Robot Learning for Autonomous Assembly
semanticscholar(2018)
摘要
We investigate autonomous assembly from a random initiation on Human Support Robot (HSR), with the aim of solving the Siemens Assembly Challenge. We formulate it as a Reinforcement Learning (RL) problem with sparse reward. A new learning paradigm, Scheduled Auxiliary Control (SAC), excels at sparse reward RL and appears to be a great fit to our problem. The key idea behind SAC is the high-level scheduling of auxiliary tasks and the execution of auxiliary policies to explore efficiently. We experiment in ROS-based Gazebo simulated environments built on top of the OpenAI Gym, with the potential of being extended to learn the same tasks on a real robot from scratch.
更多查看译文
AI 理解论文
溯源树
样例
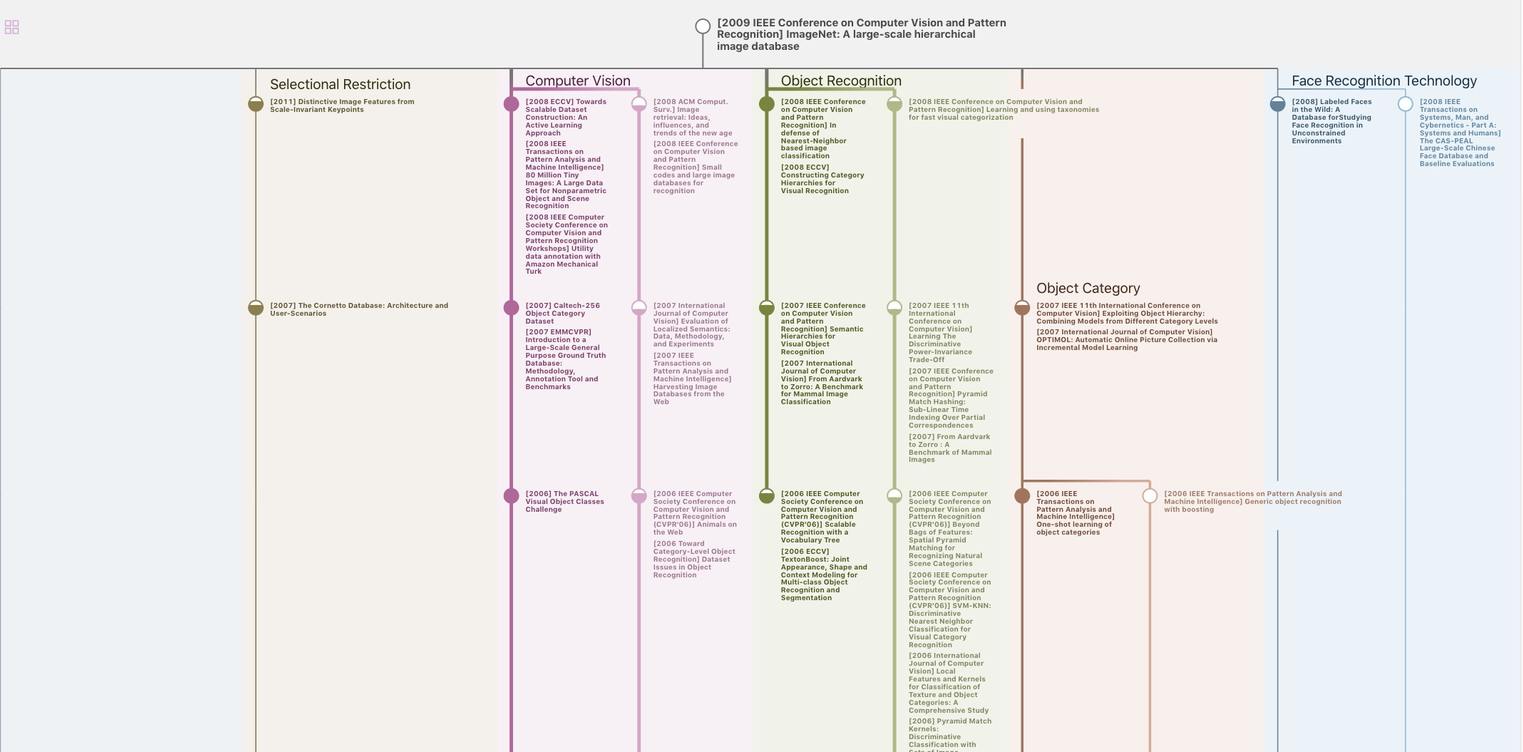
生成溯源树,研究论文发展脉络
Chat Paper
正在生成论文摘要