Missing data in amortized simulation-based neural posterior estimation
biorxiv(2023)
摘要
Amortized simulation-based neural posterior estimation provides a novel machine learning based approach for solving parameter estimation problems. It has been shown to be computationally efficient and able to handle complex models and data sets. Yet, the available approach cannot handle the in experimental studies ubiquitous case of missing data, and might provide incorrect posterior estimates. In this work, we discuss various ways of encoding missing data and integrate them into the training and inference process. We implement the approaches in the BayesFlow methodology, an amortized estimation framework based on invertible neural networks, and evaluate their performance on multiple test problems. We find that an approach in which the data vector is augmented with binary indicators of presence or absence of values performs the most robustly. Accordingly, we demonstrate that amortized simulation-based inference approaches are applicable even with missing data, and we provide a guideline for their handling, which is relevant for a broad spectrum of applications.
### Competing Interest Statement
The authors have declared no competing interest.
更多查看译文
关键词
neural posterior estimation,simulation-based
AI 理解论文
溯源树
样例
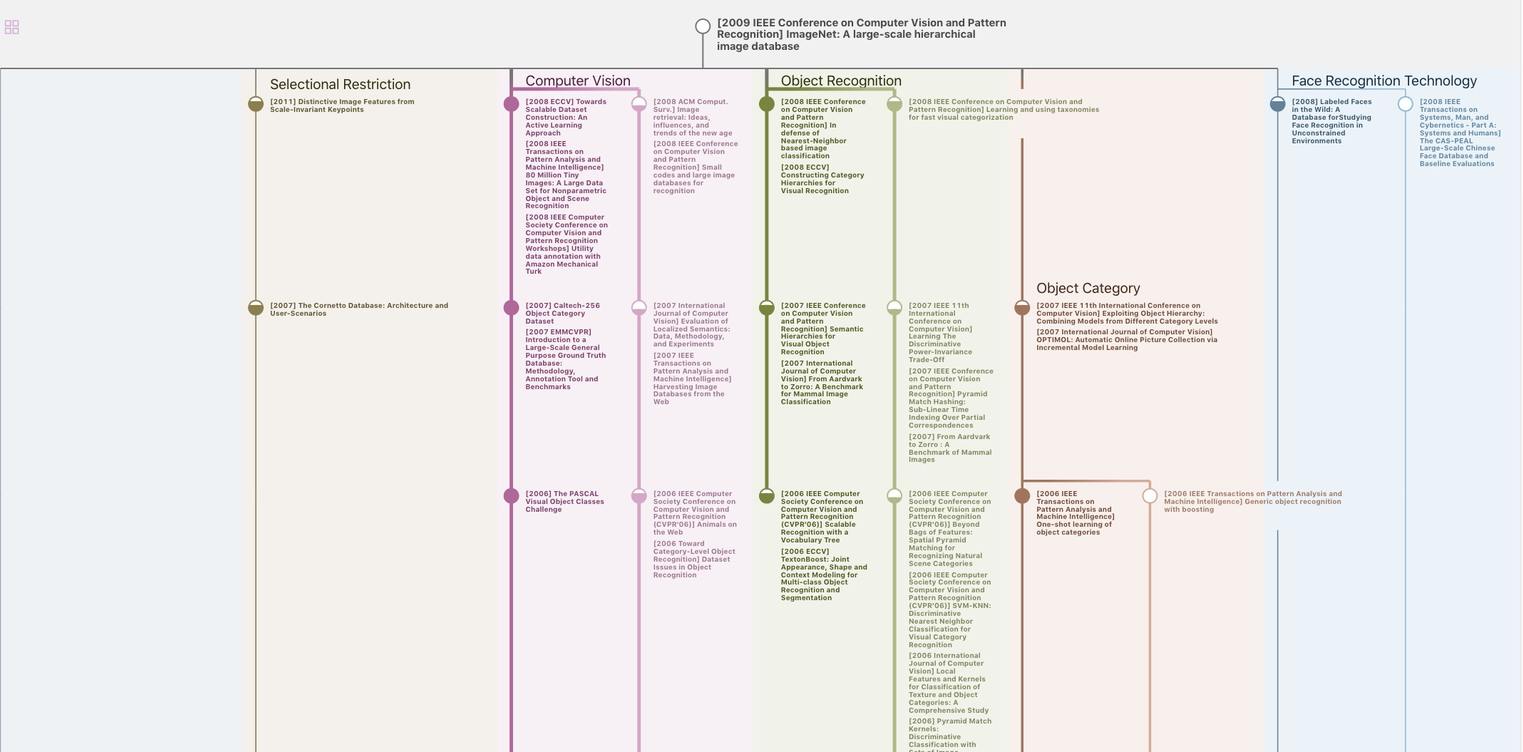
生成溯源树,研究论文发展脉络
Chat Paper
正在生成论文摘要