Integrative modeling of heterogeneous soil salinity using sparse ground samples and remote sensing images
Geoderma(2023)
Abstract
Soil salinization is a major environmental risk caused by natural or human activities especially in arid and semi -arid regions. Machine learning for rapidly monitoring large-scale spatial soil salinization becomes possible. However, machine learning often needs large training samples and obtaining extensive soil salinization infor-mation by field investigation is laborious and difficult. In practice, the field soil sampling datasets are often sparse and non-normally distributed. The intricacy of features extracted from remote sensing images increases the model complexity and often leads to degradation in the prediction performance. To solve this problem, an integrative framework is proposed to predict soil salt content (SSC) based on light gradient boosting machine (LGBM). In this model, we first introduce the data augmentation method (Mixup) to improve sample diversity and alleviate model overfitting by the sparsity of samples. To improve the generalization and robustness of the model in different spatial heterogeneity of soil salinization, the Mixup-LGBM model is adaptively and jointly optimized by combining hyperparameters and feature selection in a Bayesian optimization framework. Furthermore, model interpretability is improved using shapley additive explanations (SHAP) value based on the combination of the confidence of the synthetic data through model visualization and feature importance assessment. In addition, different cases are simulated to test the model performance. In Case I, the raw sample-sparsity model using the data augmentation algorithm has higher prediction accuracy than other unused models. In Case II, the extreme sample-sparsity model still achieves satisfactory results while the other models can't learn any effective information after multiple iterations. The experimental results reveal that the proposed model can automatically find representative features in heterogeneous environments and has strong adaptability in different study areas. This finding indicates that digital elevation model (DEM) has a high influence on SSC in both study areas. Besides the DEM, soil salinization in the Manasi River Basin is more sensitive to human ac-tivities, while that in the Werigan-Kuqa River Delta Oasis is more sensitive to natural factors. The Mixup-LGBM model is suitable for predicting SSC in different sample sparsity scenarios while ensuring the high accuracy. The model has considerable potential for dealing with other complex sample sparsity regression tasks.
MoreTranslated text
Key words
Soil salinity,Sparse samples,Mixup,Light gradient boosting machine (LightGBM),Bayesian optimization,Shapley additive explanations (SHAP)
AI Read Science
Must-Reading Tree
Example
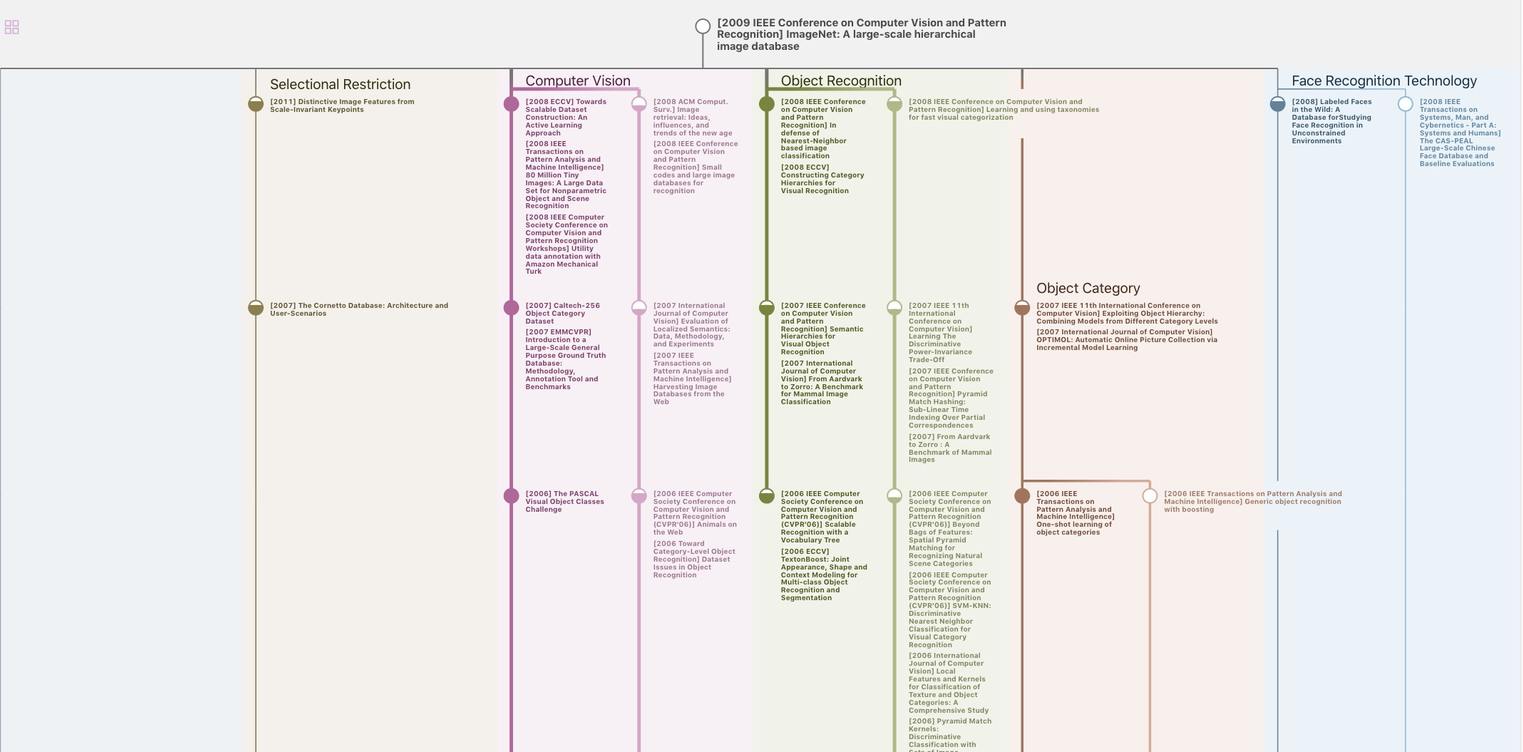
Generate MRT to find the research sequence of this paper
Chat Paper
Summary is being generated by the instructions you defined