Adaptive Impulsive Noise Suppression: A Deep Learning-Based Parameters Estimation Approach
IEEE TRANSACTIONS ON BROADCASTING(2022)
摘要
In order to obtain favorable communication performance, impulsive noise needs to be suppressed which is a major interference in scenarios such as broadcasting system and power line communication. In this paper, a time-domain impulsive noise suppression method is combined with deep learning, whose parameters are calculated by neural networks. The parameters of the denoising method are of great significance because they have a direct influence on the performance of impulsive noise suppression. By constructing a proper training set, trained neural networks can avoid the huge cost compared with traversal algorithm for determining parameters. In the meanwhile, the networks are able to estimate adaptive parameters under various noise environment avoiding fixed parameters. Furthermore, The computing resources of terminal devices are usually insufficient for a large number of calculations. Therefore, the noise suppression algorithm embedded in terminal equipment is expected to be with low complexity and achieve superior effects. Simulation experiments are carried out concerning signal to interference plus noise ratio and data rate. Simulation results of the deep learning based impulsive noise suppression scheme validate that neural networks have favorable performance in predicting parameters for the denoising algorithm.
更多查看译文
关键词
Impulsive noise suppression, deep learning, neural network, computing resource
AI 理解论文
溯源树
样例
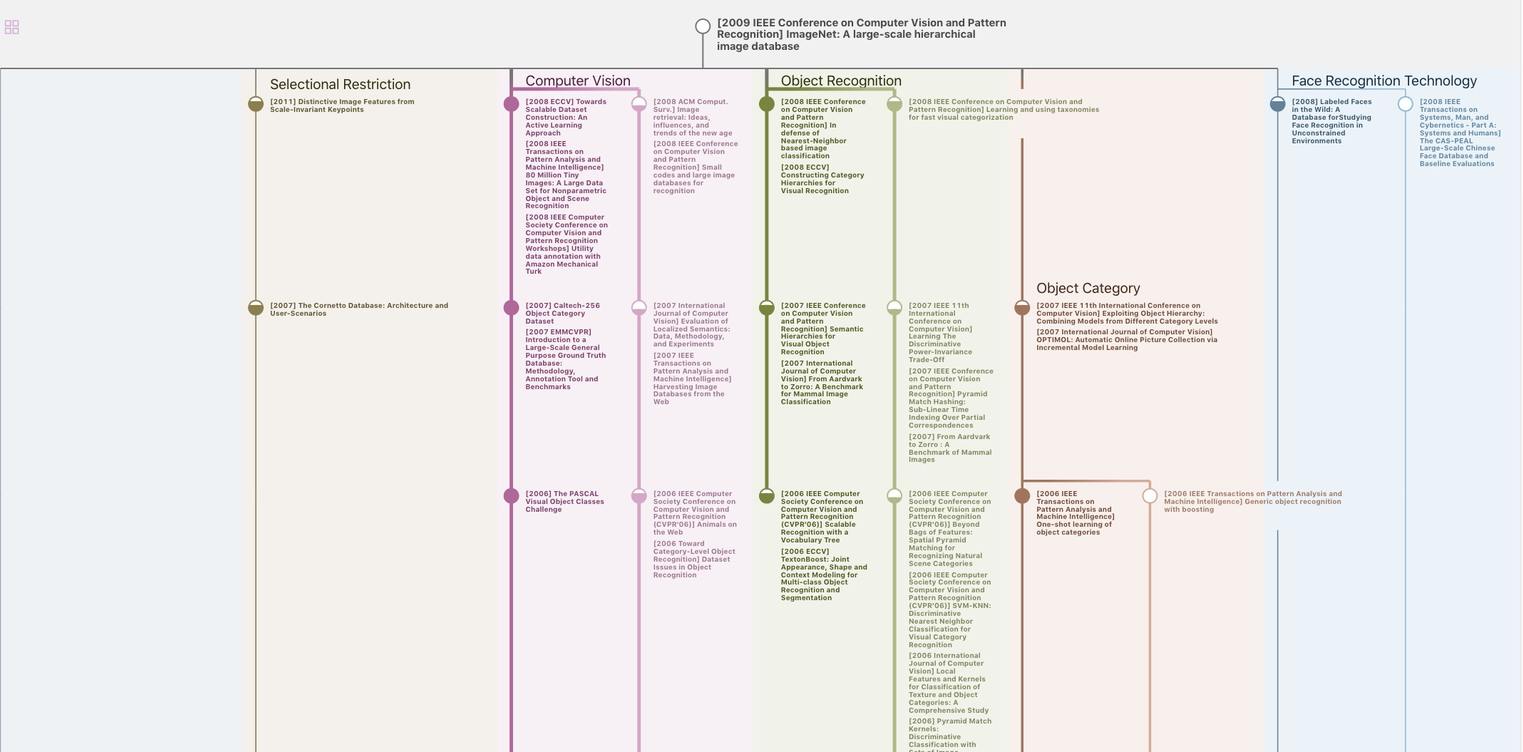
生成溯源树,研究论文发展脉络
Chat Paper
正在生成论文摘要