Combining Self-labeling with Selective Sampling
2023 23RD IEEE INTERNATIONAL CONFERENCE ON DATA MINING WORKSHOPS, ICDMW 2023(2023)
摘要
Access to labeled data is generally expensive, so semi-supervised methods are constantly popular. They enable the acquisition of large datasets without needing too many expert labels. This work combines self-labeling techniques with active learning in a selective sampling scenario by proposing how to build a classifier ensemble. When training the base classifiers, a decision is made on whether to request a new label or use the self-labeling based on evaluating the decision inconsistency of base classifiers. Additionally, a technique inspired by online bagging was used to ensure the ensemble's diversity, whereby individual learning examples are presented to base classifiers at different intensities. The preliminary studies showed that naive application of selflabeling could harm performance by introducing bias towards selected classes and consequently lead to skewed class distribution. Hence, we propose how to reduce this phenomenon. Experimental evaluation confirmed that the proposed method performs well compared to the known selective sampling methods.
更多查看译文
AI 理解论文
溯源树
样例
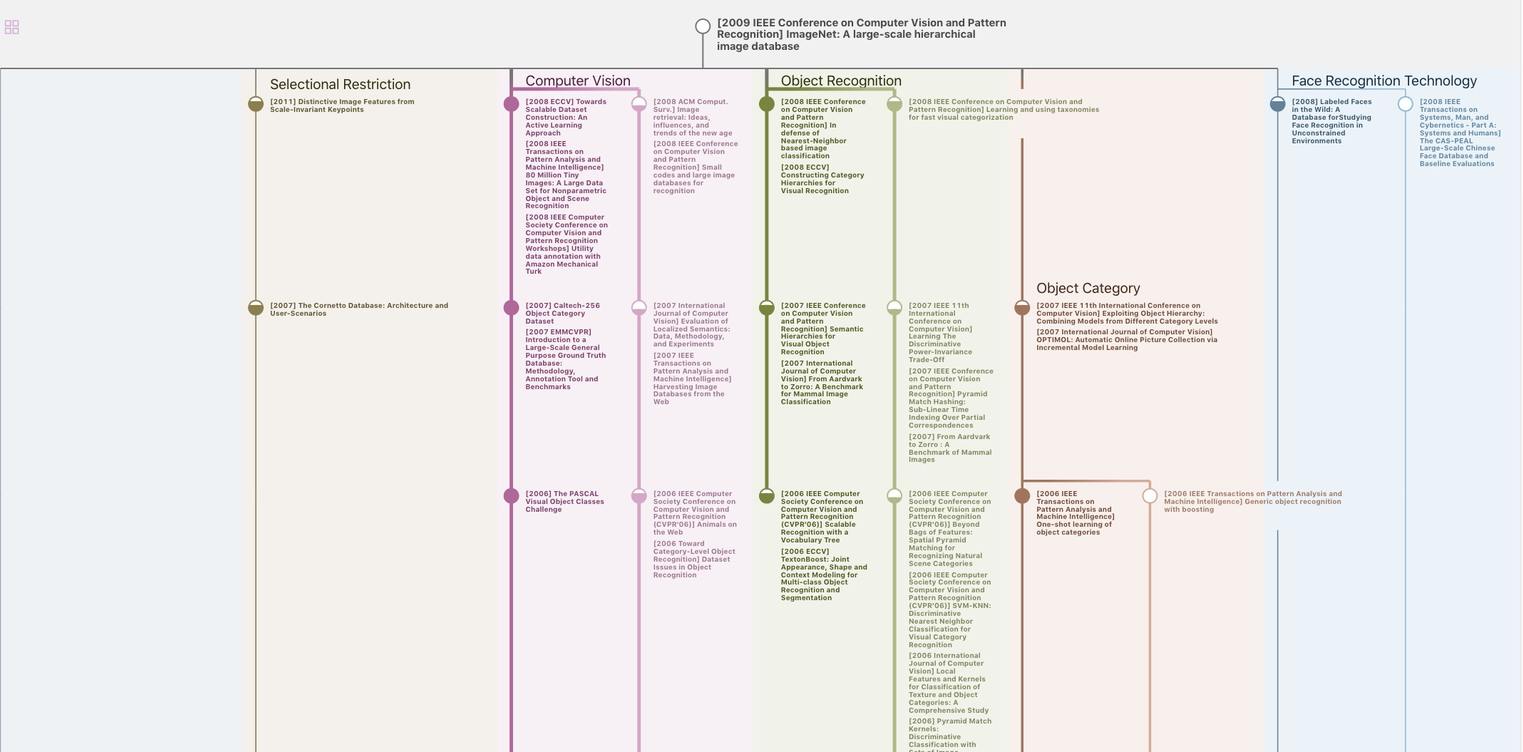
生成溯源树,研究论文发展脉络
Chat Paper
正在生成论文摘要