A Novel Multi-Model Stacking Ensemble Learning Method for Metro Traction Energy Prediction
IEEE ACCESS(2022)
Abstract
Metro traction energy prediction is the basis of abnormal monitoring and plays an indispensable role in the planning and operation of the metro system. However, current studies rarely offer a satisfactory prediction performance. To improve the prediction accuracy, a novel prediction method for metro traction energy consumption is proposed based on gradient penalty Wasserstein generative adversarial network (WGAN-GP) and stacking ensemble learning with multi-model integration. Firstly, aiming to collect effective train data, WGAN-GP is used to generate characteristic data of traction energy consumption. Then, various algorithms like BP, SVM, ELM, and XGBoost are employed to preliminarily disclose the relationship between traction energy consumption and characteristic data of traction energy consumption via K-fold verification. Thereafter, the XGBoost algorithm is implemented as the meta model to construct a stacking ensemble learning prediction model. Finally, the proposed method is verified with data from Guangzhou Metro Line 13, and the results substantiate the effectiveness of the prediction model.
MoreTranslated text
Key words
Metro traction energy, gradient penalty, Wasserstein generative adversarial network, ensemble learning, prediction model
AI Read Science
Must-Reading Tree
Example
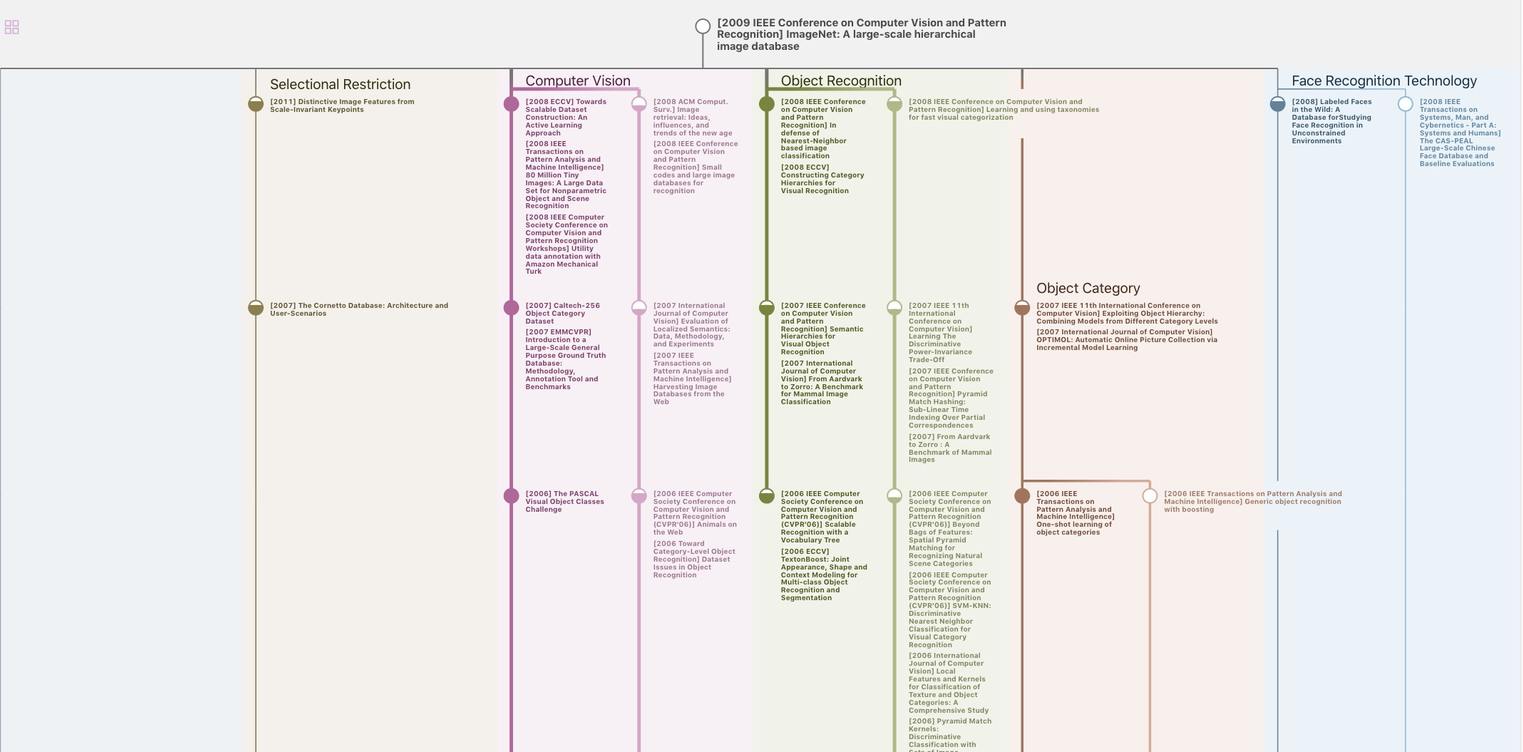
Generate MRT to find the research sequence of this paper
Chat Paper
Summary is being generated by the instructions you defined