Medical machine learning based on multiobjective evolutionary algorithm using learning decomposition
Expert Systems with Applications(2023)
摘要
Medical machine learning technology has garnered great attention from both the computer and medical fields. In this study, a multi-objective evolutionary algorithm integrating decomposition and harris hawks learning (MOEA/D-HHL) is presented for medical machine learning; harris hawks learning can guarantee a good variety and systematic MOEA/D-HHL solutions. The performance of this MOEA/D-HHL is first evaluated using these benchmarks (DTLZ1-DTLZ7). In addition, MOEA/D-HHL is used to construct machine learning algorithms for medical cancer gene expression data sets with the following three objectives in mind: selected features, classification accuracy, and correlation measures. The MOEA/D-HHL is finally applied efficiently to the clinical data of lupus nephritis and pulmonary hypertension with the best NMI of 0.9652 and ARI of 0.9749 values on lupus nephritis, and the best NMI of 0.9686 and ARI of 0.9742 values on pulmonary hypertension respectively. On clinically relevant data for lupus nephritis and pulmonary hypertension, the experimental results indicate that the proposed MOEA/D-HHL algorithm outperforms current methods. The statistical results demonstrate that all metrics have predictive capabilities and that the suggested MOEA/D-HHL is more stable for an emerging medical machine learning framework. MOEA/D-HHL may be seen as a promising computer-assisted approach for medical machine learning development.
更多查看译文
关键词
Medical machine learning,Multi-objective,Harris hawks learning,Evolutionary decomposition algorithm,Cancer gene expression data sets,Lupus nephritis,Pulmonary hypertension
AI 理解论文
溯源树
样例
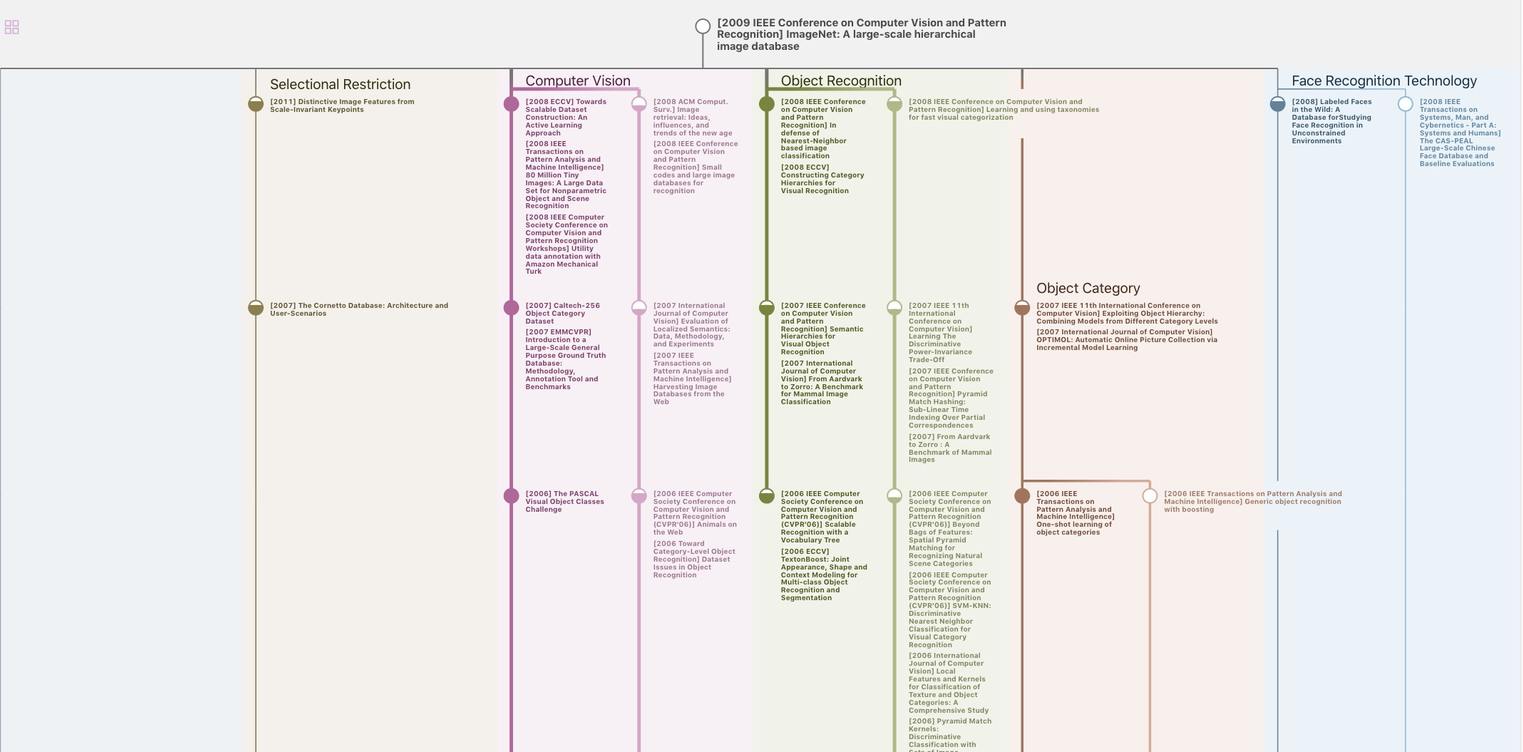
生成溯源树,研究论文发展脉络
Chat Paper
正在生成论文摘要