Estimating the differentiation potential and plasticity of glioblastoma cells using statistical mechanics
NEURO-ONCOLOGY(2022)
摘要
Abstract Glioblastoma (GBM) is the most common form of adult brain tumors and patients diagnosed with GBM face a very dismal prognosis with a median survival rate of 15 months. The challenges in treating GBM are many, there is a complex cellular and molecular heterogeneity, both between patients and within individual tumors. In a single tumor, individual cancer cells adopt a variety of stem-like, progenitor-like, and more differentiated phenotypes (a.k.a. cell states). Recent advances in single-cell technology have made it possible to detect this diversity of cellular states, but yet we lack a good explanation for how the states arise and if transitions between states can be exploited to therapeutic ends. We here propose a new computational strategy to measure the differentiation potential of GBM cells, based on the Ising models from statistical mechanics. Originally used to model coupled magnets on a lattice, the Ising models can be generalized to represent the probability of a particular configuration of any system of n variables. We show that the models can be fitted to high-dimensional single cell data in a matter of seconds by solving a convex optimization problem, and that the models help explain cell differentiation in terms of minimization of an energy function of the system. In our on-going work we have fitted the model to single cell data from our previous work on state transitions in GBM (Larsson, Dalmo et al, Molecular Systems Biology 2021) and shown that the predicted differentiation potential of cell states is consistent with the cell state hierarchies derived from lineage tracing experiments. Moving forward, we are now exploring how the Ising models can be used to model state transitions in GBM and to predict the effect of targeted therapy on the cell’s differentiation potential.
更多查看译文
关键词
glioblastoma cells,differentiation potential
AI 理解论文
溯源树
样例
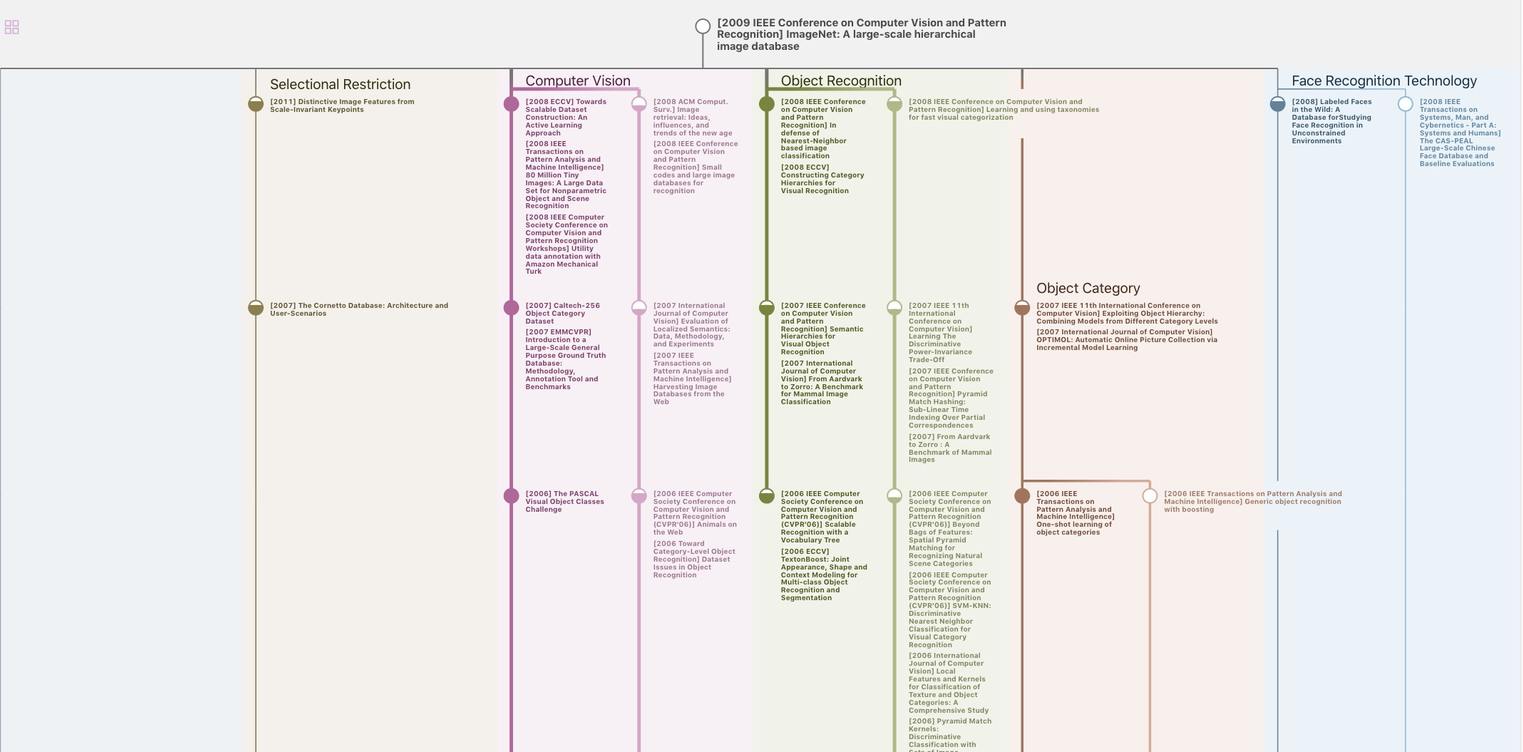
生成溯源树,研究论文发展脉络
Chat Paper
正在生成论文摘要