Deciphering raman-spectral tissue heterogeneity in glioblastoma
NEURO-ONCOLOGY(2022)
摘要
Abstract Raman Spectroscopy is able to provide a fast identification method for healthy and pathological tissues without the need for sample preparation. This makes it highly interesting for intraoperative use in identifying tumor types and borders. However, the capability of a machine-learning classifier to recognize known tissues based on Raman spectra relies on a solid ground truth that is usually provided by measuring small tissue samples first and analyzing the sample later by histopathology. This approach is limited in very closely interspersed tissues that cannot be identified macroscopically, such as glioblastoma where vital tumor tissue, necrosis and peritumoral tissue with characteristic changes to healthy brain tissue are tightly arranged. These areas have been shown to be distinct in their spectral properties, which makes tumor border identification aided by Raman Spectroscopy challenging. As infiltrative tumors leaving no tumor-cell-free brain tissue, borders need to be defined on a clinical scale, where extensive resection must be weighed against retaining functional brain tissue. For this, spectral analysis beyond tumor identification is necessary. In a computational approach we use methods of unsupervised learning and K-means Clustering to investigate heterogeneity within glioblastomas in the native state in order to reduce the complexity of this tumor type. Thereby, it was possible to extract spectra of necrotic tumor areas and to calculate a prediction probability for necrotic tissue within glioblastomas. Several clusters with spectral similarity to each other could be identified, which might represent the different areas of a glioblastoma. Two of these clusters were identified as probable grey and white matter, respectively, by comparison with autopsy tissue. In addition, this assignment independently confirmed the prediction of the classifier as non-necrotic tissue. Deciphering the spectral heterogeneity of glioblastoma in this way may be a great advantage for the surgeon to identify tumor borders and assist in resection control.
更多查看译文
AI 理解论文
溯源树
样例
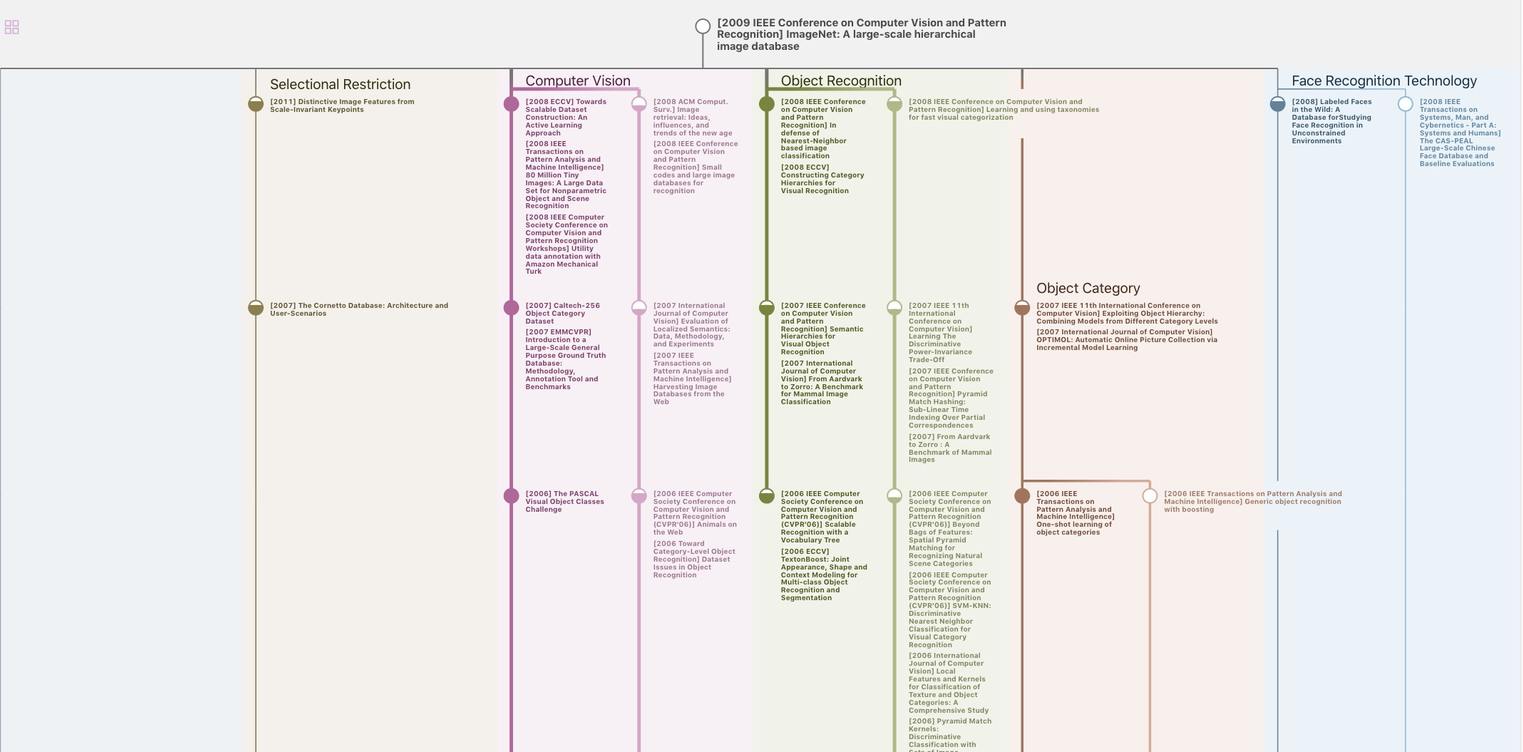
生成溯源树,研究论文发展脉络
Chat Paper
正在生成论文摘要