Exploiting a knowledge base for intelligent decision tree construction to enhance classification power
Engineering and Applied Science Research(2022)
摘要
Decision Trees are a common approach used for classifying unseen data into defined classes. The Information Gain is usually applied as splitting criteria in the node selection process for constructing the decision tree. However, bias in selecting the multi-variation attributes is a major limitation of using this splitting condition, leading to unsatisfactory classification performance. To deal with this problem, a new decision tree algorithm called “Knowledge-Based Decision Tree (KDT)” is proposed which exploits the knowledge in an ontology to assist the decision tree construction. The novelty of the study is that an ontology is applied to determine the attribute importance values using the PageRank algorithm. These values are used to modify the Information Gain to obtain appropriate attributes to be nodes in the decision tree. Four different datasets, Soybean, Heart disease, Dengue fever, and COVID-19 dataset, were employed to evaluate the proposed approach. The experimental results show that the proposed method is superior to the other decision tree algorithms, such as the traditional ID3 and the Mutual Information Decision tree (MIDT), and also performs better than a non-decision tree algorithm, e.g., the k-Nearest Neighbors.
更多查看译文
关键词
classification,id3,information gain,knowledge-base,semantic
AI 理解论文
溯源树
样例
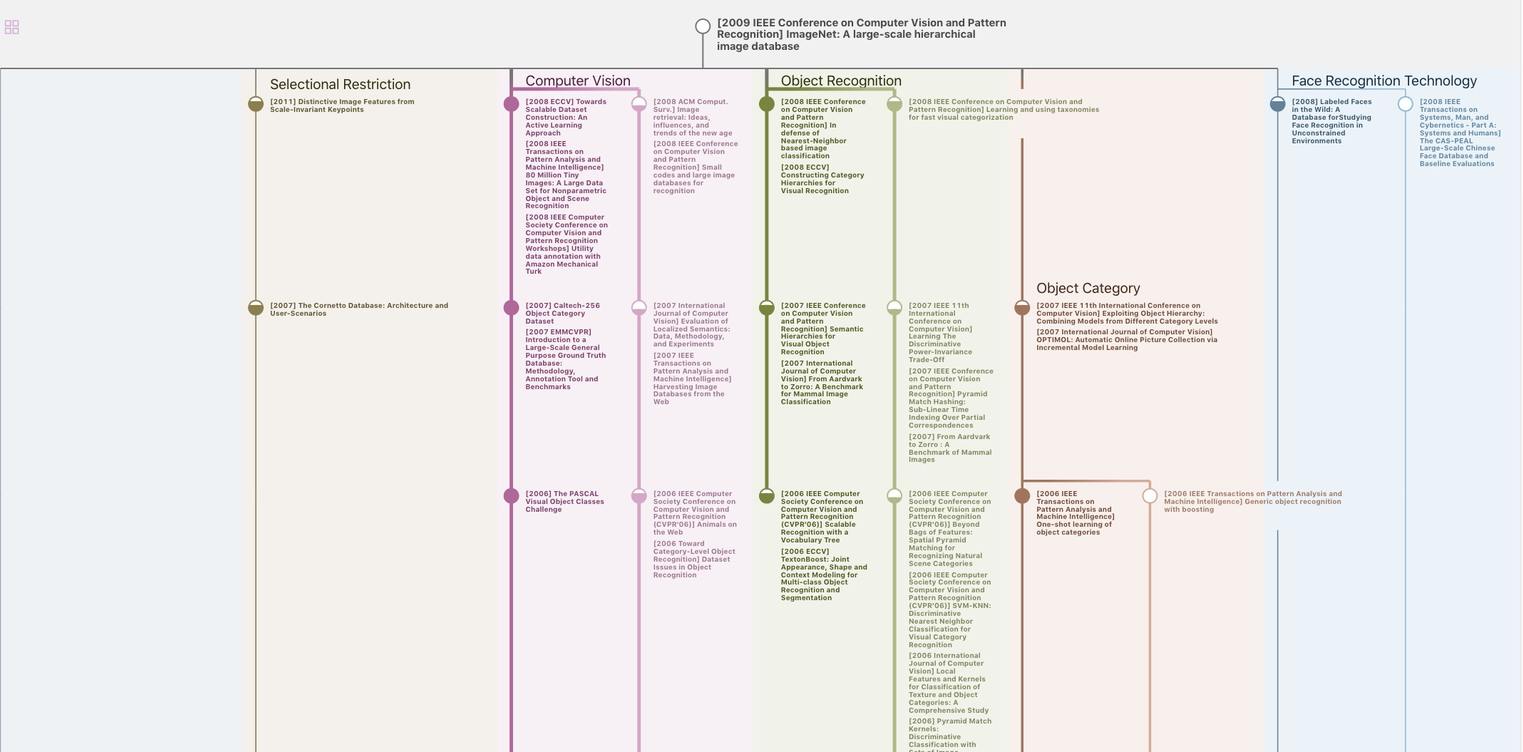
生成溯源树,研究论文发展脉络
Chat Paper
正在生成论文摘要