Forecasting of hygrothermal behaviour of direct solar floors using artificial neural networks
Renewable Energy Focus(2023)
Abstract
Due to the wide range of factors that influence the performance of the direct solar floor (DSF) hygrother-mal behaviour, the dynamic simulation using physical models is a difficult task. The DSF thermal simu-lation are tied to a number of variables that can occasionally be beyond of our control. In this study the hygrothermal behaviour of an experimental room heated by a DSF is predicted using a long short-term memory (LSTM) and a Convolutional Neural Network (CNN) models. First, we studied the DSF system performance in a Mediterranean climate experimentally, and used the results to validate the numerical part. Then, the proposed LSTM and CNN neural network methods are explored to forecast the tempera-ture and humidity of indoor air. The developed model was trained and tested using real experimental data. The predictive accuracy of the proposed models was compared with other models such as the linear switching model (PWARX) and TRNSYS tools, and evaluated using various statistical assesment metrics. The statistical indicators demonstrate that the LSTM outperforms the CNN, PWARX and TRNSYS model's in terms of forecasting accuracy.(c) 2022 Elsevier Ltd. All rights reserved.
MoreTranslated text
Key words
CNN,Direct solar floor,Heat transfer,LSTM,Prediction,Test cell,Validation
AI Read Science
Must-Reading Tree
Example
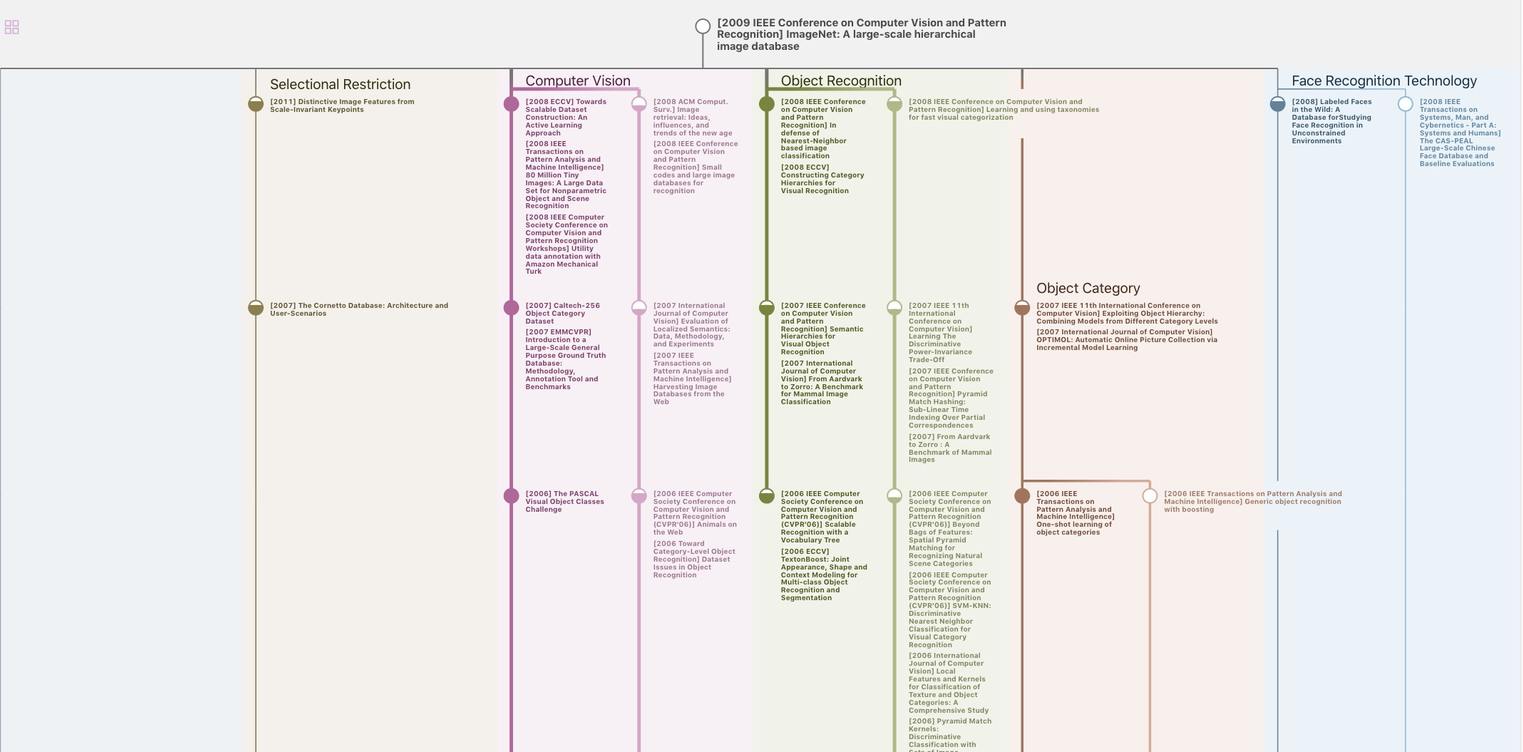
Generate MRT to find the research sequence of this paper
Chat Paper
Summary is being generated by the instructions you defined