A Hybrid Model for China's Soybean Spot Price Prediction by Integrating CEEMDAN with Fuzzy Entropy Clustering and CNN-GRU-Attention
SUSTAINABILITY(2022)
Abstract
China's soybean spot price has historically been highly volatile due to the combined effects of long-term massive import dependence and intricate policies, as well as inherent environmental elements. The accurate prediction of the price is crucial for reducing the amount of soybean-linked risks worldwide and valuable for the long-term sustainability of global agriculture. Therefore, a hybrid prediction model that combines component clustering and a neural network with an attention mechanism has been developed. After fully integrated complete ensemble empirical mode decomposition with adaptive noise (CEEMDAN) processing of the price series, the fuzzy entropy of each component is measured as the complexity characteristic. K-means clustering and reconstruction are applied to the components before being input to the CNN-GRU-Attention network for prediction to improve the model ability and adaptability of the sequences. In the empirical analysis, the proposed model outperforms other decomposition techniques and machine learning algorithms regarding prediction accuracy. After applying the decomposition part, the results have RMSE, MAPE, and MAE values of 49.59%, 22.58%, and 21.99% lower than those of the individual prediction part, respectively. This research presents a novel approach for market participants in the soybean industry for risk response. It gives a new perspective on agricultural product prices in sustainable agricultural marketing, while also providing practical tools for developing public policies and decision-making.
MoreTranslated text
Key words
soybean spot price,CNN-GRU-Attention,fuzzy entropy,CEEMDAN
AI Read Science
Must-Reading Tree
Example
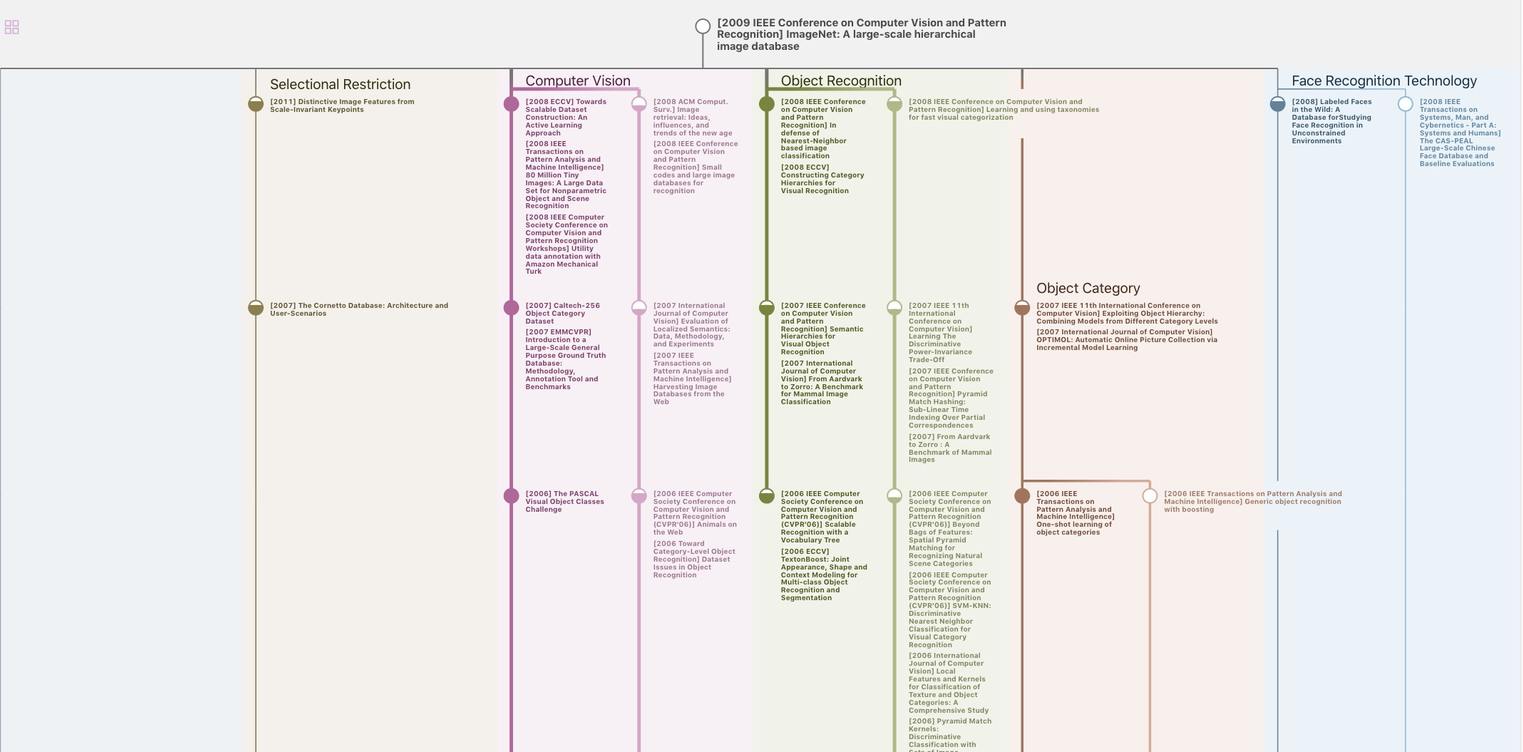
Generate MRT to find the research sequence of this paper
Chat Paper
Summary is being generated by the instructions you defined