Estimating the fundamental period of infilled RC frame structures via deep learning
Urbanism. Arhitectura. Constructii(2023)
Abstract
The fundamental period is deemed an essential dynamic parameter influencing the seismic design of buildings generally, and the RC structures specifically. In this regard, a number of machine learning models have been suggested for its estimation. However, no deep learning method was employed before, despite its wide success in other fields. The present study aims at generating an alternative model for its computation via the Deep-Neural-Network method, to overcome such limitation. Therefore, a database of 4026 cases, gathered from the literature, was developed in the modeling stage. Furthermore, five relevant inputs were chosen following the literature suggestions. Then, the significant inputs were modeled using DNN; their effectiveness was evaluated using three performance indicators plus the K-fold cross-validation method. The findings demonstrated the supremacy of the (5-9-10-1) model, trained by the Tansig transfer function. This proposed model offered the best estimation, i.e., the closest to the period target values in comparison with other models and equations suggested in the literature. Finally, a reliable and easy-to-use Android app named “Building Period Estimator” was designed and uploaded on Google play store. This latter will greatly assist civil engineers and researchers when approximating the period more efficiently, plus saving both time and money.
MoreTranslated text
Key words
infilled rc frame structures,deep learning,fundamental period
AI Read Science
Must-Reading Tree
Example
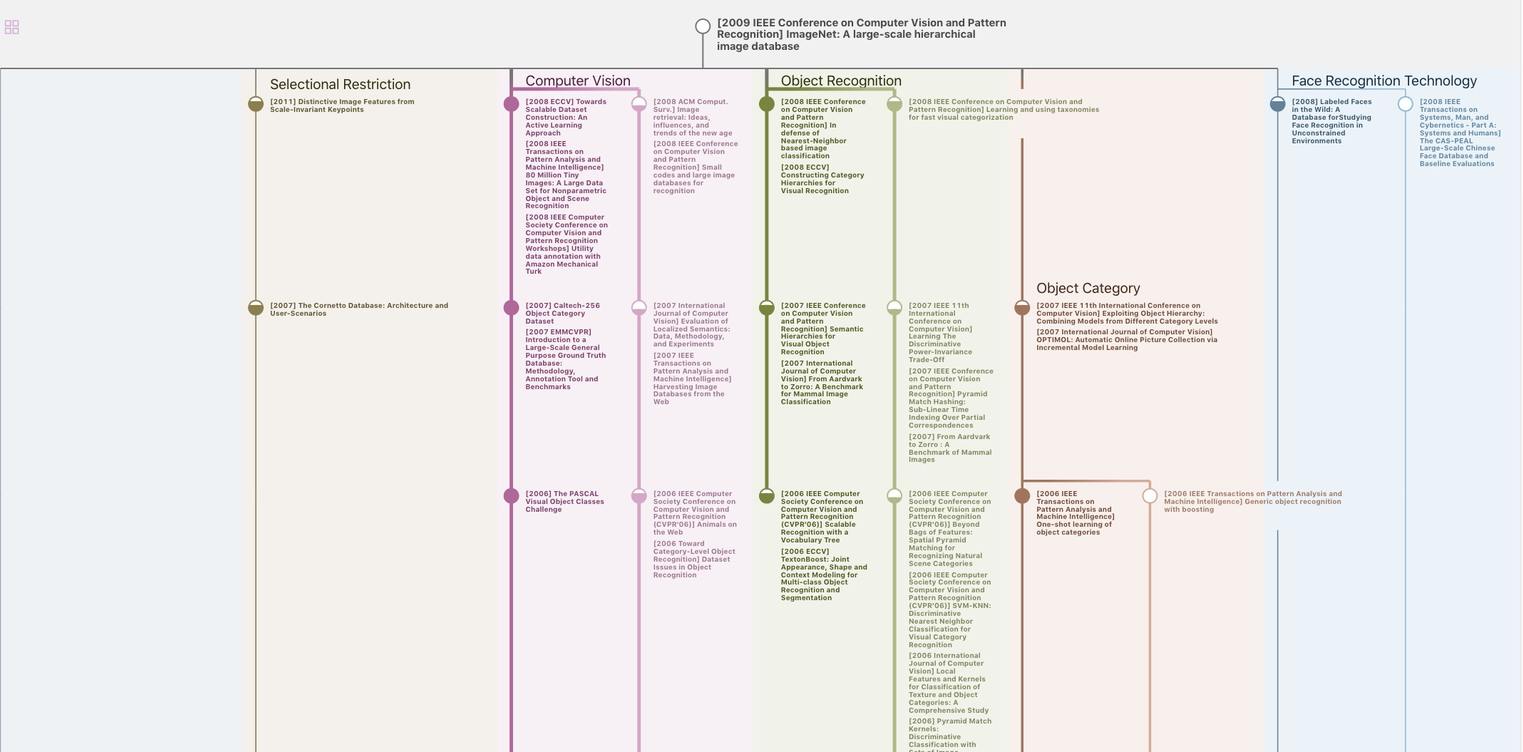
Generate MRT to find the research sequence of this paper
Chat Paper
Summary is being generated by the instructions you defined