A Hybrid Matching Network for Fault Diagnosis under Different Working Conditions with Limited Data
Computational Intelligence and Neuroscience(2022)
摘要
Intelligent fault diagnosis methods based on deep learning have achieved much progress in recent years. However, there are two major factors causing serious degradation of the performance of these algorithms in real industrial applications, i.e., limited labeled training data and complex working conditions. To solve these problems, this study proposed a domain generalization-based hybrid matching network utilizing a matching network to diagnose the faults using features encoded by an autoencoder. The main idea was to regularize the feature extractor of the network with an autoencoder in order to reduce the risk of overfitting with limited training samples. In addition, a training strategy using dropout with random changing rates on inputs was implemented to enhance the model’s generalization on unseen domains. The proposed method was validated on two different datasets containing artificial and real faults. The results showed that considerable performance was achieved by the proposed method under cross-domain tasks with limited training samples.
更多查看译文
AI 理解论文
溯源树
样例
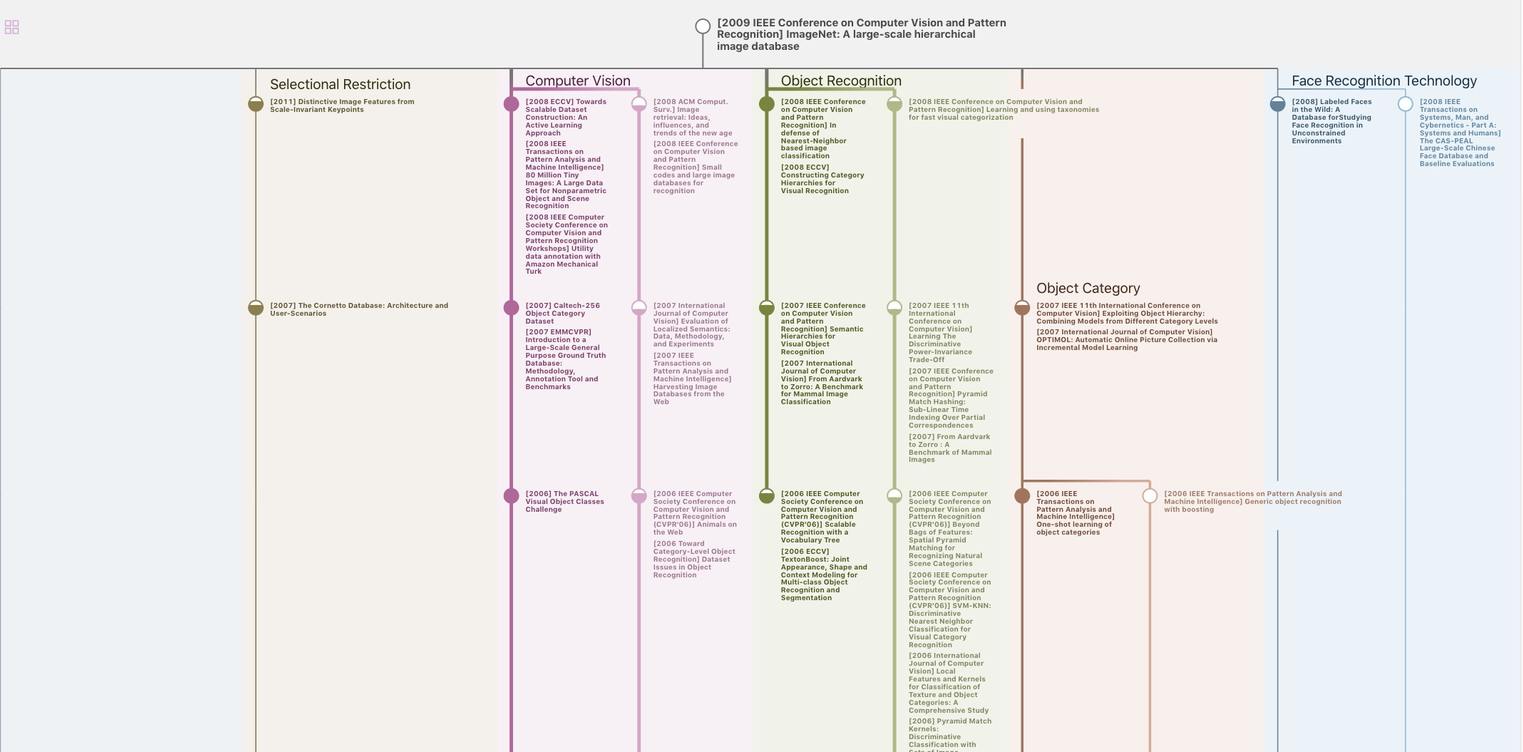
生成溯源树,研究论文发展脉络
Chat Paper
正在生成论文摘要