An Efficient Robot Precision Assembly Skill Learning Framework Based on Several Demonstrations
IEEE TRANSACTIONS ON AUTOMATION SCIENCE AND ENGINEERING(2023)
摘要
This paper proposes an efficient robot assembly skill learning framework based on only a few demonstrations. The assembly skill learning process consists of two phases, e.g., the pre-training phase and the self-learning phase. In pre-training phase, the assembly networks are initialized from demonstration data. A novel data augmentation model based on state transition model is designed, which only requires several human demonstrations to learn the parameters of the model and reduces the cost of demonstration data collection in pre-training. In self-learning phase, the pre-trained assembly networks are further optimized by a hybrid exploration strategy in assembly environment, which explores both parameter space and action space to increase exploration efficiency. On par with the learning framework, a fuzzy reward function balancing the efficiency and compliance of assembly is elaborately designed to evaluate action's performance in assembly skill self-learning process. Series of physical experiments were well conducted on a sophisticated assembly platform to verify the effectiveness of the proposed efficient robot assembly skill learning framework. Experimental results demonstrate that the learning efficiency of the proposed framework is at least three times as efficient as the state-of-art methods, while the skill performance outperforms the state-of-art by more than 50%.
更多查看译文
关键词
Robots,Reinforcement learning,Data models,Task analysis,Learning systems,Trajectory,Training,Efficient robot skill learning,demonstration learning,robot assembly,robot and automation
AI 理解论文
溯源树
样例
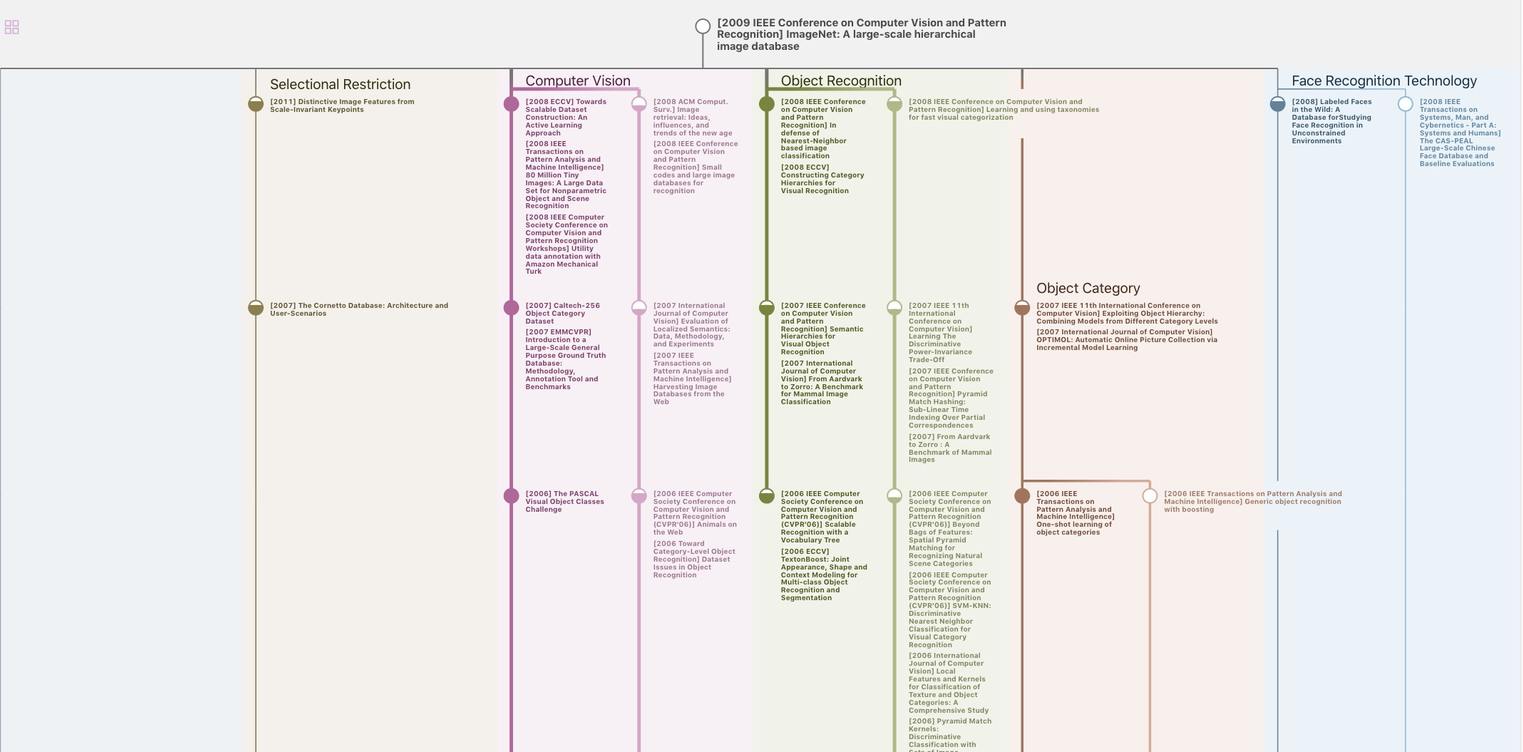
生成溯源树,研究论文发展脉络
Chat Paper
正在生成论文摘要