Deep convolutional neural network based closed-loop SOC estimation for lithium-ion batteries in hierarchical scenarios
Energy(2023)
Abstract
Battery type variation and sensor measurement noise in different scenarios decrease the accuracy and robustness of the state of charge (SOC) estimation. To develop a universal SOC estimator for different scenarios, this study proposes a closed-loop framework based on a deep convolutional neural network (DCNN). First, a two-dimensional CNN is proposed to extract the features of the input data based on the convolutional operation and average pooling to train a pre-trained model with a smaller model size, and the raw data are processed by a moving window. Then, transfer learning and pruning operations are employed to help the pre-trained model quickly adapt to hierarchical scenarios. Finally, to improve the robustness of SOC estimation under low-quality measurement, the DCNN is proposed to learn the relationship between the SOC and measurement equations of the Kalman filter to realise closed-loop estimation. Several experiments were carried out for validation, including battery tests of different types and aging states. The evaluation results show that root mean square errors (RMSEs) of less than 2.47% can be obtained by fine-tuning the parameters of the last few layers. We demonstrated the robustness of the proposed method in three hierarchical scenarios; it maintained RMSEs of less than 1.78% under severe disturbances.
MoreTranslated text
Key words
State of charge,Hierarchical scenarios,Deep convolutional neural network,Transfer learning,Pruning operation,Closed-loop estimation
AI Read Science
Must-Reading Tree
Example
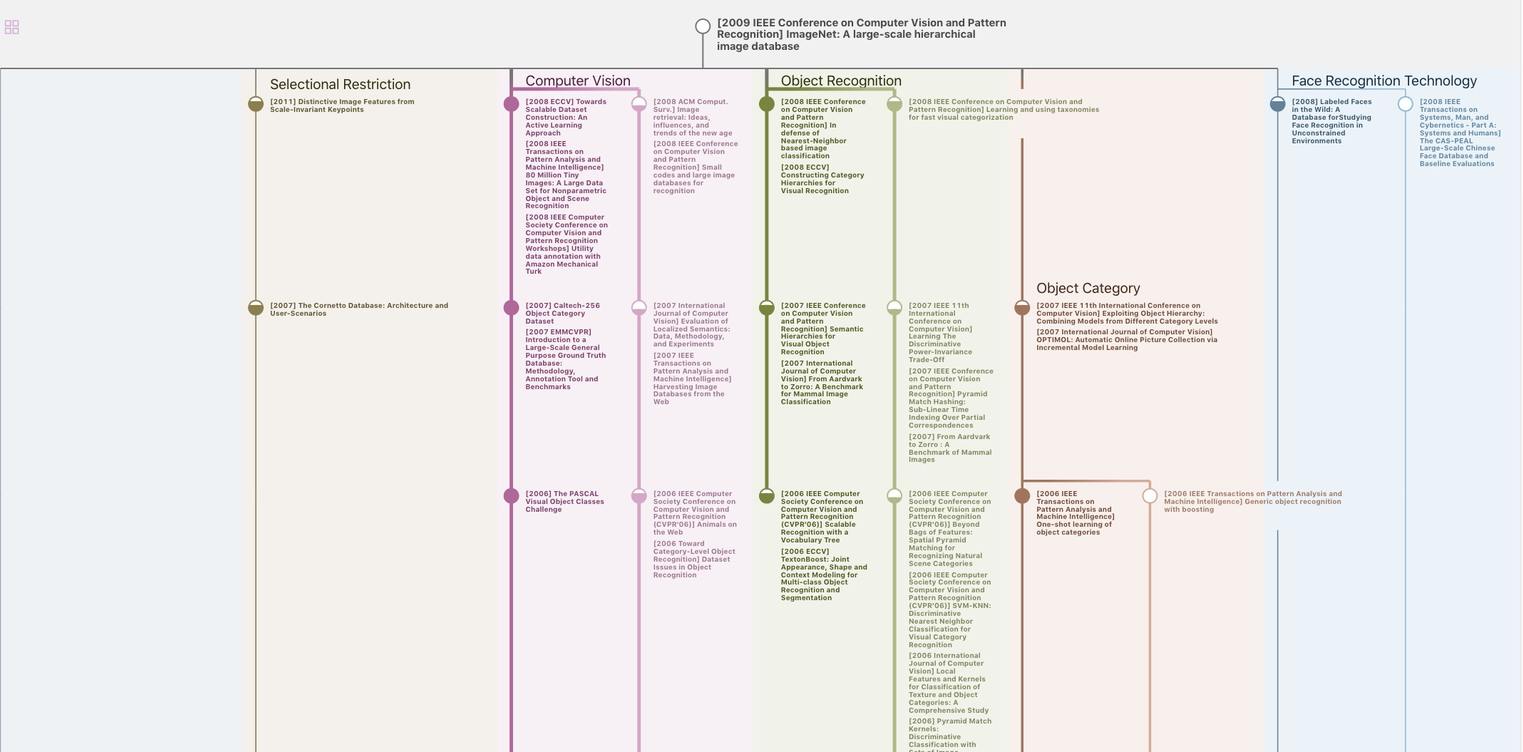
Generate MRT to find the research sequence of this paper
Chat Paper
Summary is being generated by the instructions you defined