Deep Axial Hypercomplex Networks
CoRR(2023)
Abstract
Over the past decade, deep hypercomplex-inspired networks have enhanced feature extraction for image classification by enabling weight sharing across input channels. Recent works make it possible to improve representational capabilities by using hypercomplex-inspired networks which consume high computational costs. This paper reduces this cost by factorizing a quaternion 2D convolutional module into two consecutive vectormap 1D convolutional modules. Also, we use 5D parameterized hypercomplex multiplication based fully connected layers. Incorporating both yields our proposed hypercomplex network, a novel architecture that can be assembled to construct deep axial-hypercomplex networks (DANs) for image classifications. We conduct experiments on CIFAR benchmarks, SVHN, and Tiny ImageNet datasets and achieve better performance with fewer trainable parameters and FLOPS. Our proposed model achieves almost 2% higher performance for CIFAR and SVHN datasets, and more than 3% for the ImageNet-Tiny dataset and takes six times fewer parameters than the real-valued ResNets. Also, it shows state-of-the-art performance on CIFAR benchmarks in hypercomplex space.
MoreTranslated text
AI Read Science
Must-Reading Tree
Example
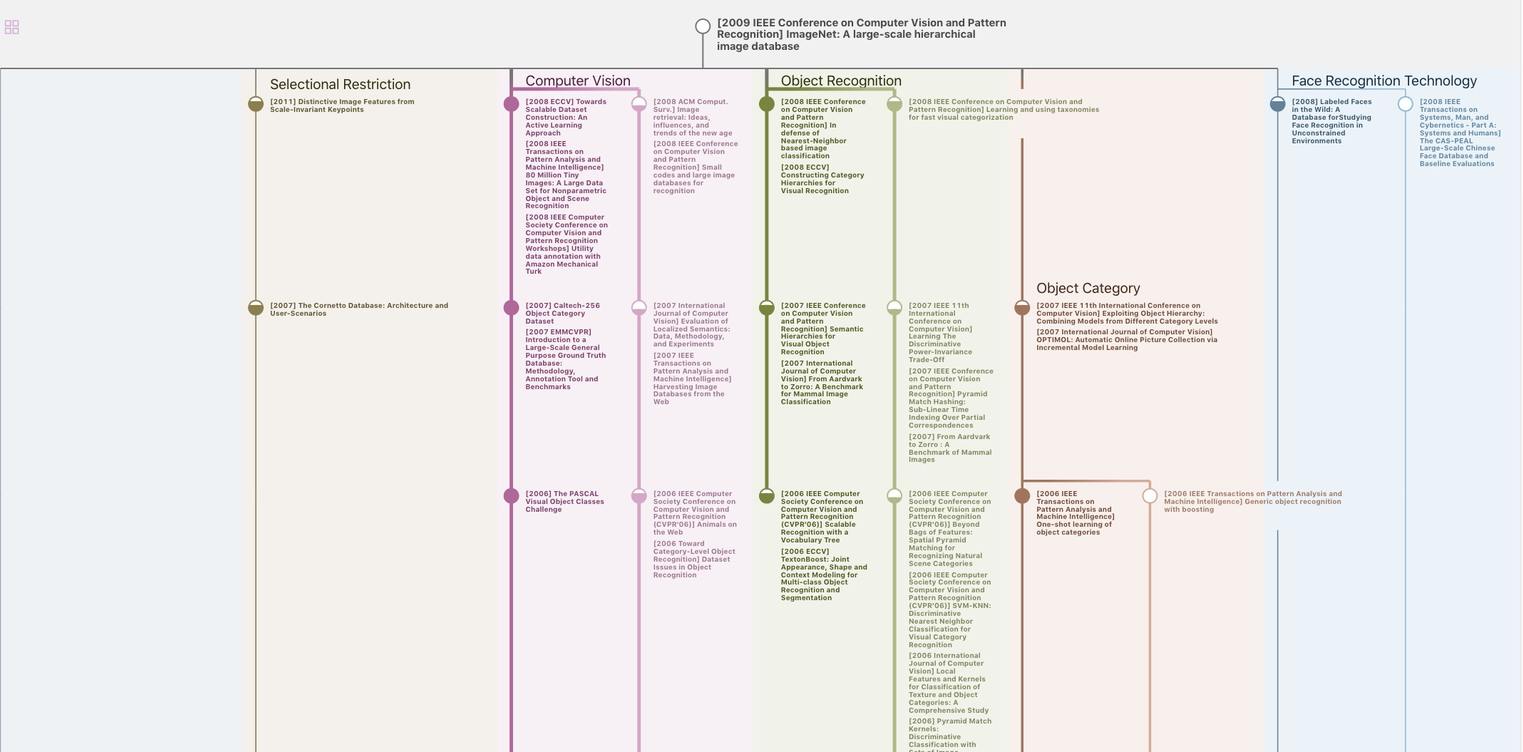
Generate MRT to find the research sequence of this paper
Chat Paper
Summary is being generated by the instructions you defined