CELR: Cloud Enhanced Local Reconstruction from low-dose sparse Scanning Electron Microscopy images
2022 25th Euromicro Conference on Digital System Design (DSD)(2022)
Abstract
Current Scanning Electron Microscopy (SEM) acquisition techniques are far too slow to capture large volumes in a feasible time. One solution is to use low-dose and sparse imaging. By computationally denoising and inpainting an image with acceptable quality can be approximated. This approach, however, requires significant compute resources. Therefore, this paper proposes CELR, a framework, that hides the computationally expensive workload of reconstructing low-dose sparse SEM images, such that (delayed) live reconstruction is possible. Live reconstruction is possible by using Convolutional Neural Networks (CNNs) that approximate a classical reconstruction algorithm like GOAL. The reconstruction by CNNs is done locally, while recurring training of CNNs is done in the cloud. Moreover, training labels are generated by GOAL in the cloud. Next to the framework, this paper evaluates and optimizes the CNN reconstruction throughput by employing Nvidia's TensorRT. This paper also touches upon open research questions about on-the-fly CNN training. The combination of CELR and TensorRT enables large volume acquisitions with a dwell-time of
$\mathbf{1}\mu s$
and 10% pixel coverage to be reconstructed on a single GPU.
MoreTranslated text
Key words
cloud enhanced local reconstruction,electron,low-dose
AI Read Science
Must-Reading Tree
Example
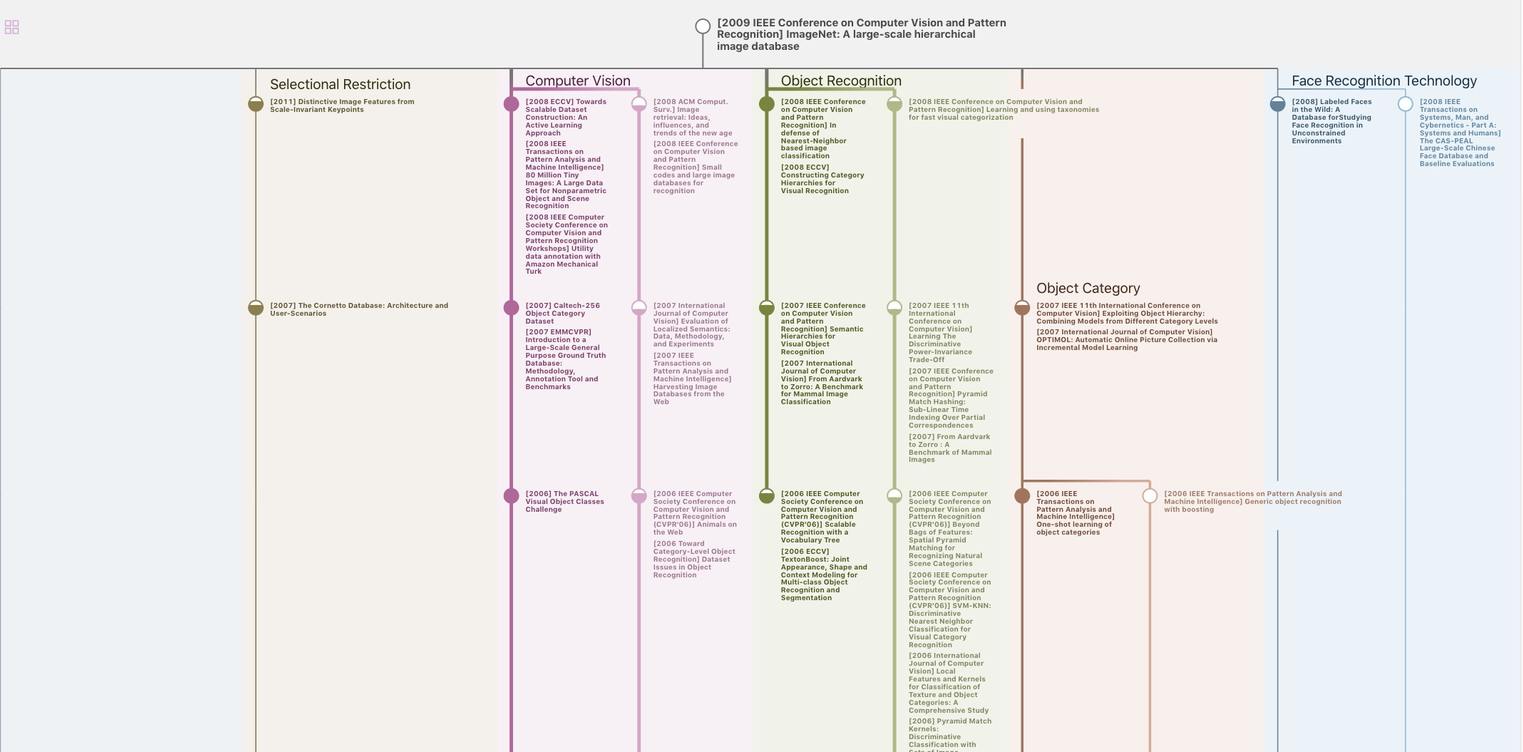
Generate MRT to find the research sequence of this paper
Chat Paper
Summary is being generated by the instructions you defined