MORE: Toward Improving Author Name Disambiguation in Academic Knowledge Graphs
INTERNATIONAL JOURNAL OF MACHINE LEARNING AND CYBERNETICS(2024)
摘要
Author name disambiguation (AND) is a fundamental task in knowledge alignment for building a knowledge graph network or an online academic search system. Existing AND algorithms tend to cause over-splitting and over-merging problems of papers, severely jeopardizing the performance of downstream tasks. In this paper, we demonstrate the problem of paper over-splitting and over-merging when constructing an academic knowledge graph. To address the problems, we systematically investigate and propose a unified architecture, MORE, which utilizes LightGBM and HAC FOR paper clusteRing as well as HGAT for both cluster alignmEnt and knowledge graph representation learning. Specifically, we first propose a novel representation learning method which leverages OAG-BERT to learn paper entity embedding and utilizes SimCSE to regularizes pre-trained embedding anisotropic space. We then apply LightGBM to calculate the similarity matrix of papers through entity embedding. We also use hierarchical agglomerative clustering (HAC) for grouping clusters to alleviate over-merging. Finally, considering co-author relationships, we improve the HGAT model using hard-cross graph attention mechanism to generate semantic and structural embedding. Experimental results on two large real-world datasets show that our proposed method achieves 6%similar to 16% improvement against the baseline models on F1-score.
更多查看译文
关键词
Name Disambiguation,Knowledge Graph,Knowledge Alignment,HGAT,Contrastive Learning
AI 理解论文
溯源树
样例
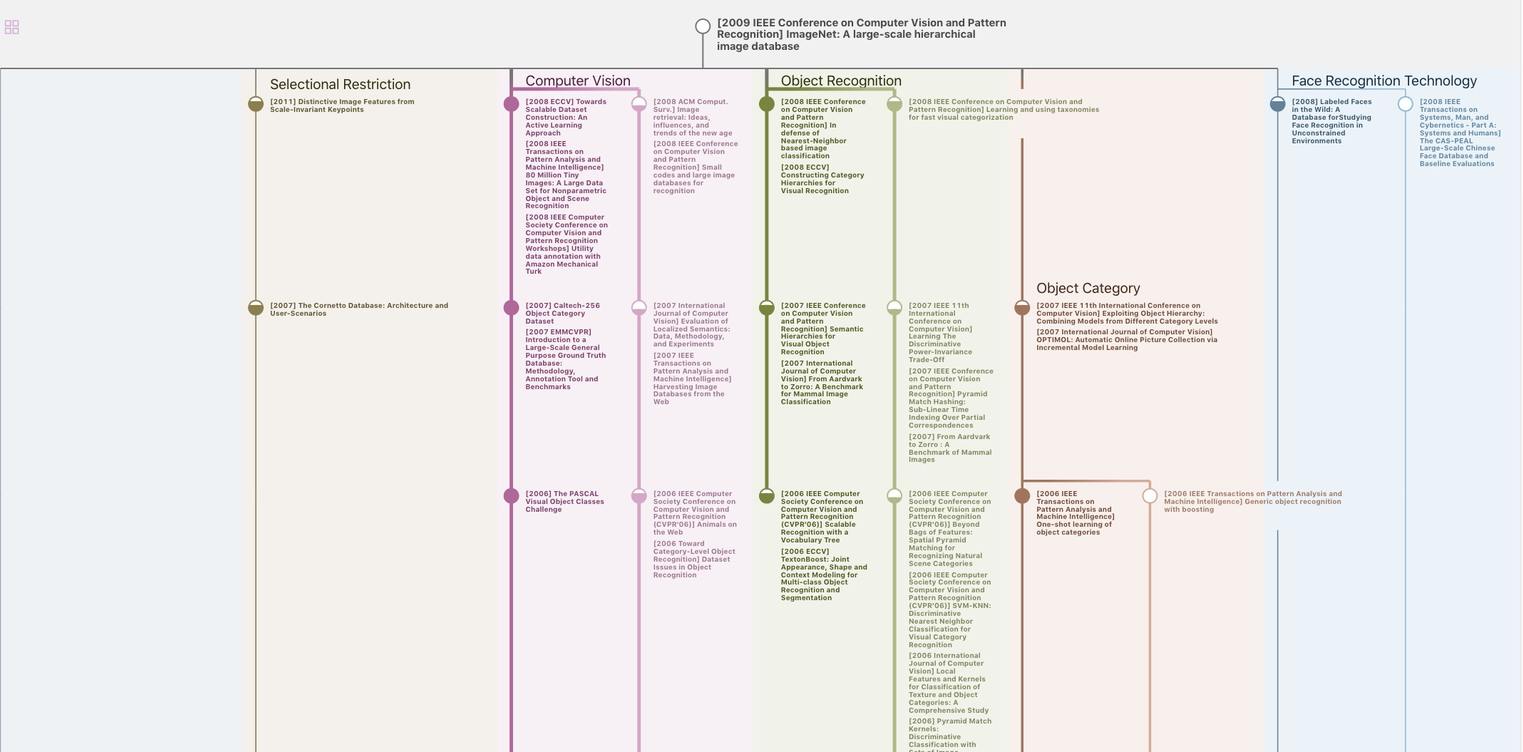
生成溯源树,研究论文发展脉络
Chat Paper
正在生成论文摘要