Explainable artificial intelligence for photovoltaic fault detection: A comparison of instruments
Solar Energy(2023)
摘要
Faults in photovoltaic arrays are known to cause severe energy losses. Data-driven models based on machine learning have been developed to automatically detect and diagnose such faults. A majority of the models proposed in the literature are based on artificial neural networks, which unfortunately represent black-boxes, hindering user interpretation of the models’ results. Since the energy sector is a critical infrastructure, the security of energy supply could be threatened by the deployment of such models. This study implements explainable artificial intelligence (XAI) techniques to extract explanations from a multi-layer perceptron (MLP) model for photovoltaic fault detection, with the aim of shedding some light on the behavior of XAI techniques in this context. Three techniques were implemented: Shapley Additive Explanations (SHAP), Anchors and Diverse Counterfactual Explanations (DiCE), each representing a distinct class of local explainability techniques used to explain predictions. For a model with 99.11% accuracy, results show that SHAP explanations are largely in line with domain knowledge, demonstrating their usefulness to generate valuable insights on model behavior which could potentially increase user trust in the model. Compared to Anchors and DiCE, SHAP demonstrated a higher degree of stability and consistency.
更多查看译文
关键词
Photovoltaic fault detection,Machine learning,Artificial intelligence,XAI
AI 理解论文
溯源树
样例
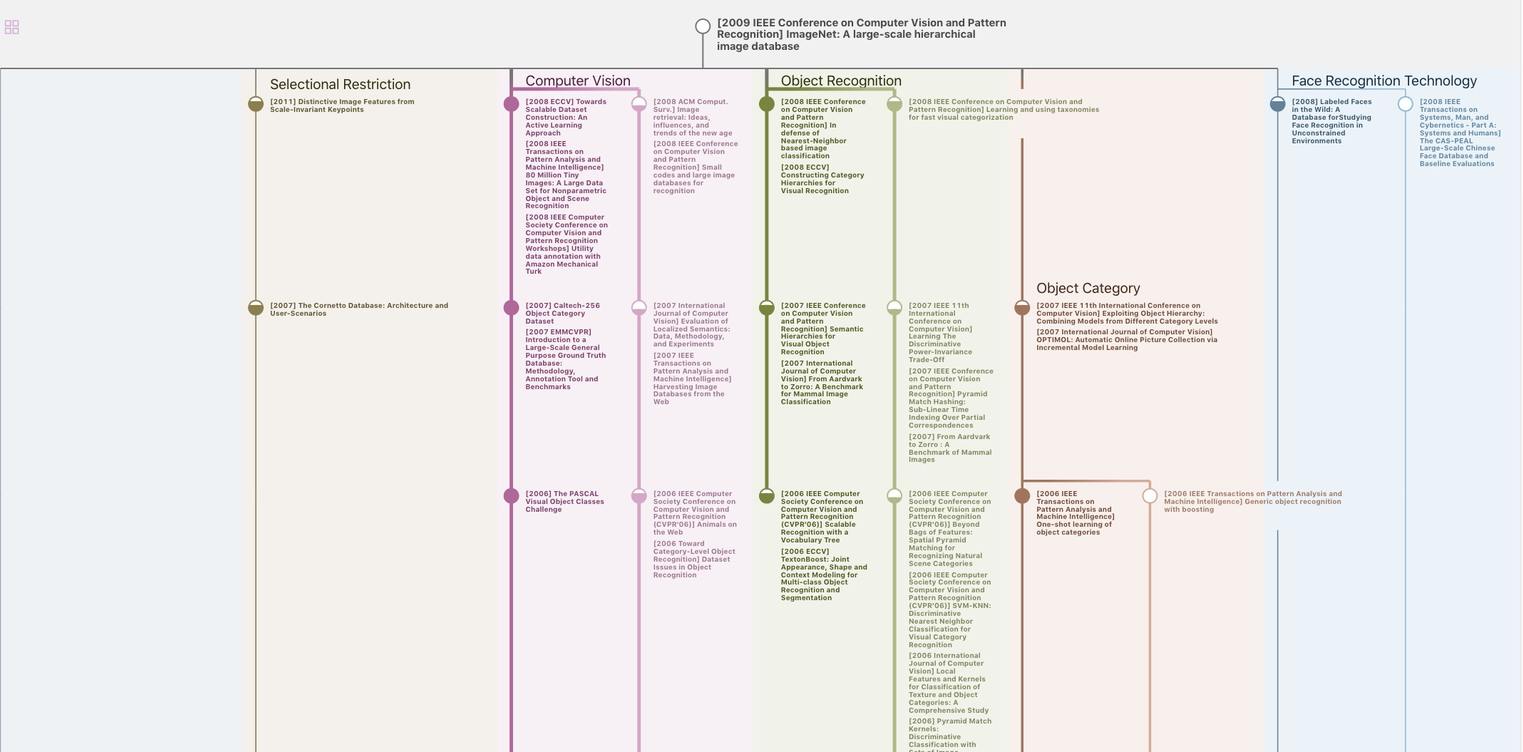
生成溯源树,研究论文发展脉络
Chat Paper
正在生成论文摘要