FIMF score-CAM: Fast score-CAM based on local multi-feature integration for visual interpretation of CNNS
IET IMAGE PROCESSING(2023)
Abstract
The interpretability of the model is a hot issue in the field of computer vision. Score-CAM is a kind of interpretable CAM method with good discrimination and gradient free calculation. It is a representative work in this field. However, it has the disadvantages of long calculation time and incomplete heatmap coverage. Therefore, this paper proposes an improved Score-CAM method named FIMF Score-CAM, which can fast integrate multiple features. Its contribution is reflected in two aspects: The weight of the feature map is directly calculated by using the feature template. Unlike Score-CAM, this model greatly saves computation time because it only requires one convolutional calculation. Another contribution is that the feature map used to generate the heatmap integrates various semantic features of the local space, so that the heatmap of the object of interest can be generated with more complete coverage and better interpretation. The FIMF Score-CAM is superior to the existing mainstream models in interpreting the visual performance and fairness indicators of the decision-making, having more complete explanations of object classes and the advantage of faster calculation speed.
MoreTranslated text
Key words
class activation mapping,computer vision,deep network,model interpretation
AI Read Science
Must-Reading Tree
Example
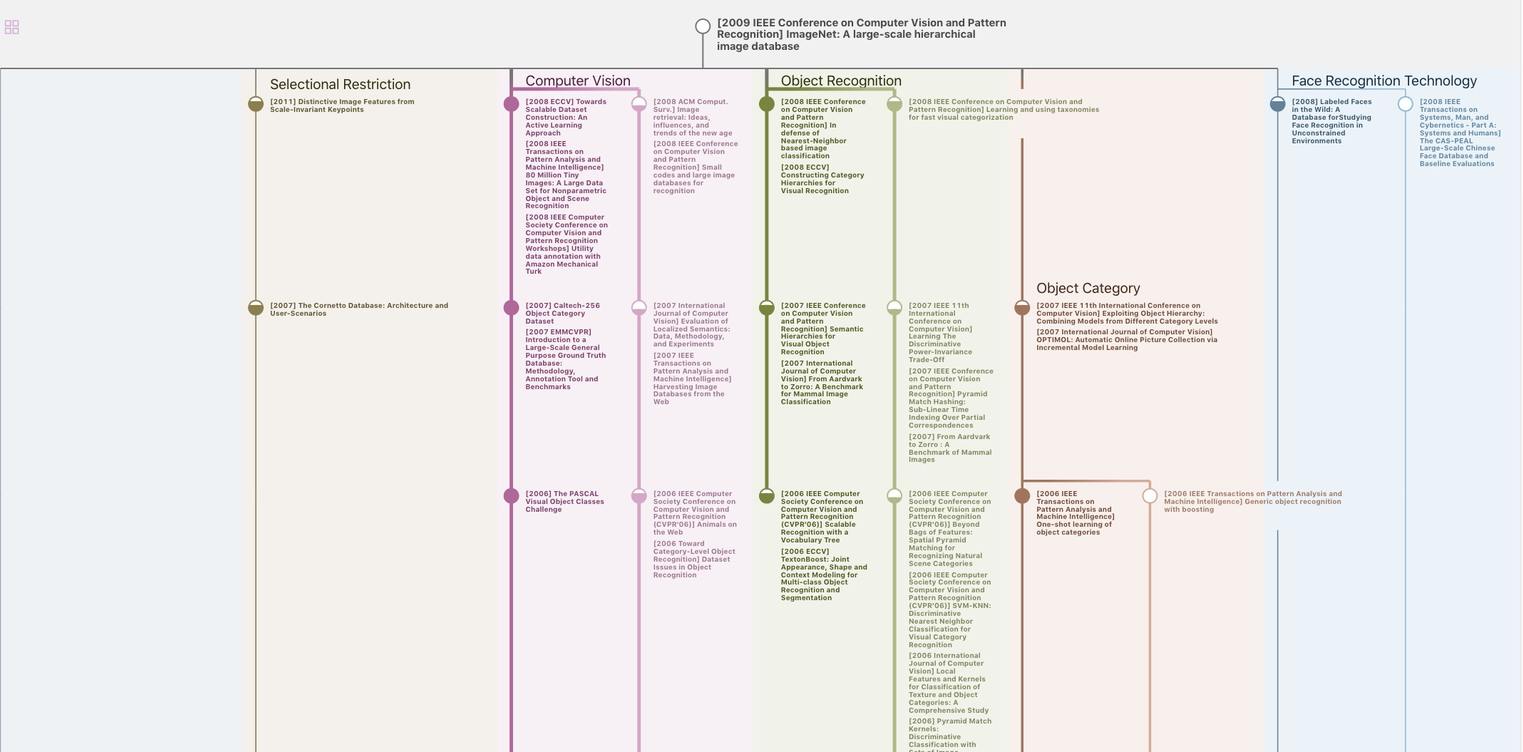
Generate MRT to find the research sequence of this paper
Chat Paper
Summary is being generated by the instructions you defined