Part-Aware Framework for Robust Object Tracking
IEEE Transactions on Image Processing(2023)
摘要
The local parts of the target are vitally important for robust object tracking. Nevertheless, existing excellent context regression methods involving siamese networks and discrimination correlation filters mostly represent the target appearance from the holistic model, showing high sensitivity in scenarios with partial occlusion and drastic appearance changes. In this paper, we address this issue by proposing a novel part-aware framework based on context regression, which simultaneously considers the global and local parts of the target and fully exploits their relationship to be collaboratively aware of the target state online. To this end, the spatial-temporal measure among context regressors corresponding to multiple parts is designed to evaluate the tracking quality of each part regressor by solving the imbalance among global and local parts. The coarse target locations provided by part regressors are further aggregated by treating their measures as weights to refine the final target location. Furthermore, the divergence of multiple part regressors in each frame reveals the interference degree of background noise, which is quantified to control the proposed combination window functions in part regressors to adaptively filter redundant noise. Besides, the spatial-temporal information among part regressors is also leveraged to assist in accurately estimating the target scale. Extensive evaluations demonstrate that the proposed framework help many context regression trackers achieve performance improvements and perform favorably against state-of-the-art methods on the popular benchmarks: OTB, TC128, UAV, UAVDT, VOT, TrackingNet, GOT-10k, LaSOT.
更多查看译文
关键词
Object tracking,siamese network,discrimina-tion correlation filter,global and local parts
AI 理解论文
溯源树
样例
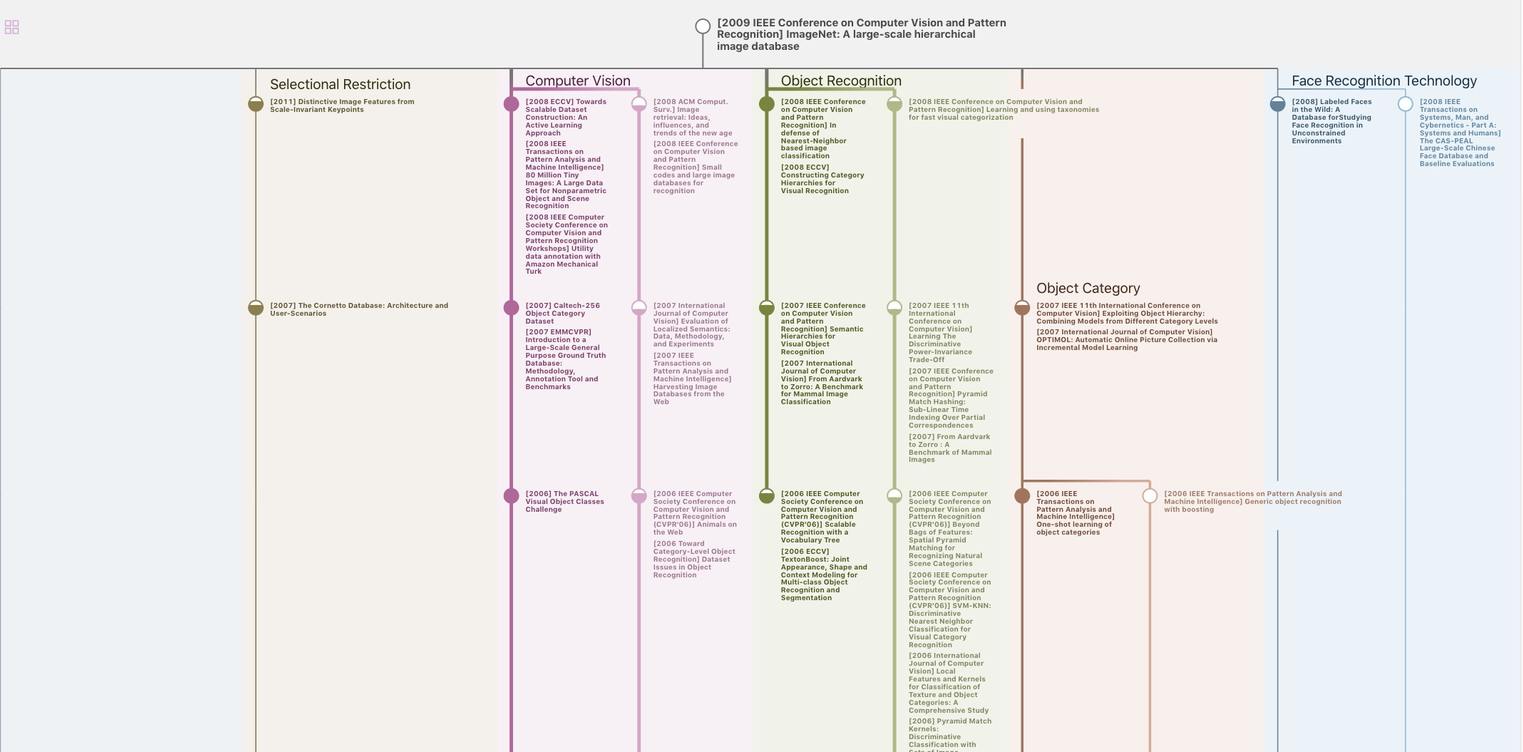
生成溯源树,研究论文发展脉络
Chat Paper
正在生成论文摘要