Residual strength prediction of corroded pipelines using multilayer perceptron and modified feedforward neural network
RELIABILITY ENGINEERING & SYSTEM SAFETY(2023)
Abstract
Corrosion defects occurring in natural gas pipelines are common and annoying. The residual strength prediction of corroded pipelines is usually carried out based on theoretical, numerical, and experimental methods. However, the results are hard to obtain when it comes to high nonlinear problems. In this paper, an artificial neural network (ANN) was used to predicting residual strength of corroded pipelines. Due to inadequate training data from previous experiments and extreme iterations which were needed to ensure precision of predicting results, the overfitting phenomenon occurred. To solve the overfitting phenomenon, the training accuracy was reduced artificially by using ReLU activation function and dropout method which was cutting down neurons during ANN training. The results showed that the multilayer perceptron (MLP) conducted dropout method had the highest precision for inadequate sample data compared with relatively simple feedforward neural network (FFNN) structure and FFNN optimized by particle swarm optimization (PSO).
MoreTranslated text
Key words
Residual strength prediction,Corroded pipelines,ANN,FFNN,PSO,Overfitting solution
AI Read Science
Must-Reading Tree
Example
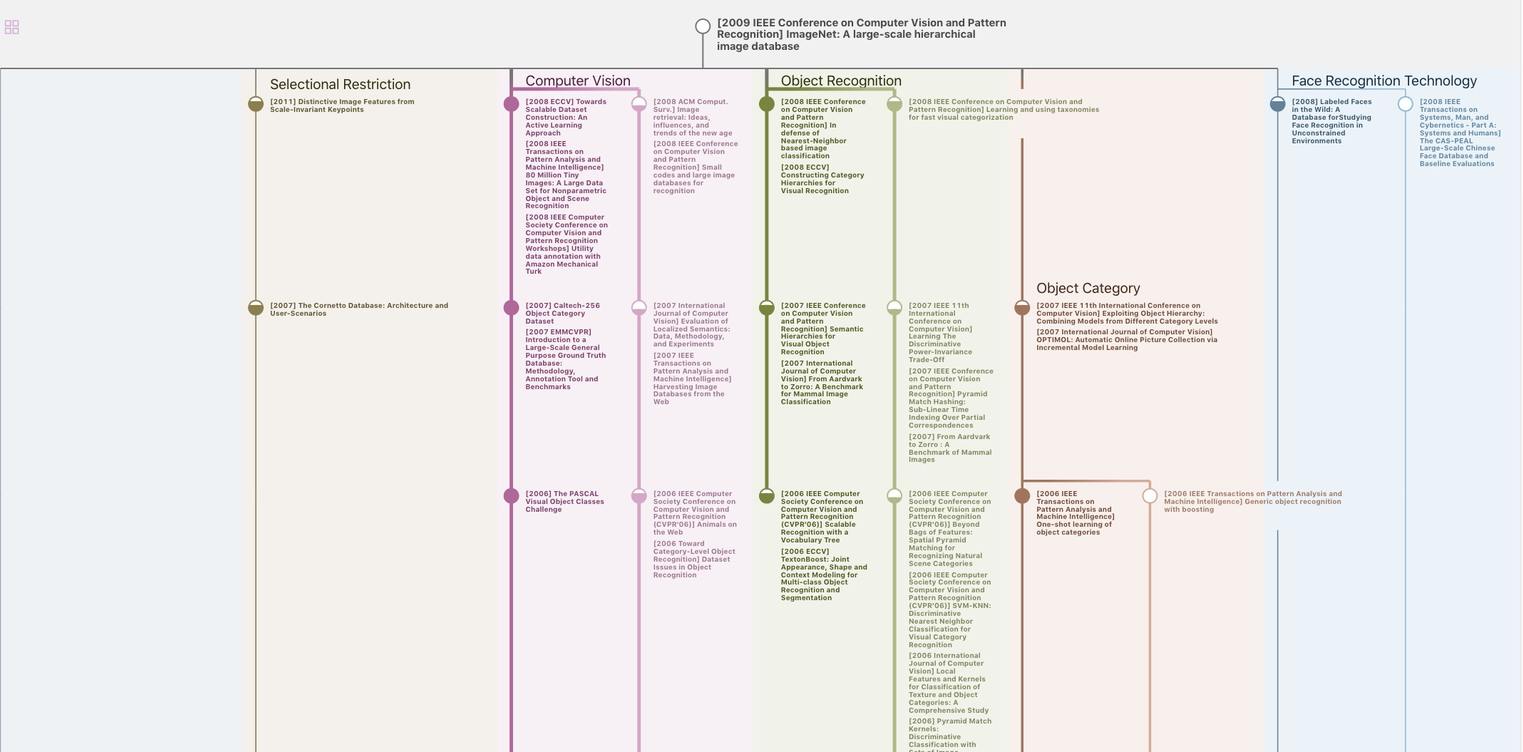
Generate MRT to find the research sequence of this paper
Chat Paper
Summary is being generated by the instructions you defined