Deep learning enabled cross-lingual search with metaheuristic web based query optimization model for multi-document summarization
CONCURRENCY AND COMPUTATION-PRACTICE & EXPERIENCE(2023)
摘要
Due to the exponential increase in the generation of digital documents and in the online search user diversity, multilingual information is highly available on the Internet. However, the huge amount of multilingual data cannot be analyzed manually. Therefore, cross lingual multi-document summarization (CLMDS) model is introduced to generate a summary of several documents in which the summary language is different from the source document language. This paper presents a Deep Learning Enabled Cross-lingual Search with Metaheuristic based Query Optimization (DLCLS-MQO) model for Multi-document summarization. The DLCLS-MQO model allows to offer a query in Tamil, summarize several English documents, and lastly translate the summary into Tamil. The DLCLS-MQO model encompasses four stages of operation such as multilingual search, query optimization, automatic sematic lexicon builder, and document summarization. Firstly, bidirectional long short-term memory (BiLSTM) model is applied to perform multilingual searching process. Followed by, sunflower optimization (SFO) algorithm based query optimization process is carried out. Moreover, global vectors (GloVe) method is used for the construction of domain oriented sentiment lexicons. Finally, extreme gradient boosting (XGBoost) model is applied for the CLMDS. A detailed simulation analysis takes place to highlight the betterment of the DLCLS-MQO model. The resultant experimental values portrayed the superior performance of the DLCLS-MQO model over the compared methods.
更多查看译文
关键词
cross-lingual, deep learning, metaheuristics, multi-document summarization, query optimization
AI 理解论文
溯源树
样例
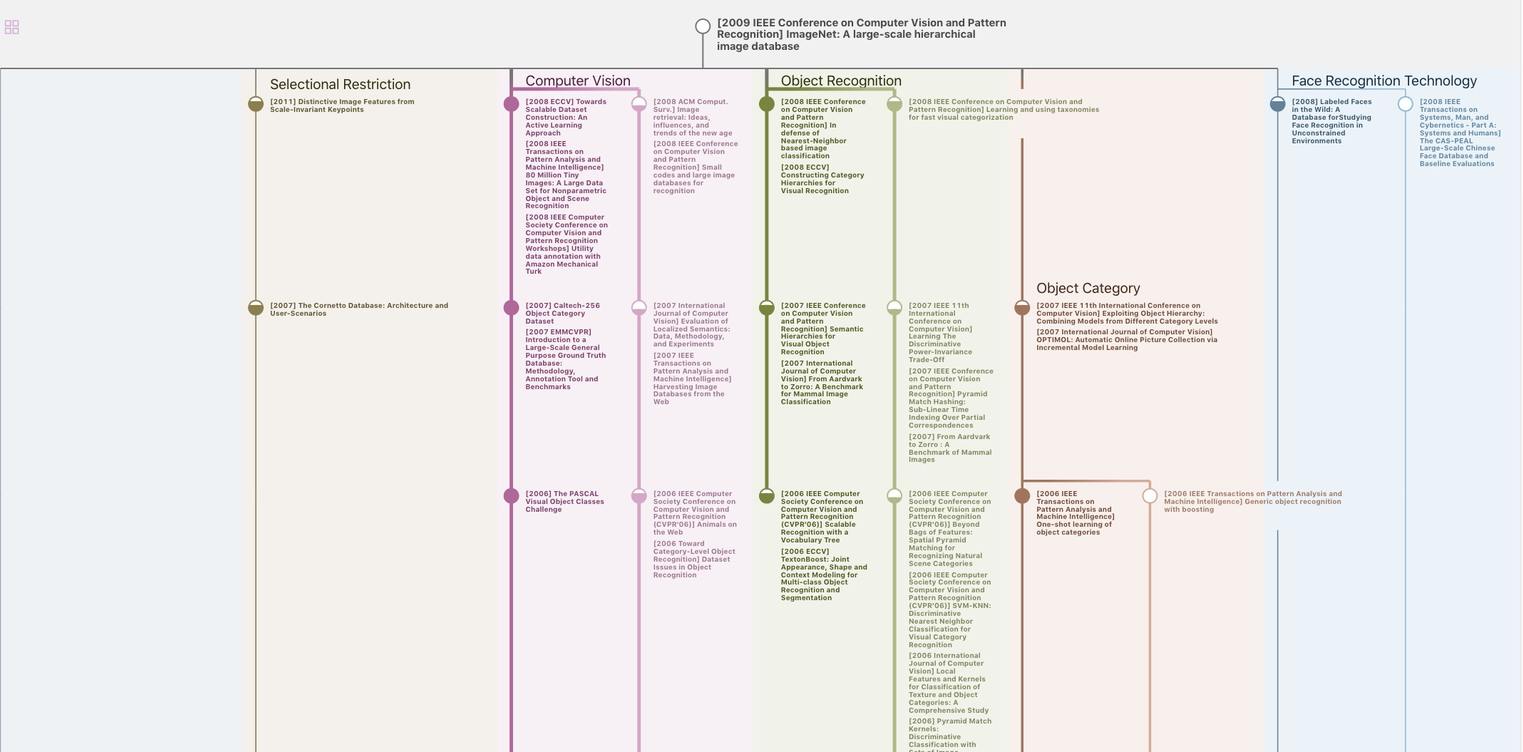
生成溯源树,研究论文发展脉络
Chat Paper
正在生成论文摘要