Spectral method for macro and micronutrient prediction in soybean leaves using interval partial least squares regression
European Journal of Agronomy(2023)
Abstract
Studies on the use of hyperspectral remote sensing for nutrient prediction in soybean (Glycine max L.) leaves are limited, especially regarding the selection of wavelengths. Therefore, the objective of this study was to estimate macro and micronutrients in soybean leaves by employing reflectance data modeled by Partial Least Squares Regression (PLSR) from bands selected by the iPLS (Interval PLS), method with a script developed for MATLAB R2018a. The experimental area was treated with different doses of limestone, with and without incorporation, also with phosphogypsum application in additional treatments. The soybean crops of 2018/2019 and 2019/2020 had the nutritional status evaluated by a random collection of the third fully developed trefoil in the R2 (full flowering) and R5.5 (76–100% grain filling) reproductive phenological stages. The leaf samples were submitted to conventional laboratory analyses. The Ca, Mg, K, P, S, Cu, Fe, Mn, and Zn elements in the leaves were quantified through spectrometry. A field spectroradiometer performed spectral data acquisition in the visible-near infrared, short-wave infrared spectral range of soybean leaves. The spectral curves were subjected to iPLS, and PLSR models were fitted. After iPLS selection, the coefficient of determination (R2) values of PLSR models were on average 15.3% and 16.6% higher in calibration and validation, respectively, compared to classical methods. The PLSR model for Mn, which obtained R2 above 0.90 in the calibration and validation phases, stood out. The Root Mean Square Error (RMSE) decreased, on average, more than 15.0%, both in calibration and validation, after applying the iPLS selection. The models for Ca and Mn stood out with over a 30.0% decrease in RMSE, in calibration and validation. The iPLS selection was efficient in improving the performance and accuracy of the PLSR models, increasing the R2 and decreasing the RMSE, opening new possibilities for the analysis and prediction of macro and micronutrients in plants.
MoreTranslated text
Key words
Chemometrics,IPLS,Selection of variables,Hyperspectral,Proximal remote sensing
AI Read Science
Must-Reading Tree
Example
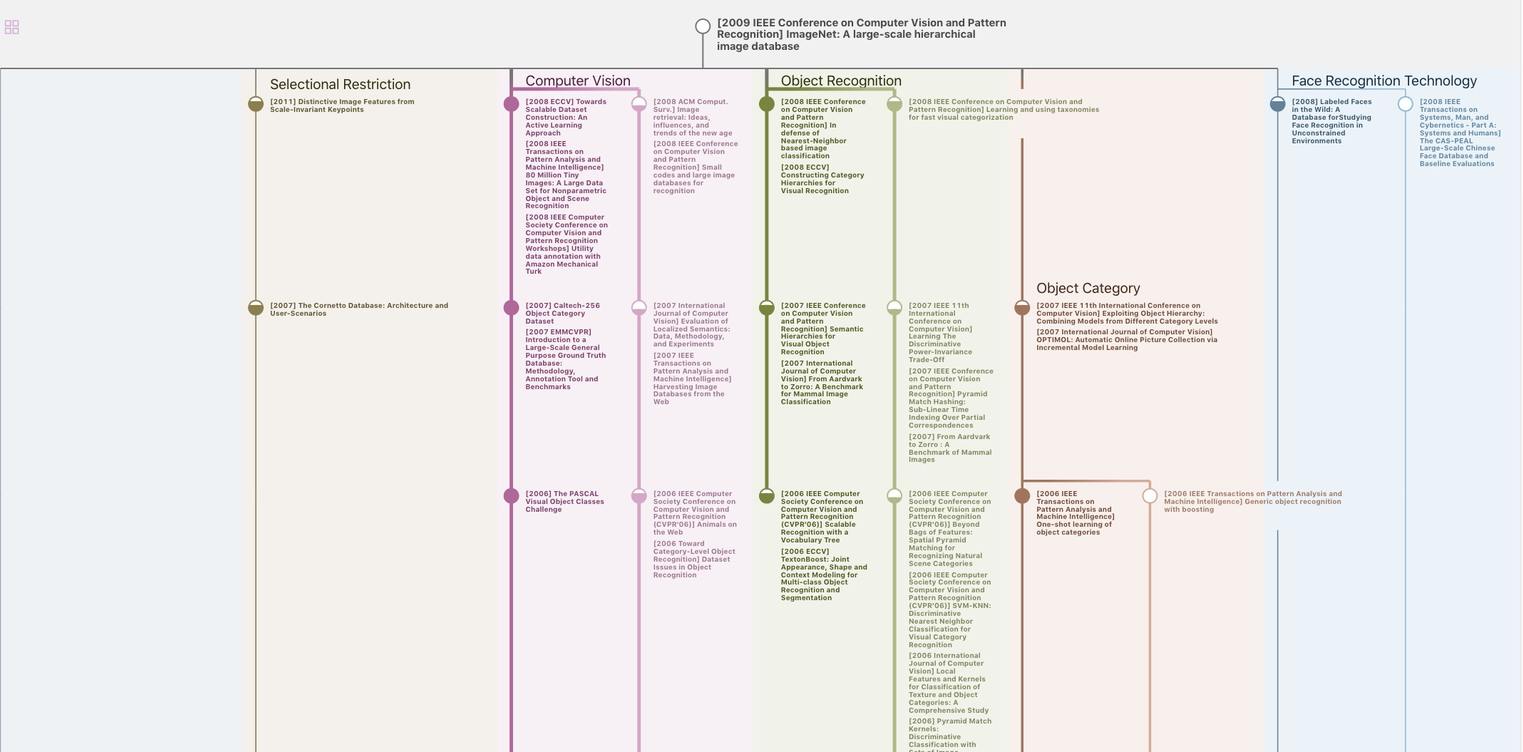
Generate MRT to find the research sequence of this paper
Chat Paper
Summary is being generated by the instructions you defined