Evaluation of linear, nonlinear and ensemble machine learning models for landslide susceptibility assessment in southwest China
GEOCARTO INTERNATIONAL(2022)
摘要
Machine learning models are gradually replacing traditional techniques used for landslide susceptibility assessment. This study aims to comprehensively compare multiple models, including linear, nonlinear, and ensemble models, based on 5281 historical landslides in southwest China, the area most severely affected by the landslide disaster. Linear models represented by logistic regression (LR), nonlinear models represented by support vector machine (SVM), artificial neural network (ANN) and classification 5.0 decision tree (C5.0 DT), and ensemble models represented by random forest (RF) and categorical boosting (Catboost) were selected. The correlation coefficient, variance inflation factor (VIF), and relative important analysis were used to select the dominate landslide conditioning factors. Using multiple statistical indicators (e.g. Area Under the Receiver Operating Characteristic curve (AUC) and Kappa), cross-validation and qualitative methods to evaluate the models' performance. The findings are: (1) Regarding the model predictive performance, the best predictive performance was demonstrated by the ensemble models Catboost (AUC = 0.823 and Kappa = 0.593) and RF (AUC = 0.821 and Kappa = 0.582), followed by the nonlinear models SVM (AUC = 0.775 and Kappa = 0.520), ANN (AUC = 0.770 and Kappa = 0.486) and C5.0 DT (AUC = 0.751 and Kappa = 0.497), while the linear model LR (AUC = 0.756 and Kappa = 0.456) had a more limited performance. The ensemble model, which uses a tree as its baseline classifier, has a lot of potential for studies into the landslide susceptibility. (2) Regarding the model robustness, the three types of models in nonspatial cross-validation (CV) performed relatively similarly in terms of predictive power, while in spatial cross-validation (SPCV), the linear model LR (median AUC = 0.714) achieved better results than the ensemble and nonlinear models. It implies that when the distribution of landslides is not homogeneous, linear models may be the most robust. It is advisable to consider various evaluation metrics from different perspectives and integrate them with specialist qualitative geomorphological empirical knowledge to determine the best model. (3) The Gini index-based RF model suggests that road density was the dominant factor in the frequency of landslides in the study area.
更多查看译文
关键词
Evaluation of machine learning models, cross-validation, landslide susceptibility assessment, southwest China
AI 理解论文
溯源树
样例
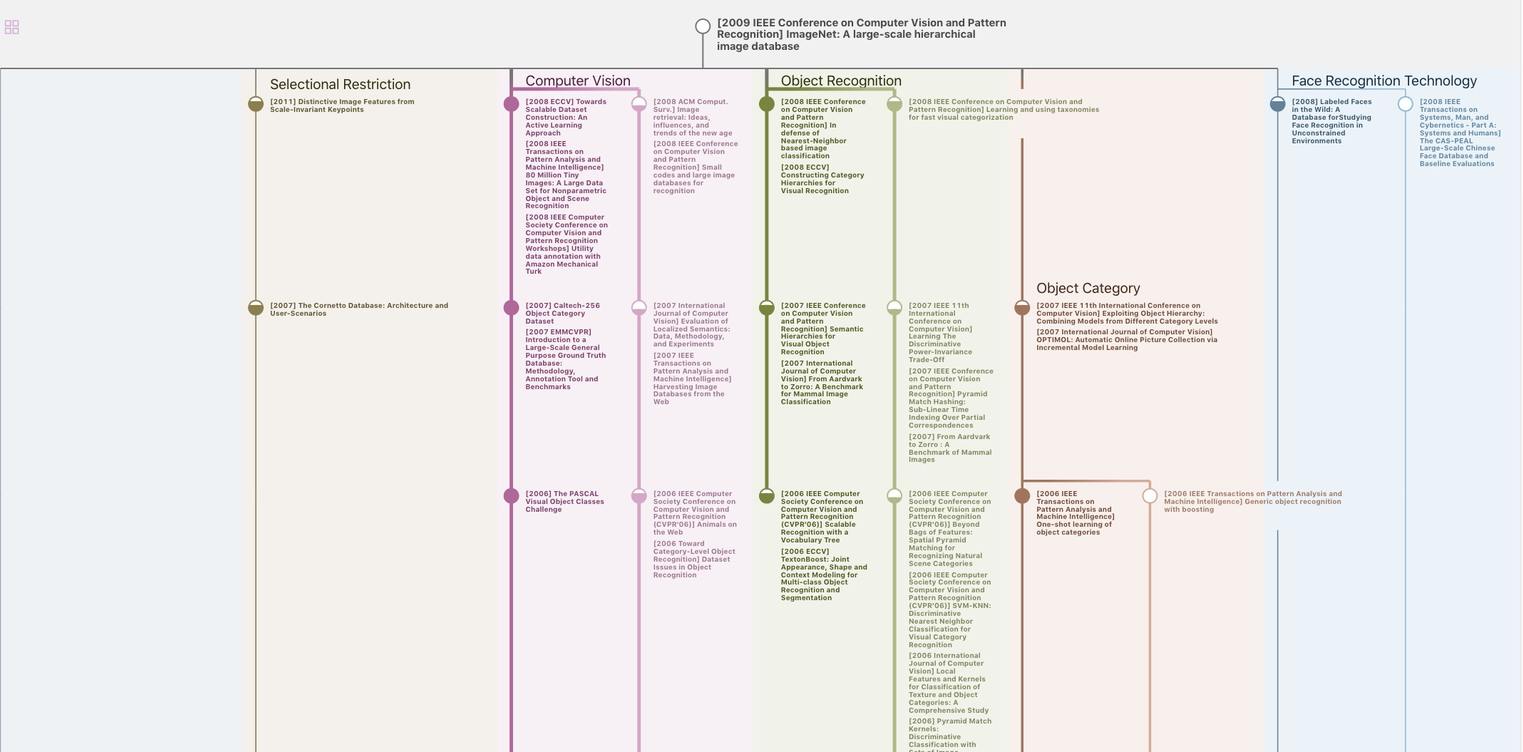
生成溯源树,研究论文发展脉络
Chat Paper
正在生成论文摘要