Eigen-Entropy: A metric for multivariate sampling decisions
Information Sciences(2023)
摘要
Sampling is a technique to help identify a representative data subset that captures the characteristics of the whole dataset. Most existing sampling algorithms require distribu-tion assumptions of the multivariate data, which may not be available beforehand. This study proposes a new metric called Eigen-Entropy (EE), which is based on information entropy for the multivariate dataset. EE is a model-free metric because it is derived based on eigenvalues extracted from the correlation coefficient matrix without any assumptions on data distributions. We prove that EE measures the composition of the dataset, such as its heterogeneity or homogeneity. As a result, EE can be used to support sampling deci-sions, such as which samples and how many samples to consider with respect to the appli-cation of interest. To demonstrate the utility of the EE metric, two sets of use cases are considered. The first use case focuses on classification problems with an imbalanced data -set, and EE is used to guide the rendering of homogeneous samples from minority classes. Using 10 public datasets, it is demonstrated that two oversampling techniques using the proposed EE method outperform reported methods from the literature in terms of preci-sion, recall, F-measure, and G-mean. In the second experiment, building fault detection is investigated where EE is used to sample heterogeneous data to support fault detection. Historical normal datasets collected from real building systems are used to construct the baselines by EE for 14 test cases, and experimental results indicate that the EE method out-performs benchmark methods in terms of recall. We conclude that EE is a viable metric to support sampling decisions.(c) 2022 Published by Elsevier Inc.
更多查看译文
关键词
Information entropy,Correlation coefficient,Eigenvalues,Sampling,Model-free
AI 理解论文
溯源树
样例
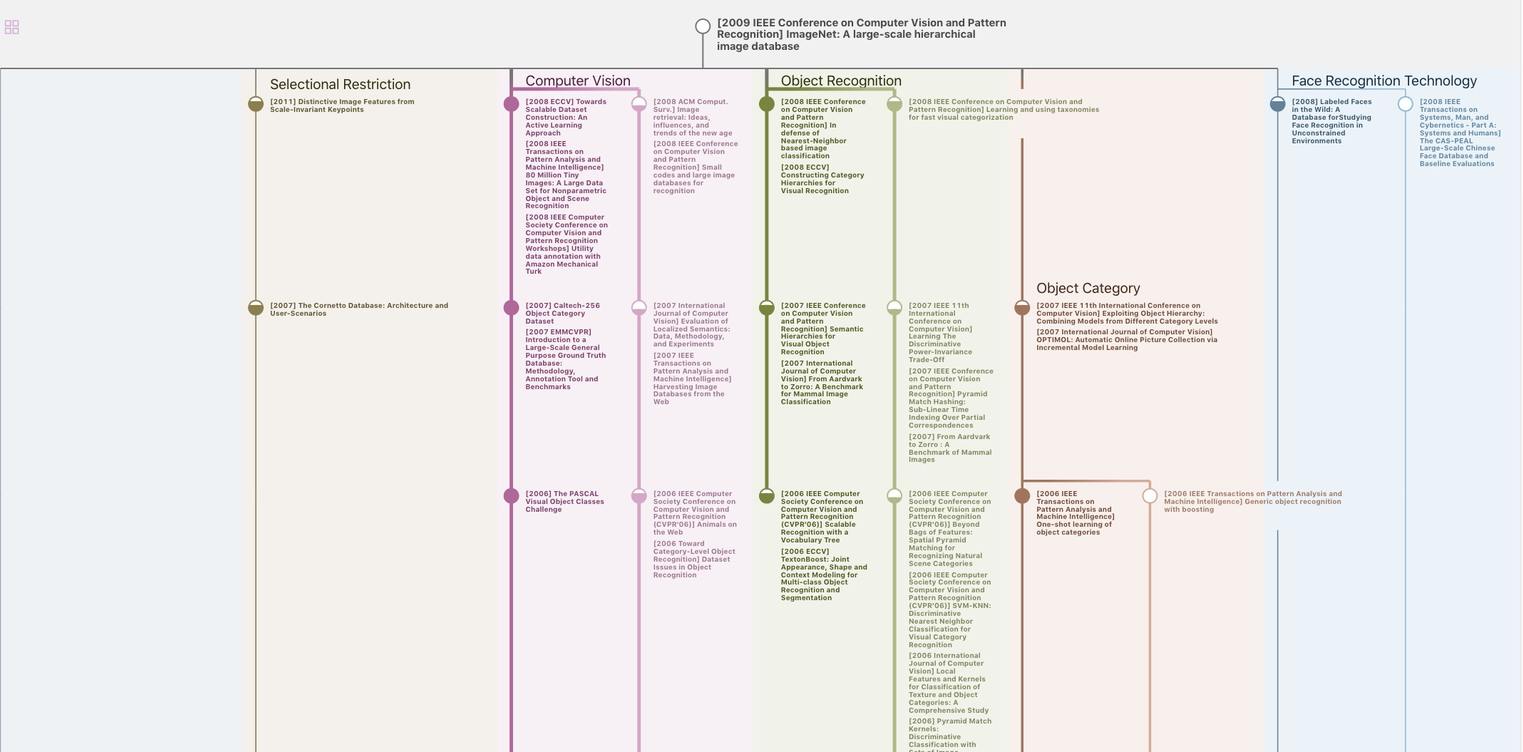
生成溯源树,研究论文发展脉络
Chat Paper
正在生成论文摘要