Forest landscape restoration: Spectral behavior and diversity of tropical tree cover classes
Remote Sensing Applications: Society and Environment(2023)
Abstract
Forest landscape restoration (FLR) commitments have been established in the past years to restore over 200 million hectares, as part of the global Bonn Challenge goal, mostly through the implementation of several different restorative practices in degraded lands, ranging from com-mercial tree monocultures to restoration plantings. The potential of such contrasting restorative practices to support biodiversity conservation and ecosystem services provision vary over space and time, making the monitoring of FLR programs an emerging challenge. Remote sensing techniques, together with innovative technologies for data acquisition, treatment, and analysis have proven to be strategic for planning and monitoring FLR, yet there are still important un-resolved questions. Here, we evaluated the potential of multispectral orbital images of the high spatial (5 m) and spectral (12 bands) resolution VEN mu S microsatellite, joint project of the Israeli Space Agency and CNES, to classify the spectral behavior and diversity of six tree cover classes (savanna woodlands, old-and second-growth semi-deciduous forests, young restoration plant-ings, and eucalyptus and pine tree monocultures) commonly found in FLR programs in tropical regions. We assessed how these tree cover classes located in a study landscape in southeastern Brazil differ according to their spectral response (winter and summer bands, and vegetation indices), canopy variability (textural features), seasonal behavior (delta layers -difference be-tween summer and winter vegetation indexes), and spectral diversity, and used these attributes as variables to the model. We used the Random Forest algorithm to generate the models and evaluate how the tree cover classes differ in the classification and how the variables supported the model. We achieved high values of global accuracy (91.9%) and "F1 score" (above 0.8) for all tree cover classes, in which second-growth forest presented the lowest accuracy. The textural layers, delta layers, and the spectral diversity layers were the most important attributes to discriminate among tree cover classes. We demonstrate here the potential of using VEN mu S or similar sensor images together with different image processing and machine learning algorithms to monitor FLR programs, allowing further remote sensing approaches and in-deep field assessments to advance evaluation of FLR benefits for nature and people. We demonstrated how the fusion of all these types of data and innovative approaches to data processing, can result in novel ways to assess restoration performance and open new avenues to upscale monitoring, bridging the gap between FLR expectations and achieved goals.
MoreTranslated text
Key words
Remote sensing,Forest restoration,Tropical forest,Forest classification,Multispectral satellite images,High resolution,Random forest
AI Read Science
Must-Reading Tree
Example
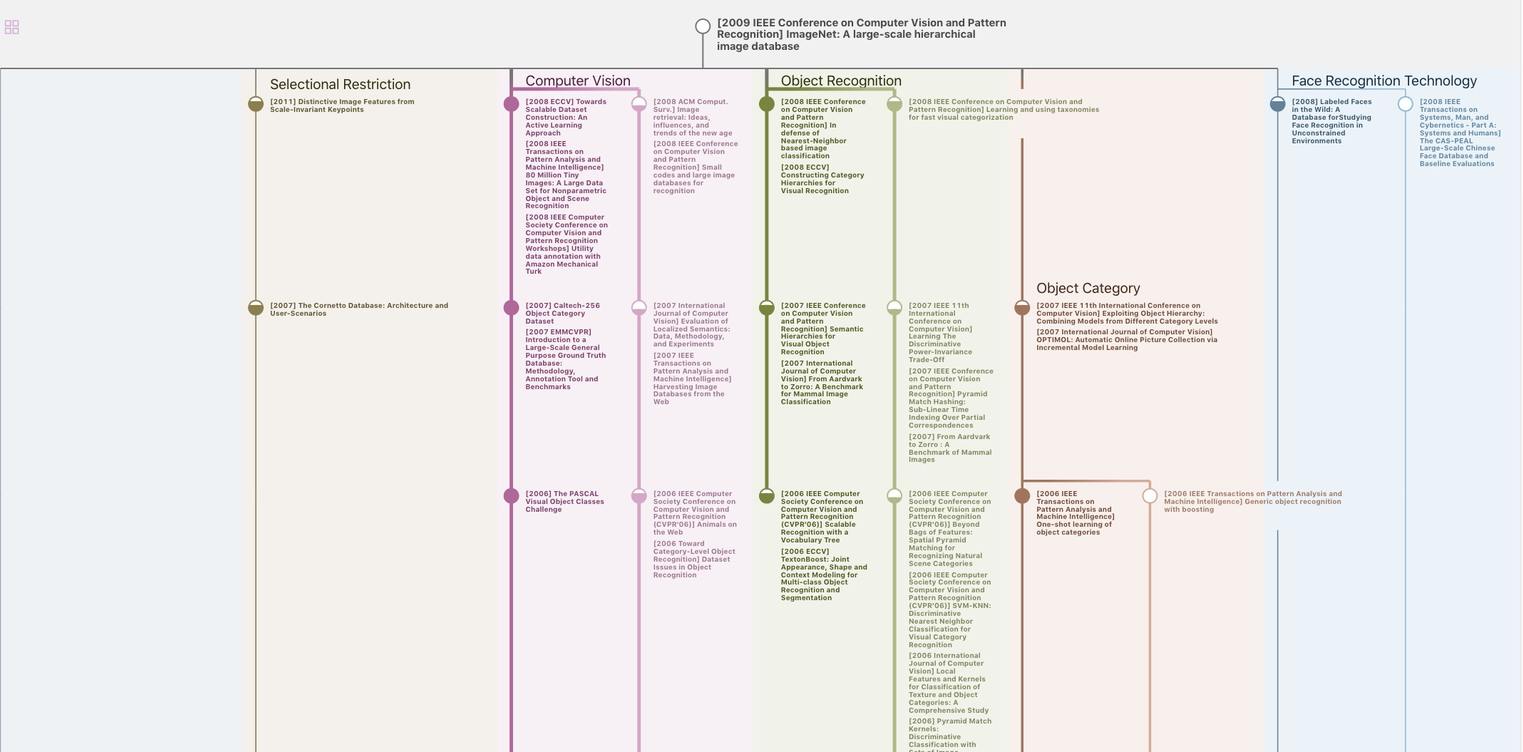
Generate MRT to find the research sequence of this paper
Chat Paper
Summary is being generated by the instructions you defined