Modeling graph-structured contexts for image captioning
Image and Vision Computing(2023)
摘要
The performance of image captioning has been significantly improved recently through deep neural network architectures combining with attention mechanisms and reinforcement learning optimization. Exploring visual relationships and interactions between different objects appearing in the image, however, is far from being investigated. In this paper, we present a novel approach that combines scene graphs with Transformer, which we call SGT, to explicitly encode available visual relationships between detected objects. Specifically, we pretrain an scene graph generation model to predict graph representations for images. After that, for each graph node, a Graph Convolutional Network (GCN) is employed to acquire relationship knowledge by aggregating the information of its local neighbors. As we train the captioning model, we feed the potential relation-aware information into the Transformer to generate descriptive sentence. Experiments on the MSCOCO dataset and the Flickr30k dataset validate the superiority of our SGT model, which can realize state-of-the-art results in terms of all the standard evaluation metrics.
更多查看译文
关键词
Image captioning,Transformer,Scene graph,Reinforcement learning,Attention mechanism
AI 理解论文
溯源树
样例
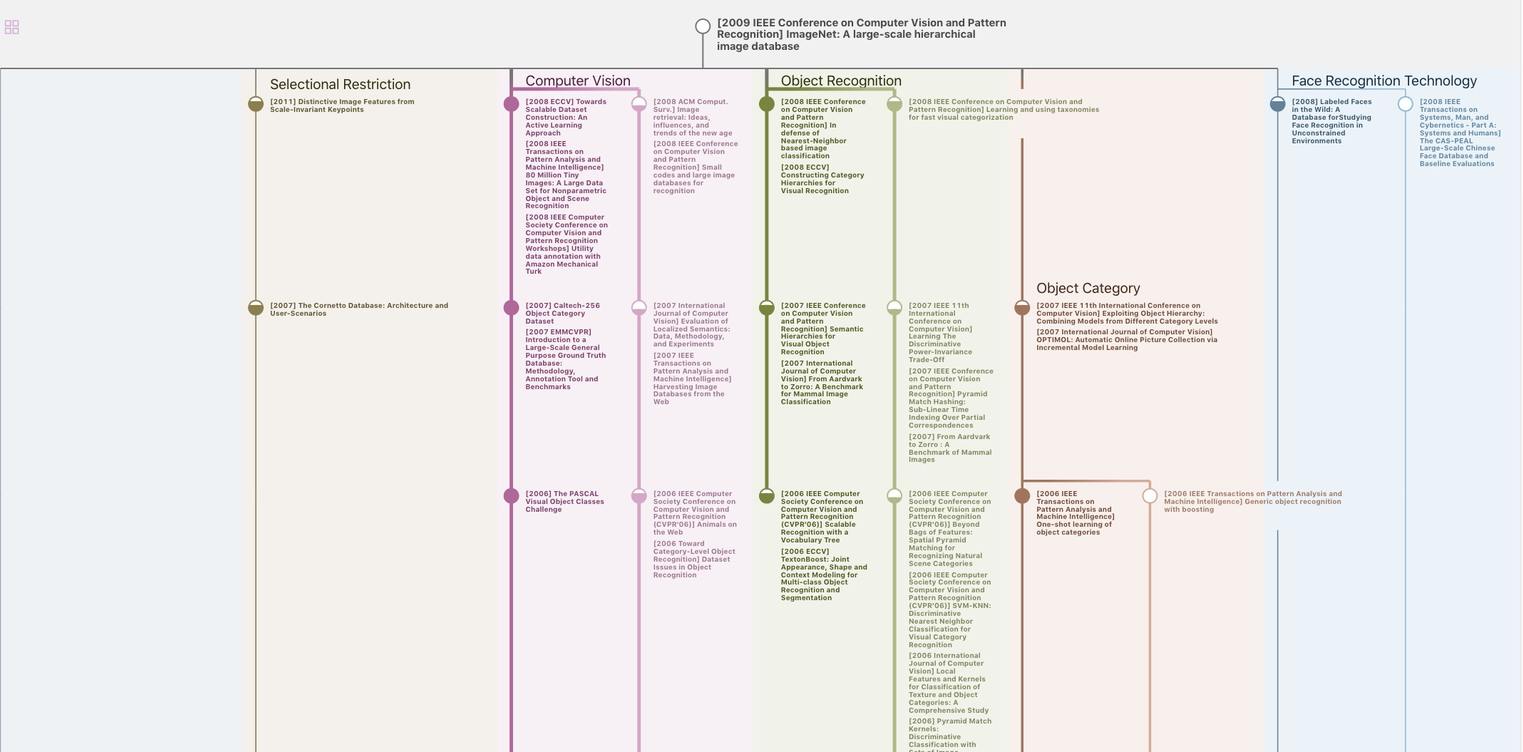
生成溯源树,研究论文发展脉络
Chat Paper
正在生成论文摘要