A Sample-Rebalanced Outlier-Rejected $k$ -Nearest Neighbor Regression Model for Short-Term Traffic Flow Forecasting
IEEE Access(2020)
摘要
Short-term traffic flow forecasting is a fundamental and challenging task due to the stochastic dynamics of the traffic flow, which is often imbalanced and noisy. This paper presents a sample-rebalanced and outlier-rejected k-nearest neighbor regression model for short-term traffic flow forecasting. In this model, we adopt a new metric for the evolutionary traffic flow patterns, and reconstruct balanced training sets by relative transformation to tackle the imbalance issue. Then, we design a hybrid model that considers both local and global information to address the limited size of the training samples. We employ four real-world benchmark datasets often used in such tasks to evaluate our model. Experimental results show that our model outperforms state-of-the-art parametric and non-parametric models.
更多查看译文
关键词
Intelligent transportation systems,road transportation,time series analysis,stochastic processes
AI 理解论文
溯源树
样例
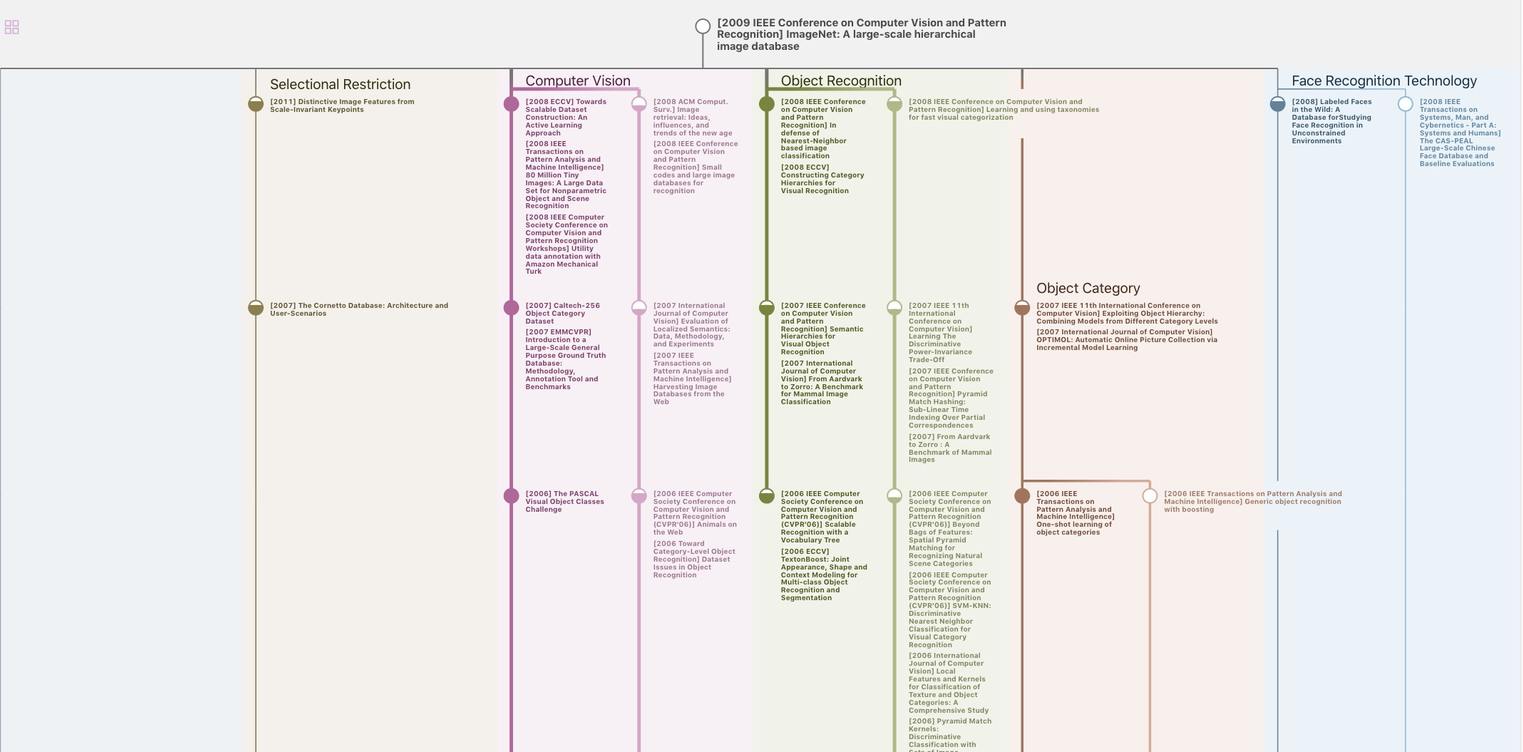
生成溯源树,研究论文发展脉络
Chat Paper
正在生成论文摘要