Joint multimodal sentiment analysis based on information relevance
Information Processing & Management(2023)
摘要
Social media users are increasingly turning to express opinions with both images and text, while the visual content and text description may cover some conflicting information diverse from each other. Information relevance refers to the matching degree between cross-modal features at the emotional semantic level, which is not systematically studied. In order to exploit the discriminative features and the internal correlation among different modalities, the mid-level representation extracted by a visual sentiment concept classifier is used to determine information relevance, with the integration of other features, including attended textual and visual features. Then grid search is applied to tune weighting coefficients of the decision fusion scheme, followed by a multimodal adaptive method for joint sentiment analysis based on image -text relevance. The superiority of our architecture approach is demonstrated experimentally by comparing it with several state-of-the-art baselines, such as the vision-aware language modeling approach and contrastive learning-based model. The results indicate that fused multiple features lead to more precise classification than unimodal ones, while the contributions of each single modality differ obviously in emotional expression. Besides, the performance of every involved model varies markedly per dataset of different content correlation, which proves that it is of extensive theoretical significance and application prospect to introduce the image-text relevance classifier into a multimodal task.
更多查看译文
关键词
Network public opinion,Sentiment classification,Image-text relevance,Multimodal fusion,Multimodal deep learning
AI 理解论文
溯源树
样例
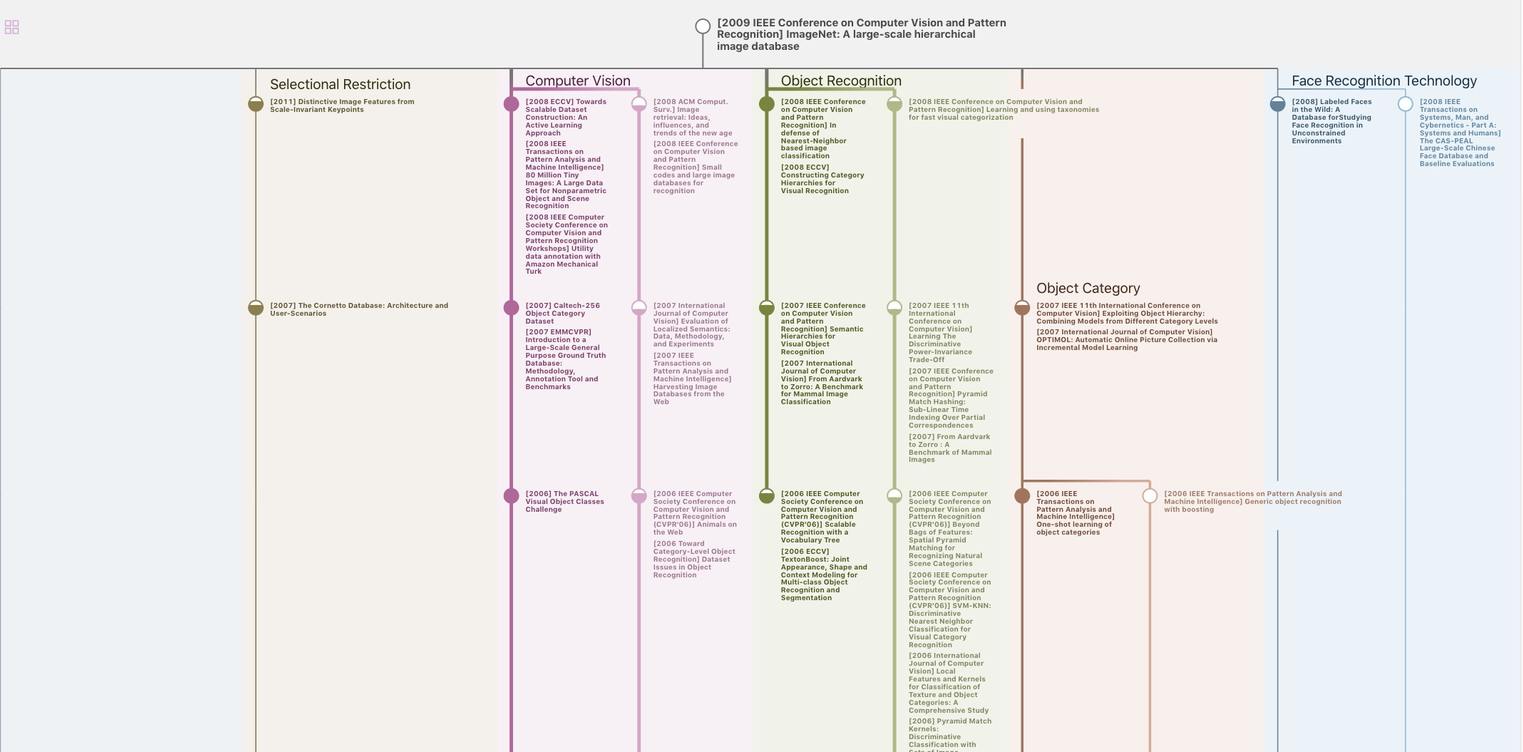
生成溯源树,研究论文发展脉络
Chat Paper
正在生成论文摘要