Parallel learned generative adversarial network with multi-path subspaces for cross-modal retrieval
Information Sciences(2023)
Abstract
Cross-modal retrieval aims to narrow the heterogeneity gap between different modalities, such as retrieving images through texts or vice versa. One of the key challenges of cross-modal retrieval is the inconsistent distribution across diverse modalities. Most existing methods tend to construct a common representation subspace to overcome the challenge. However, the supervision information is not fully explored in most single-path cross-modal learning approaches. In this paper, we present a novel Parallel Learned generative adversarial network with Multi-path Subspaces (PLMS) for cross-modal retrieval. PLMS is a parallel learned architecture that aims to capture more effective information in an end-to-end trained cross-modal retrieval model. To be specific, a dual-branch network is constructed in the modality-specific generator, thereby the overall framework learns two common subspaces to emphasize discrepant supervision information and preserve more effective transformed features. We further design two objective functions for the training of the dual branches in generators. Through joint training, the feature representations generated by dual branches in a specific modality are fused for similarity measurement between modalities. To avoid redundancy and overlap during fusion, a Multi-source Domain Balancing (MDB) mechanism is presented to explore the contribution of the two specific-task branches. Extensive experiments show that our proposed method is effective and achieves state-of-the-art results on four widely-used databases.
MoreTranslated text
Key words
Cross-modal retrieval,Generative adversarial network,Parallel learning architecture,Multi-path subspace,Multi-source domain balancing
AI Read Science
Must-Reading Tree
Example
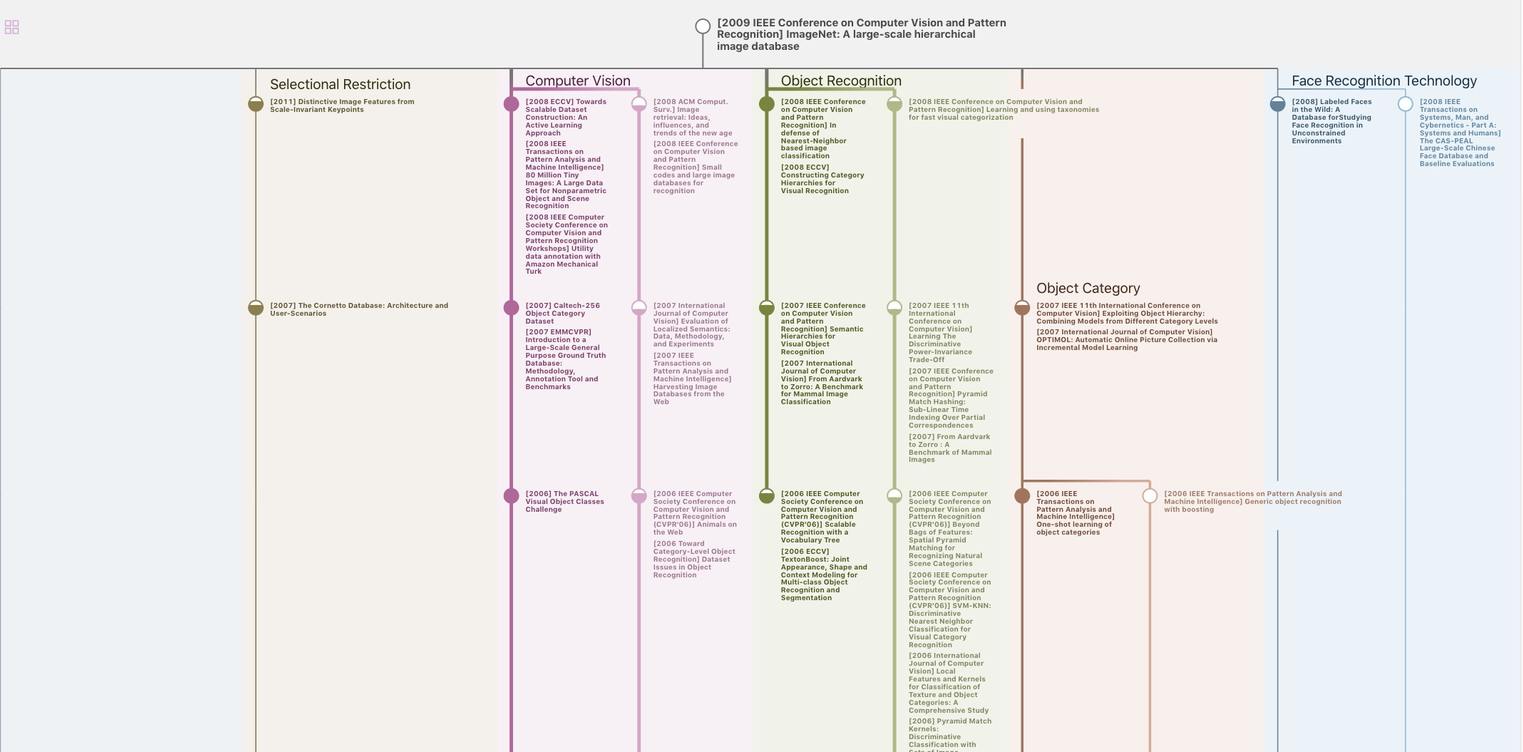
Generate MRT to find the research sequence of this paper
Chat Paper
Summary is being generated by the instructions you defined