OCD diagnosis via smooth sparse network and fused sparse auto-encoder learning
Expert Systems with Applications(2023)
摘要
Obsessive-compulsive disorder (OCD) brings many problems to patients. Redundant information in the OCD data can be removed to preserve valuable biological functions through sparse learning methods. Therefore, con-structing a brain functional connectivity network (BFCN) with sparse learning is beneficial to objectively di-agnose OCD. However, most studies ignore the relationship between subjects. Therefore, a new smooth sparse network (SSN) model is proposed to construct BFCN. Specifically, a smoothing term is designed in the objective function to capture the relationship between subjects. Then, a fused sparse auto-encoder (FSAE) model is pro-posed to learn the deep feature and decrease feature dimension of BFCN. The FASE is able to fuse the regularized sparse auto-encoder (RSAE) features and regularized stacking SAE (RSSAE) features for diagnosing OCD. Spe-cifically, the l2-norm regularization is integrated in RSAE and RSSAE to address overfitting. Our proposed method combines the traditional machine learning with deep learning, which can achieve promising OCD diagnosis performance on our self-collected data.
更多查看译文
关键词
Obsessive -compulsive disorder,Brain functional connectivity network,Smooth sparse network,Fused regularized auto -encoders
AI 理解论文
溯源树
样例
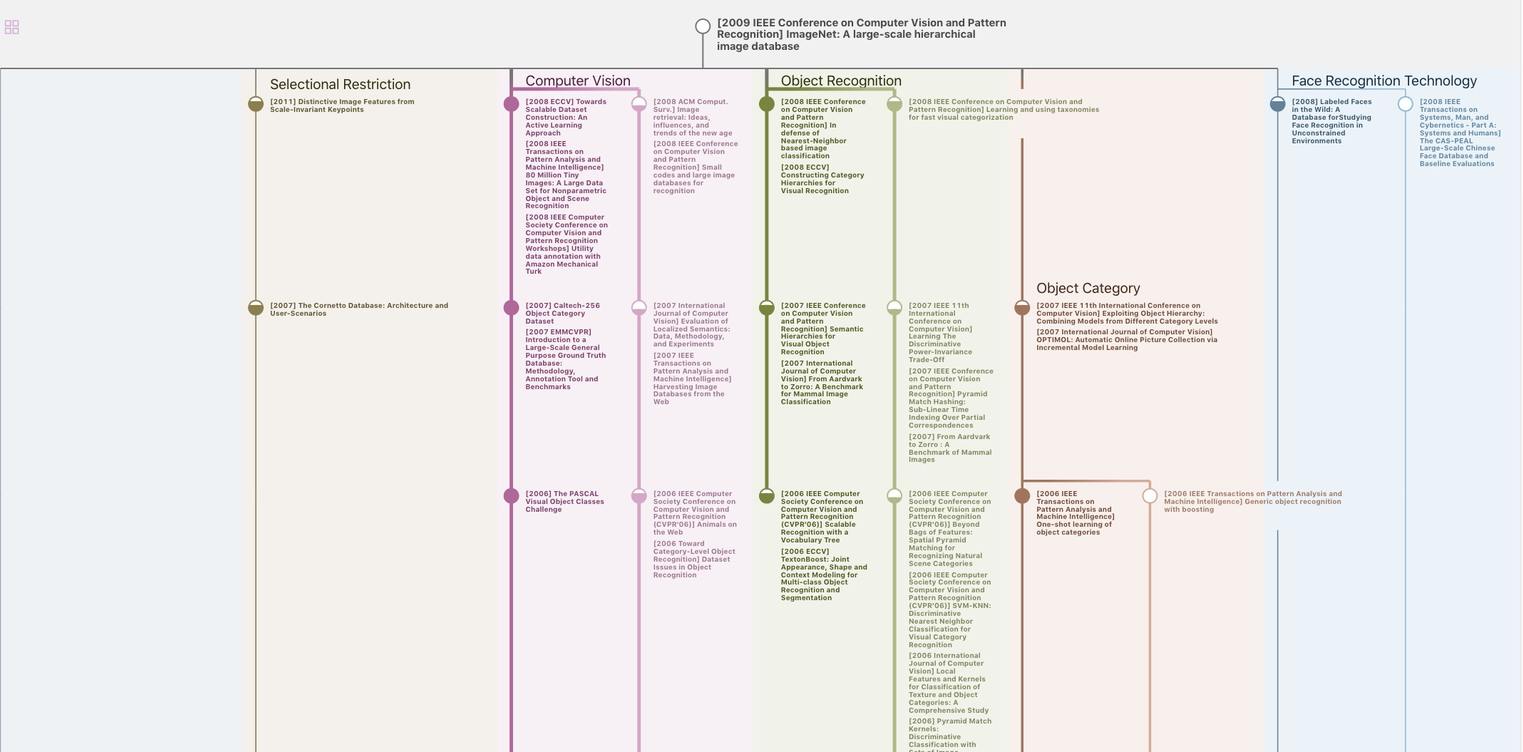
生成溯源树,研究论文发展脉络
Chat Paper
正在生成论文摘要