Identification of Nontechnical Losses in Distribution Systems Adding Exogenous Data and Artificial Intelligence
ENERGIES(2022)
摘要
Nontechnical losses (NTL) are irregularities in the consumption of electricity and mainly caused by theft and fraud. NTLs can be characterized as outliers in historical data series. The use of computational tools to identify outliers is the subject of research around the world, and in this context, artificial neural networks (ANN) are applicable. ANNs are machine learning models that learn through experience, and their performance is associated with the quality of the training data together with the optimization of the model's architecture and hyperparameters. This article proposes a complete solution (end-to-end) using the ANN multilayer perceptron (MLP) model with supervised classification learning. For this, data mining concepts are applied to exogenous data, specifically the ambient temperature, and endogenous data from energy companies. The association of these data results in the improvement of the model's input data that impact the identification of consumer units with NTLs. The test results show the importance of combining exogenous and endogenous data, which obtained a 0.0213 improvement in ROC-AUC and a 6.26% recall (1).
更多查看译文
关键词
nontechnical losses, outliers identification, exogenous data, power system distribution, artificial neural networks, hyperparameter optimization, data mining, big data
AI 理解论文
溯源树
样例
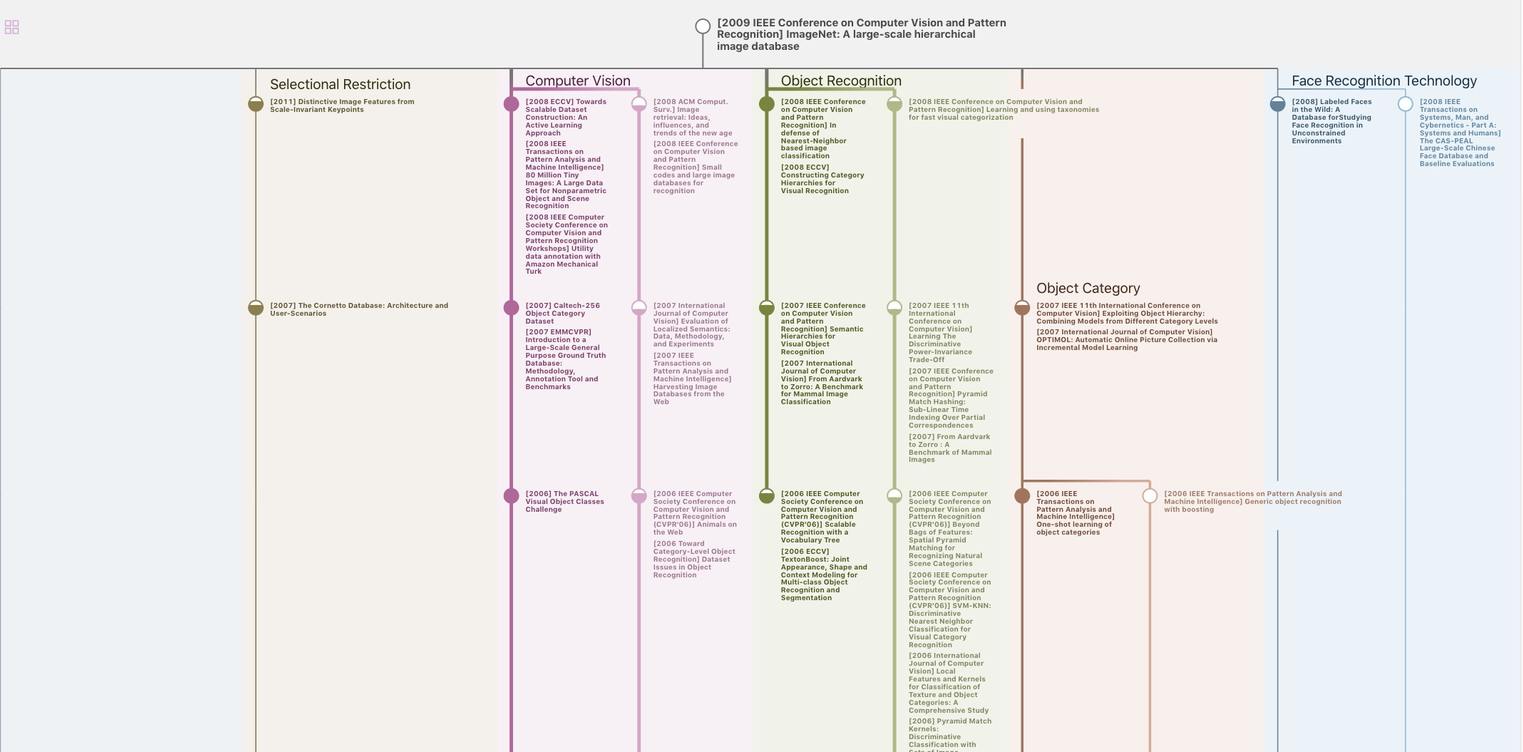
生成溯源树,研究论文发展脉络
Chat Paper
正在生成论文摘要