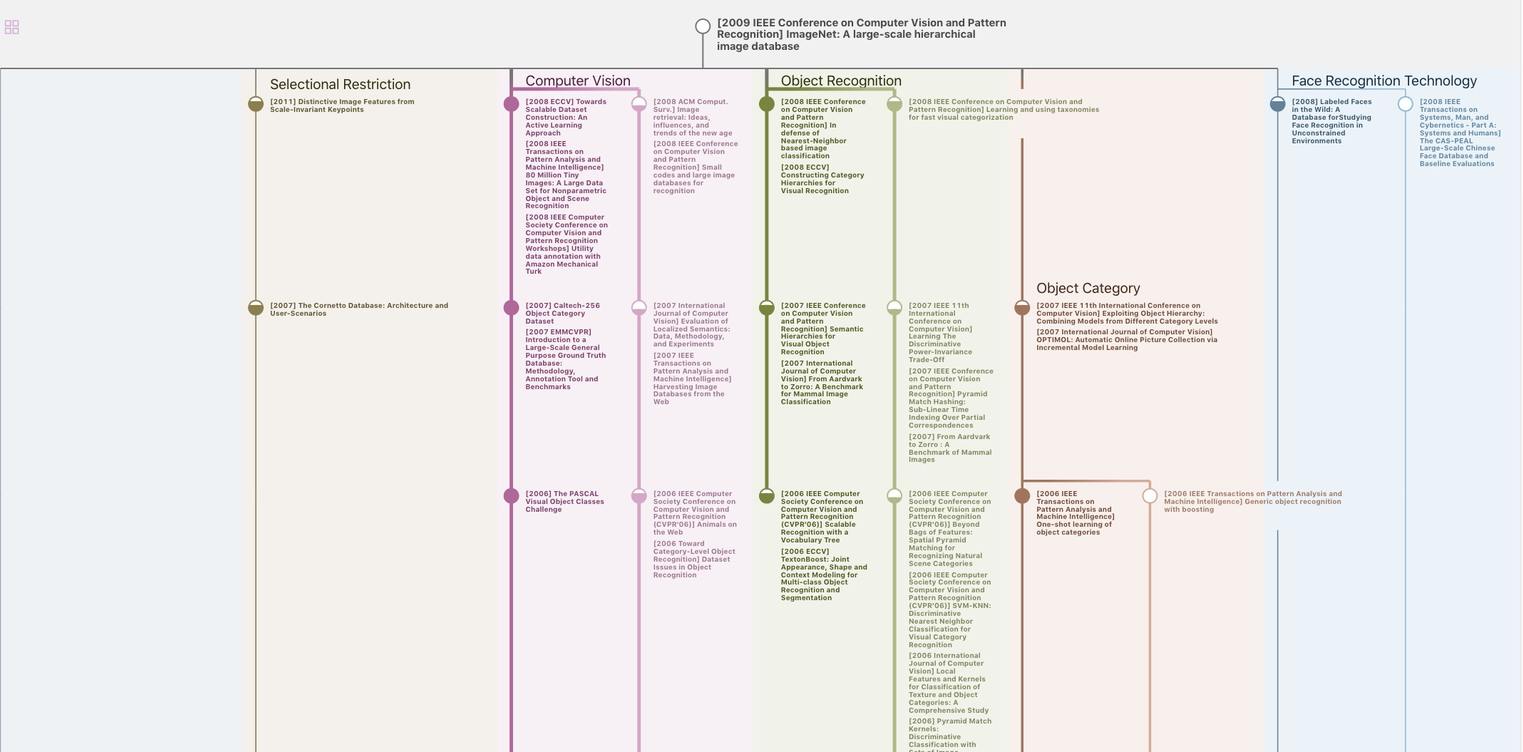
Generate MRT to find the research sequence of this paper
Chat Paper
Summary is being generated by the instructions you defined