An End-to-End Localizer for Long-Term Topological Localization in Large-Scale Changing Environments
IEEE Transactions on Industrial Electronics(2023)
摘要
Long-term localization is an essential task for unmanned ground vehicles; however, it suffers from scene changes due to various environmental conditions (e.g., lighting conditions, seasonal shifts, viewpoint changes, or occlusions). In this article, we propose a novel end-to-end localizer for robust long-term localization in changing environments. First, a lightweight two-head place classification network is proposed in which a place is regarded as a category. Considering seasonal changes lead to severe variants of local structures, large-sized filters in the first layer and a large-scale average-pooling strategy are designed to focus regional context features of scenes. Motivated by the reward and punishment mechanisms in reinforcement learning, an incremental learning strategy is proposed to distinguish distinctive scenes for robust topological localization. Extensive experiments tested on three datasets whose collecting periods are over a year are utilized to validate the feasibility and performance of our method.
更多查看译文
关键词
End-to-end localizer,incremental learning,long-term topological localization,unmanned ground vehicles (UGVs)
AI 理解论文
溯源树
样例
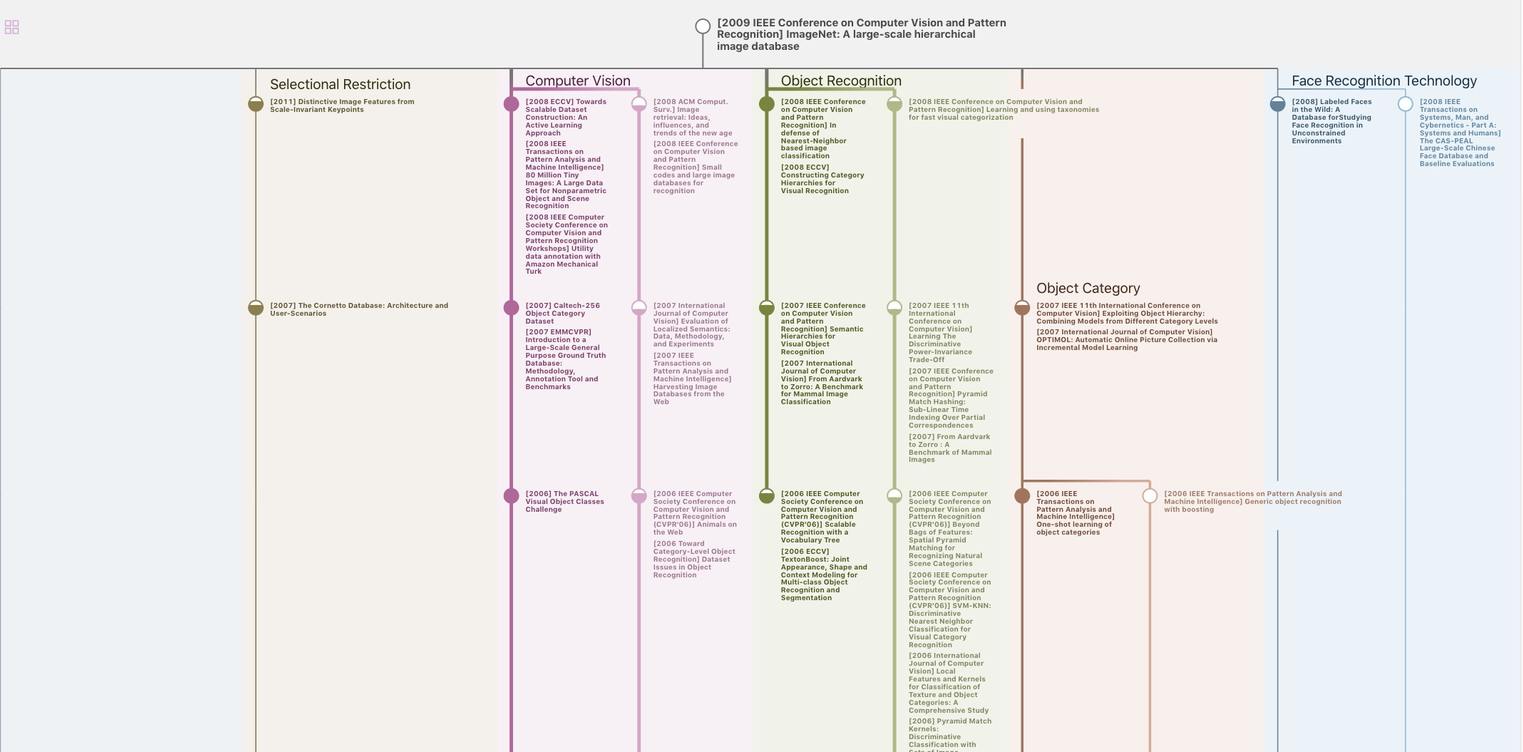
生成溯源树,研究论文发展脉络
Chat Paper
正在生成论文摘要