Learning a spatial-temporal symmetry network for video super-resolution
Applied Intelligence(2022)
摘要
The video super-resolution (VSR) method is designed to estimate and restore high-resolution (HR) sequences from low-resolution (LR) input. For the past few years, many VSR methods with machine learning have been proposed that combine both the convolutional neural network (CNN) and motion compensation. Most mainstream approaches are based on optical flow or deformation convolution, and both need accurate estimates for motion compensation. However, most previous methods have not been able to fully utilize the spatial-temporal symmetrical information from input sequences. Moreover, much computation is consumed by aligning every neighbouring frame to the reference frame separately. Furthermore, many methods reconstruct HR results on only a single scale, which limits the reconstruction accuracy of the network and its performance in complex scenes. In this study, we propose a spatial-temporal symmetry network (STSN) to solve the above deficiencies. STSN includes four parts: prefusion, alignment, postfusion and reconstruction. First, a two-stage fusion strategy is applied to reduce the computation consumption of the network. Furthermore, ConvGRU is utilized in the prefusion module, the redundant features between neighbouring frames are eliminated, and several neighbouring frames are fused and condensed into two parts. To generate accurate offset maps, we present a spatial-temporal symmetry attention block (STSAB). This component exploits the symmetry of spatial-temporal combined spatial attention. In the reconstruction module, we propose an SR multiscale residual block (SR-MSRB) to enhance reconstruction performance. Abundant experimental results that test several datasets show that our method possesses better effects and efficiency in both quantitative and qualitative measurement indices compared with state-of-the-art methods.
更多查看译文
关键词
Video super-resolution, Motion estimation, Spatial-temporal symmetry, Convolutional neural network
AI 理解论文
溯源树
样例
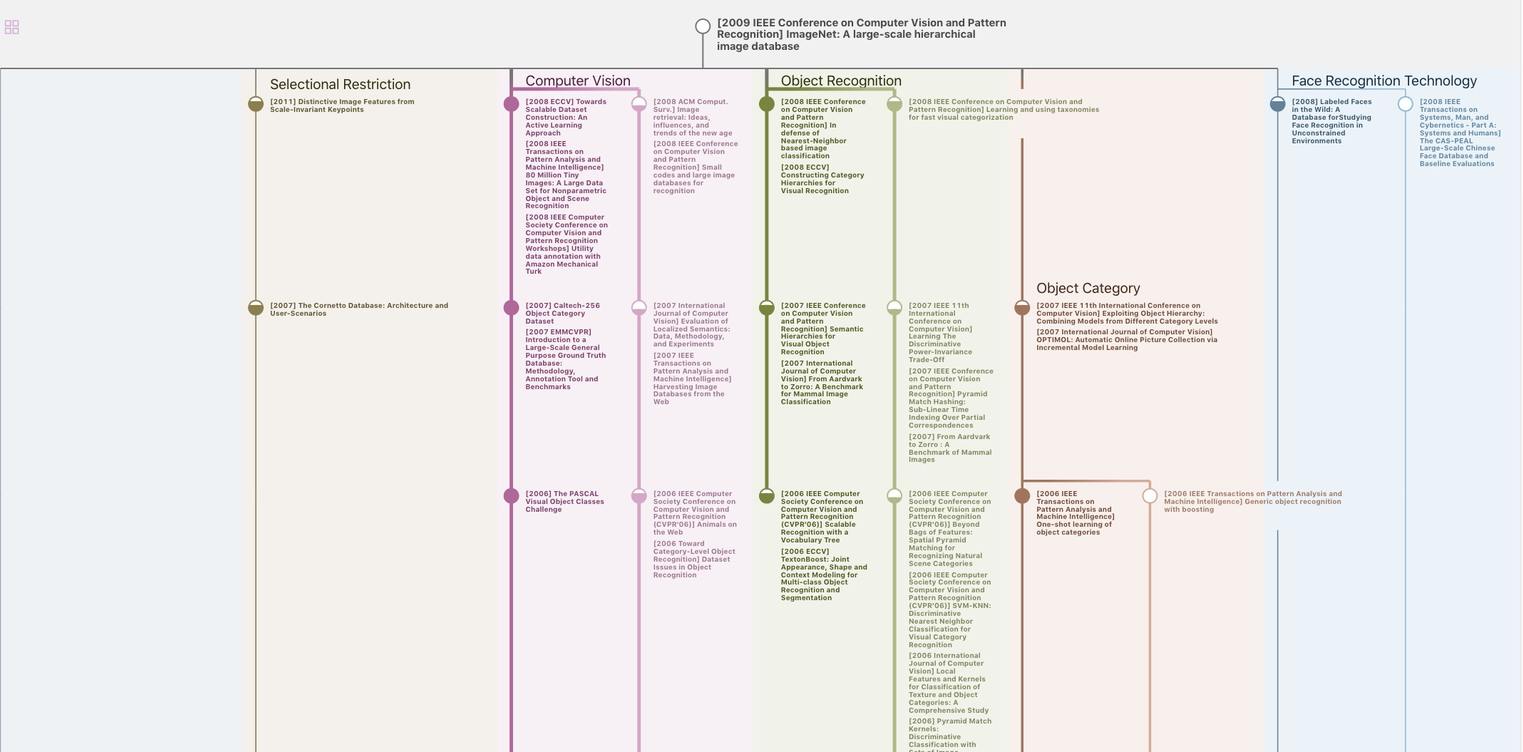
生成溯源树,研究论文发展脉络
Chat Paper
正在生成论文摘要