Energy Pattern Classification and Prediction in an Educational Institution using Deep Learning Framework
ELECTRIC POWER COMPONENTS AND SYSTEMS(2022)
Abstract
Building Energy Management is the most promising, trending, and essential way to enhance Building energy performance but it varies from one system to another. It can be achieved only by extracting the entire knowledge of the system. For this, a deep learning framework is proposed. The framework visualizes the Energy profiles of various workspaces in a building and also accounts for the Generating sources feeding them. It imparts awareness among consumers on effective handling of available Energy sources. kRNN-LSTM is the proposed framework applied to real-time Smart energy meter data observed in the Two-storeyed Electrical department building block of Thiagarajar College of Engineering, Madurai. k-means clustering is done to achieve the underlying pattern uniformities while RNN based LSTM gives a one-month ahead prediction. The LSTM model is compared with the Machine learning-based ARIMA model and basic Naive time series model by employing quality metrics computed from each model. The proposed model gives 94% accuracy and the reason behind the superiority of the model is due to temporal analytics dependencies and application of sliding window concept to Data for updating and learning the patterns of energy usage. In addition to this, the framework also points out some sort of information such as workspaces with higher energy profiles, Annual peaks in consumption, duration, and range of existence of peak demand, preferable Generating source on a cost basis, etc., Moreover, a suggestion for reducing the cost involved in Energy utilization is also depicted.
MoreTranslated text
Key words
Building Energy Management (BEM), building energy performance, deep learning framework, energy demand prediction, pattern defining, smart meter technology
AI Read Science
Must-Reading Tree
Example
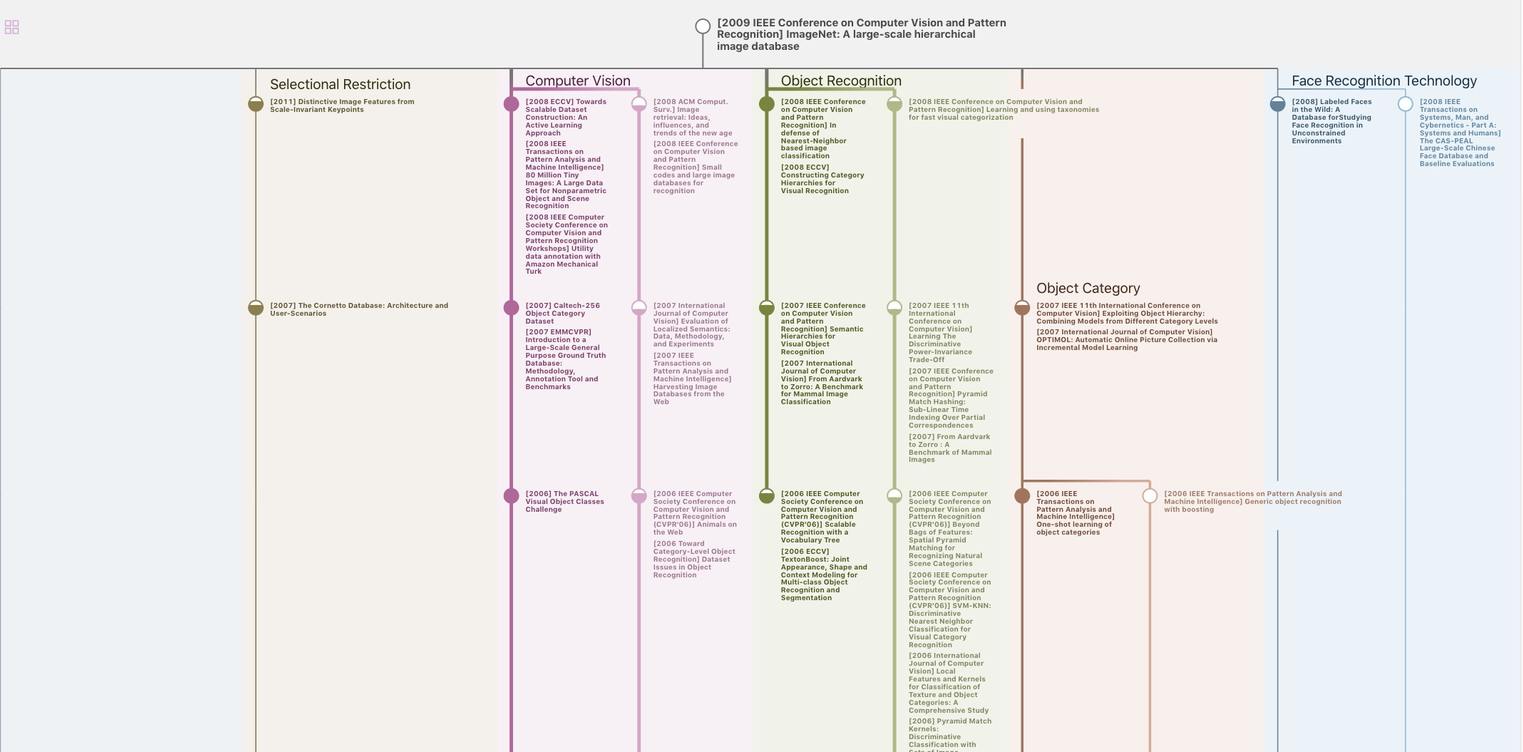
Generate MRT to find the research sequence of this paper
Chat Paper
Summary is being generated by the instructions you defined