Personalized Dynamic Attention Multi-task Learning model for document retrieval and query generation
Expert Systems with Applications(2023)
摘要
In the actual retrieval scenario, queries from different users tend to have different retrieval intentions. Accurately understanding users’ retrieval intentions is a fundamental challenge for search engines. In this paper, we propose a Personalized Dynamic Attention Multi-task Learning model (PDAML) to solve this problem, which can clarify the user’s retrieval intention and generate a personalized document list. Specifically, we design a personalized retrieval model that can learn user retrieval preferences based on the user’s historical behavior. In addition, we propose an ad-hoc model based on multi-task learning to train query generation tasks and document retrieval tasks to enhance query representation and integrate document-aware interactive information into query representation through a dynamic attention mechanism. We can generate query representations with intent information and rank candidate documents through the interaction of the two sub-models. Experimental results on the publicly available AOL and SogouQ datasets demonstrate the effectiveness of PDAML, which improves the retrieval accuracy and personalized experience for users.
更多查看译文
关键词
Multi-task learning,Context-aware model,Personalized document retrieval,Deep learning
AI 理解论文
溯源树
样例
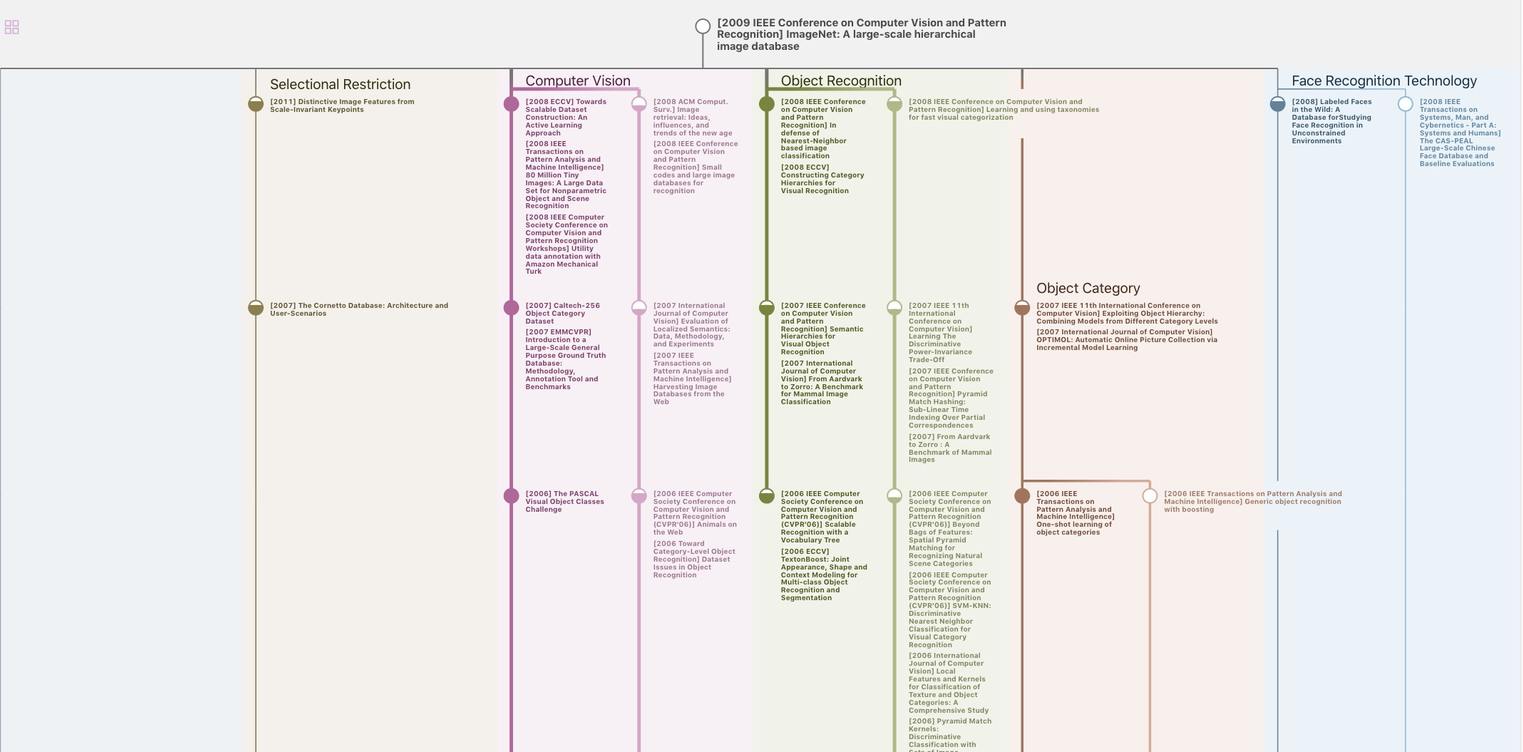
生成溯源树,研究论文发展脉络
Chat Paper
正在生成论文摘要