Finding Meanings in Low Dimensional Structures: Stochastic Neighbor Embedding Applied to the Analysis of Indri indri Vocal Repertoire
Animals(2019)
摘要
Although there is a growing number of researches focusing on acoustic communication, the lack of shared analytic approaches leads to inconsistency among studies. Here, we introduced a computational method used to examine 3360 calls recorded from wild indris (Indri indri) from 2005–2018. We split each sound into ten portions of equal length and, from each portion we extracted spectral coefficients, considering frequency values up to 15,000 Hz. We submitted the set of acoustic features first to a t-distributed stochastic neighbor embedding algorithm, then to a hard-clustering procedure using a k-means algorithm. The t-distributed stochastic neighbor embedding (t-SNE) mapping indicated the presence of eight different groups, consistent with the acoustic structure of the a priori identification of calls, while the cluster analysis revealed that an overlay between distinct call types might exist. Our results indicated that the t-distributed stochastic neighbor embedding (t-SNE), successfully been employed in several studies, showed a good performance also in the analysis of indris’ repertoire and may open new perspectives towards the achievement of shared methodical techniques for the comparison of animal vocal repertoires.
更多查看译文
关键词
lemurs,vocal communication,unsupervised analyses
AI 理解论文
溯源树
样例
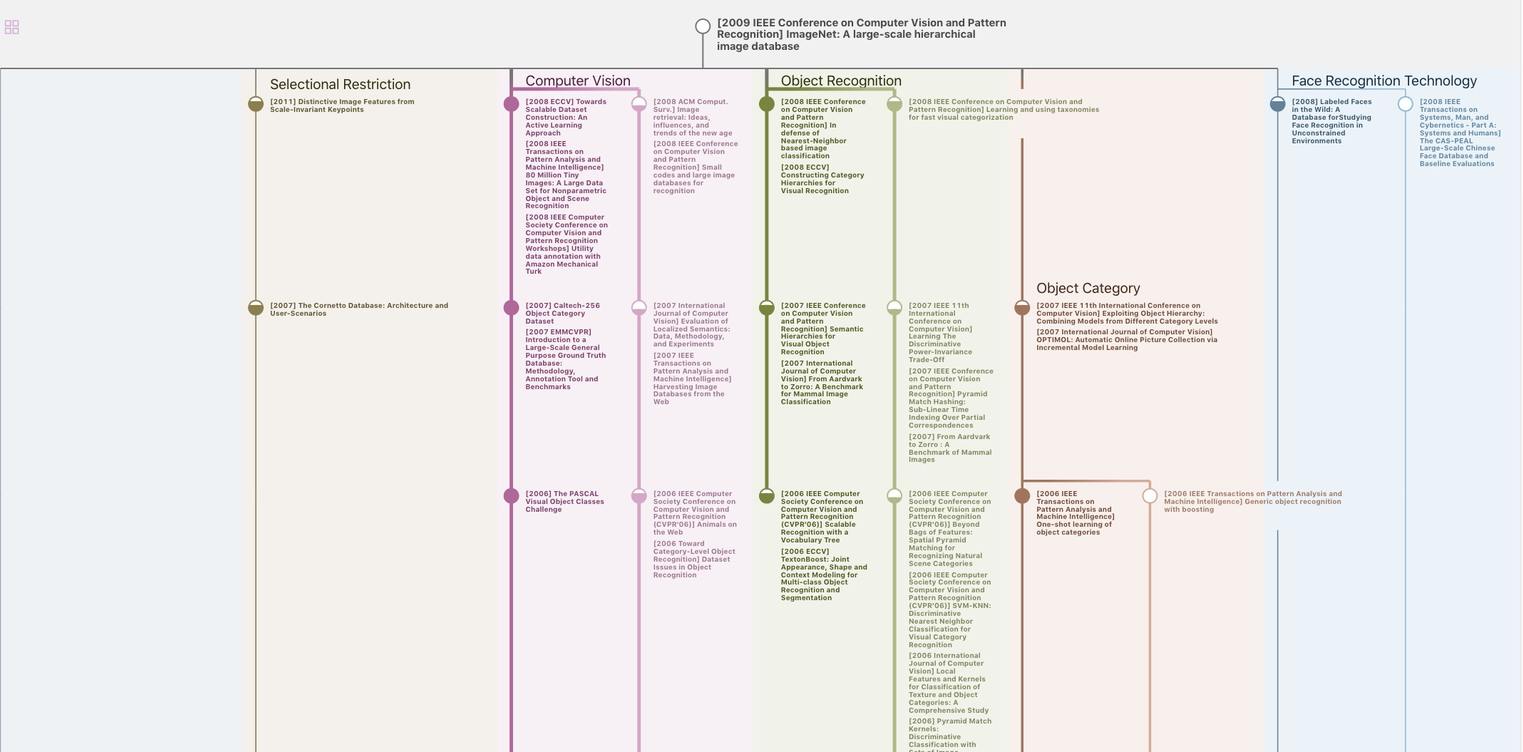
生成溯源树,研究论文发展脉络
Chat Paper
正在生成论文摘要