A genetic algorithm for multivariate missing data imputation
Information Sciences(2023)
Abstract
Some data mining, AI and data processing tasks might have data loss whose estimation/imputation is an important problem to be solved. Genetic algorithms are efficient and flexible global optimization methods able to deal with both multiple missing observations and multiple features such as continuous/discrete/binary data which are often found in multivariate databases unlike classical missing data estimation methods which only deal with univariate–continuous data. This paper presents a genetic algorithm to impute multiple missing observations in multivariate data which minimizes a new multi–objective (fitness) function based on the Minkowski distance of the means, variances, covariances and skewness between available/completed data. To do so, two sets of examples were tested: a continuous/discrete dataset which is compared to both the EM algorithm and auxiliary regressions, and a comparison over seven benchmark datasets.
MoreTranslated text
Key words
Missing data,Genetic algorithms,Multivariate missing data,Data imputation
AI Read Science
Must-Reading Tree
Example
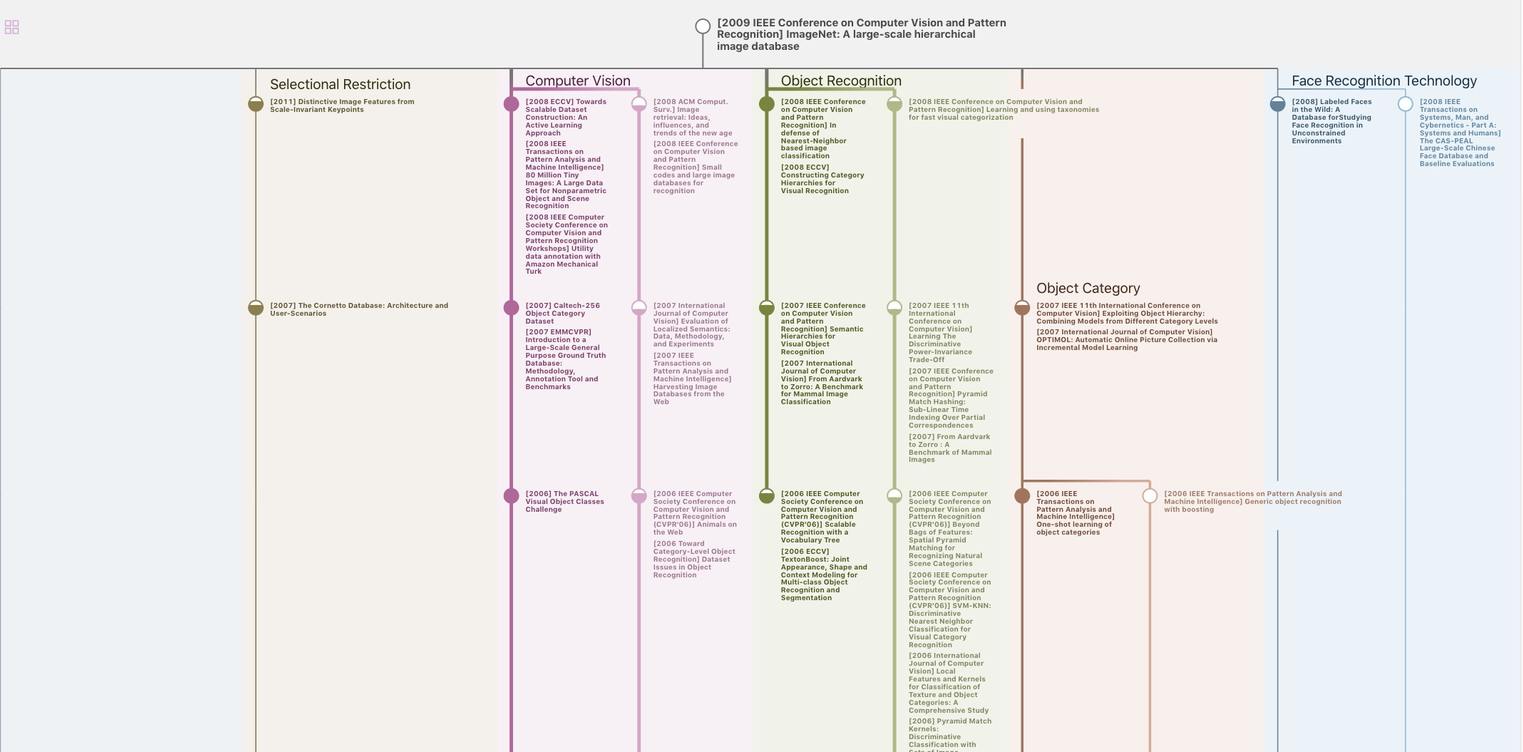
Generate MRT to find the research sequence of this paper
Chat Paper
Summary is being generated by the instructions you defined