Enhancing ResNet Image Classification Performance by using Parameterized Hypercomplex Multiplication
2023 26th International Conference on Computer and Information Technology (ICCIT)(2023)
Abstract
Recently, many deep networks have introduced hypercomplex and related calculations into their architectures. In regard to convolutional networks for classification, these enhancements have been applied to the convolution operations in the frontend to enhance accuracy and/or reduce the parameter requirements while maintaining accuracy. Although these enhancements have been applied to the convolutional frontend, it has not been studied whether adding hypercomplex calculations improves performance when applied to the densely connected backend. This paper studies ResNet architectures and incorporates parameterized hypercomplex multiplication (PHM) into the backend of residual, quaternion, and vectormap convolutional neural networks to assess the effect. We show that PHM does improve classification accuracy performance on several image datasets, including small, low-resolution CIFAR 10/100 and large high-resolution ImageNet and ASL, and can achieve state-of-the-art accuracy for hypercomplex networks.
MoreTranslated text
Key words
QPHM,VPHM,PHM,ResNet,Deep Networks
AI Read Science
Must-Reading Tree
Example
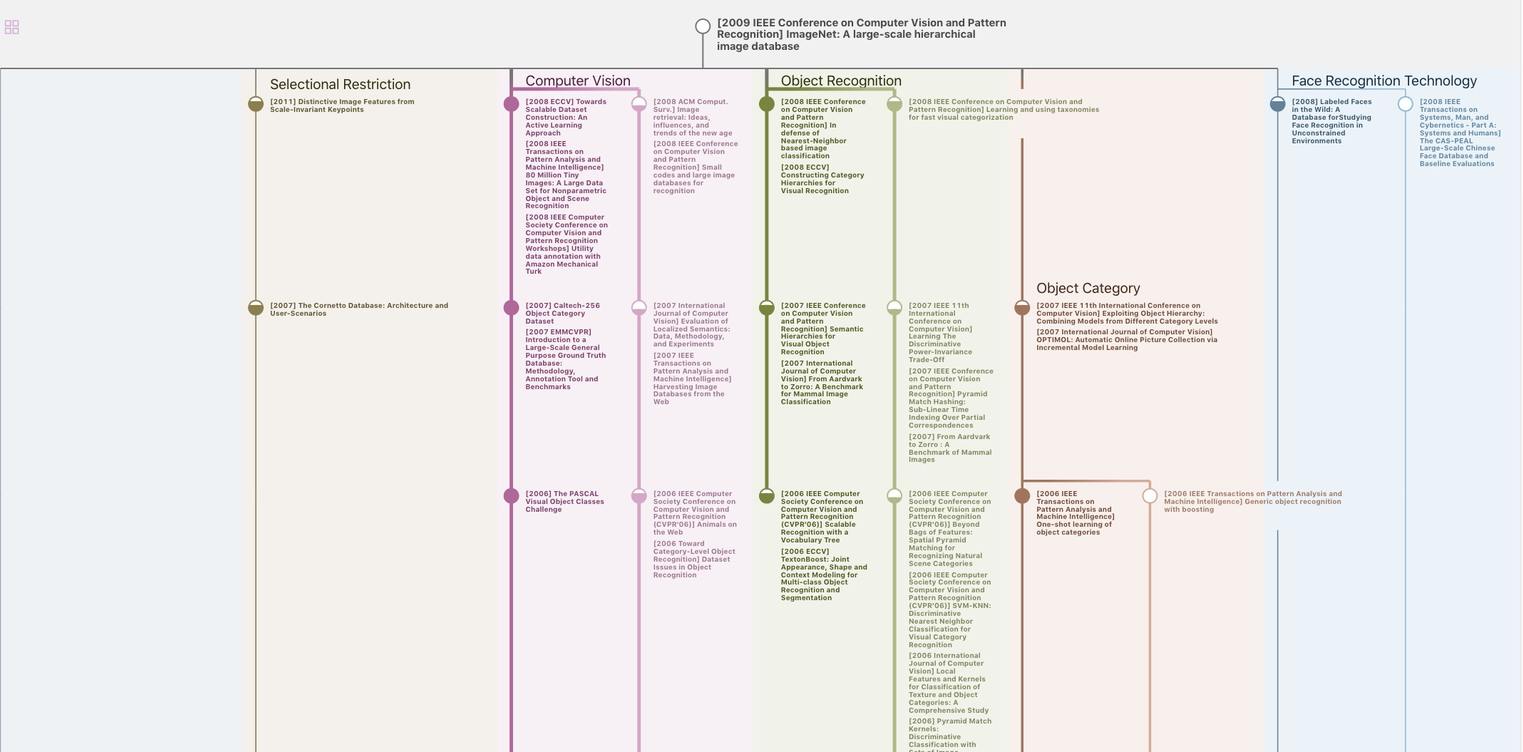
Generate MRT to find the research sequence of this paper
Chat Paper
Summary is being generated by the instructions you defined