On enhancing prediction abilities of vision-based metallic surface defect classification through adversarial training
Engineering Applications of Artificial Intelligence(2023)
Abstract
Computer vision is augmented in various manufacturing industries to perform automated inspection operations accurately and efficiently. It has been observed that the performance of vision-based inspection approaches degrades considerably upon utilizing images captured under shop-floor conditions. This work proposes utilizing Histogram Equalization and adversarial training through Neural Structure Learning (NSL) for developing a robust vision-based Surface Defect Classification framework. A novel deep neural network architecture obtains adversarial samples in the extracted feature space instead of obtaining the same in the original input image space. The architecture can be easily integrated and employed with various machine learning models. A commonly employed steel surface defect dataset (NEU) with practical relevance to industrial cases is selected for the model training and experimental studies. The robustness of the proposed approach is evaluated over the Extended Diversity Enhanced (ENEU) dataset derived by simulating image acquisition variations similar to shop floor conditions. The results reveal that the proposed approach enhances the recognition accuracy of the baseline method from 87.7% to 92.4% over ENEU. The prediction accuracy of the proposed approach is considerably better than the traditional methods and deep learning competitors over ENEU. The qualitative and quantitative comparison of results obtained using the present approach with methods reported in the literature demonstrates the effectiveness of adversarial training in improving the generalization abilities of machine learning models.
MoreTranslated text
Key words
Computer vision,Defect detection,Steel surface,Adversarial training,Histogram Equalization
AI Read Science
Must-Reading Tree
Example
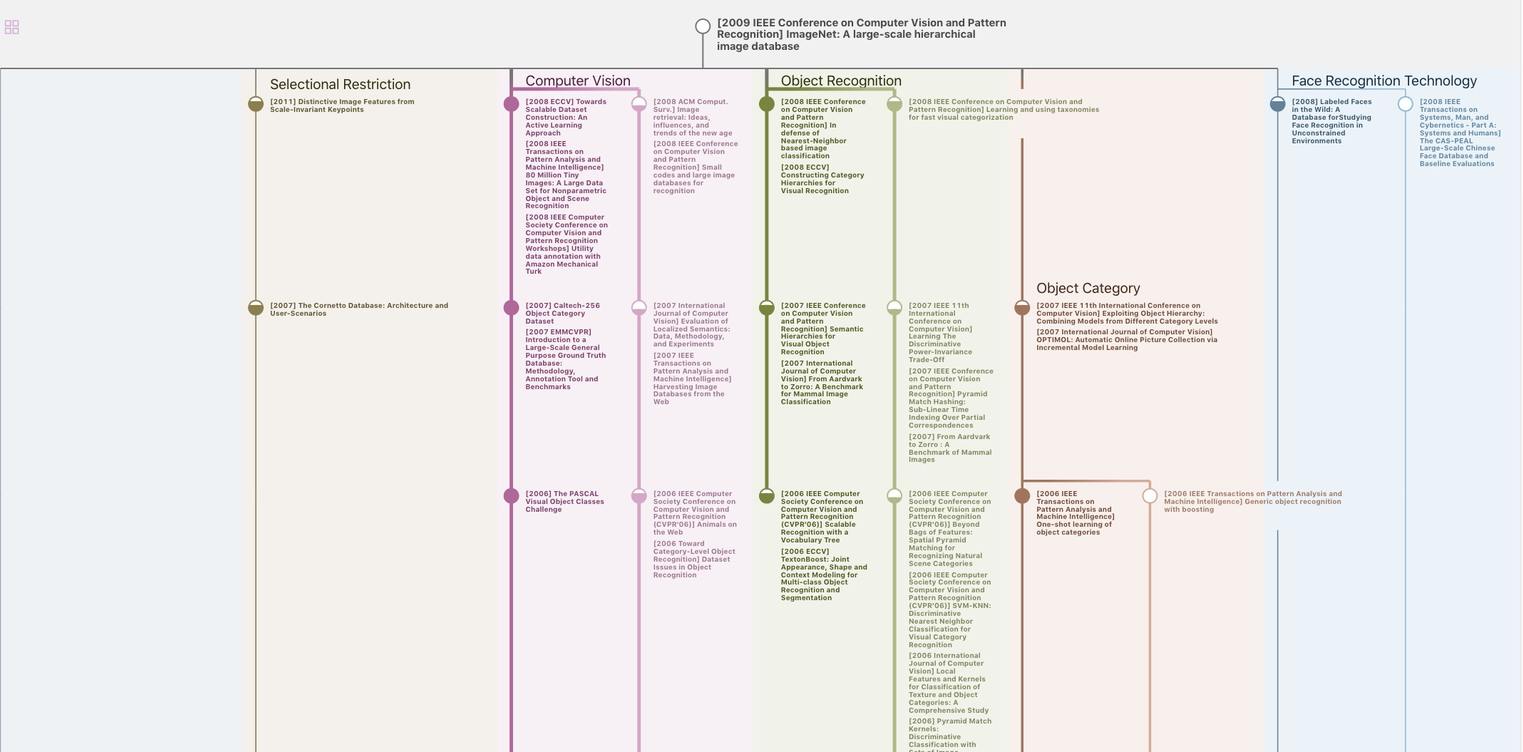
Generate MRT to find the research sequence of this paper
Chat Paper
Summary is being generated by the instructions you defined