Clustering disease trajectories in contrastive feature space for biomarker discovery in age-related macular degeneration
arXiv (Cornell University)(2023)
摘要
Age-related macular degeneration (AMD) is the leading cause of blindness in the elderly. Current grading systems based on imaging biomarkers only coarsely group disease stages into broad categories and are unable to predict future disease progression. It is widely believed that this is due to their focus on a single point in time, disregarding the dynamic nature of the disease. In this work, we present the first method to automatically discover biomarkers that capture temporal dynamics of disease progression. Our method represents patient time series as trajectories in a latent feature space built with contrastive learning. Then, individual trajectories are partitioned into atomic sub-sequences that encode transitions between disease states. These are clustered using a newly introduced distance metric. In quantitative experiments we found our method yields temporal biomarkers that are predictive of conversion to late AMD. Furthermore, these clusters were highly interpretable to ophthalmologists who confirmed that many of the clusters represent dynamics that have previously been linked to the progression of AMD, even though they are currently not included in any clinical grading system.
更多查看译文
关键词
macular degeneration,contrastive feature space,biomarker discovery,clustering,disease trajectories,age-related
AI 理解论文
溯源树
样例
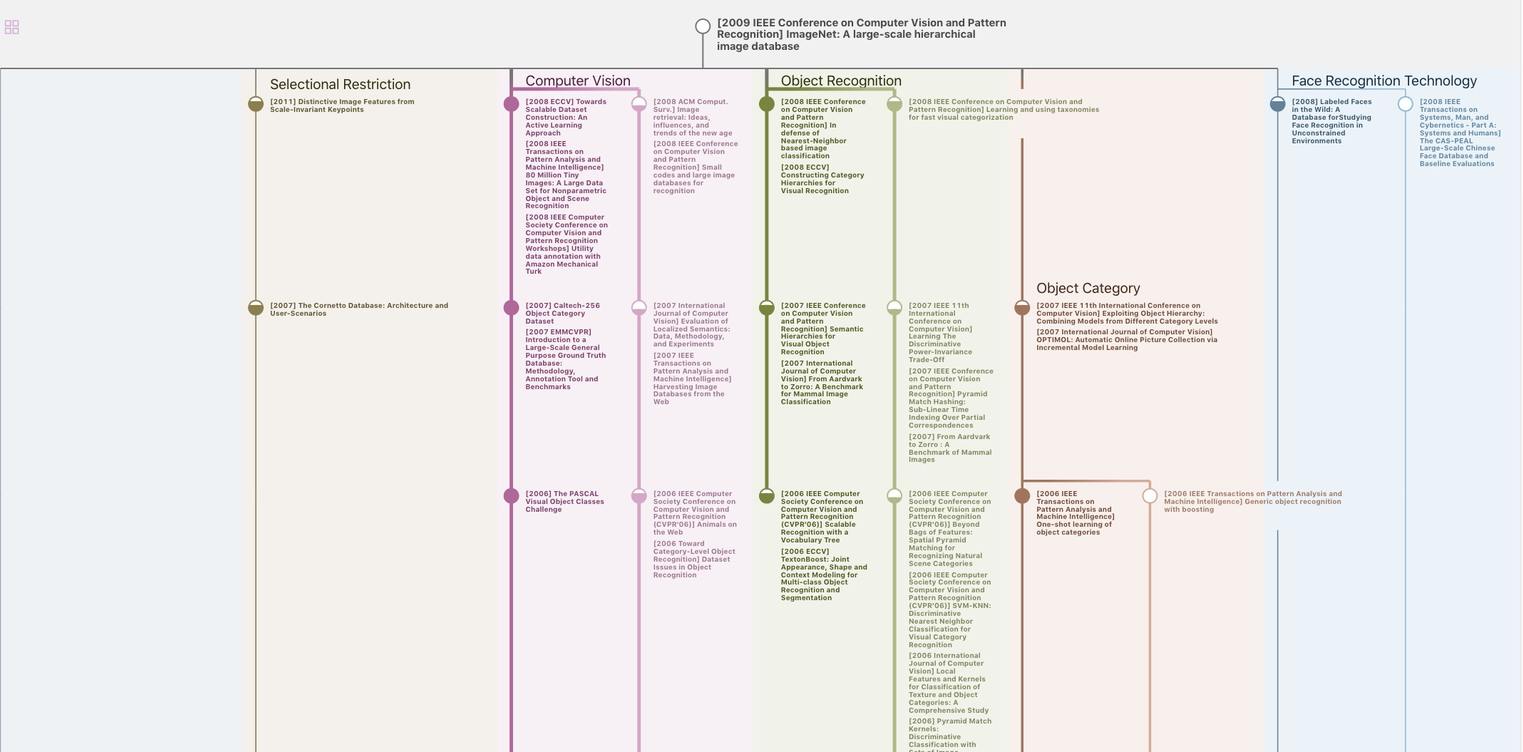
生成溯源树,研究论文发展脉络
Chat Paper
正在生成论文摘要